Statistical Learning under Heterogenous Distribution Shift
CoRR(2023)
Abstract
This paper studies the prediction of a target $\mathbf{z}$ from a pair of random variables $(\mathbf{x},\mathbf{y})$, where the ground-truth predictor is additive $\mathbb{E}[\mathbf{z} \mid \mathbf{x},\mathbf{y}] = f_\star(\mathbf{x}) +g_{\star}(\mathbf{y})$. We study the performance of empirical risk minimization (ERM) over functions $f+g$, $f \in \mathcal{F}$ and $g \in \mathcal{G}$, fit on a given training distribution, but evaluated on a test distribution which exhibits covariate shift. We show that, when the class $\mathcal{F}$ is "simpler" than $\mathcal{G}$ (measured, e.g., in terms of its metric entropy), our predictor is more resilient to \emph{heterogenous covariate shifts} in which the shift in $\mathbf{x}$ is much greater than that in $\mathbf{y}$. These results rely on a novel H\"older style inequality for the Dudley integral which may be of independent interest. Moreover, we corroborate our theoretical findings with experiments demonstrating improved resilience to shifts in "simpler" features across numerous domains.
MoreTranslated text
Key words
heterogenous distribution shift,learning
AI Read Science
Must-Reading Tree
Example
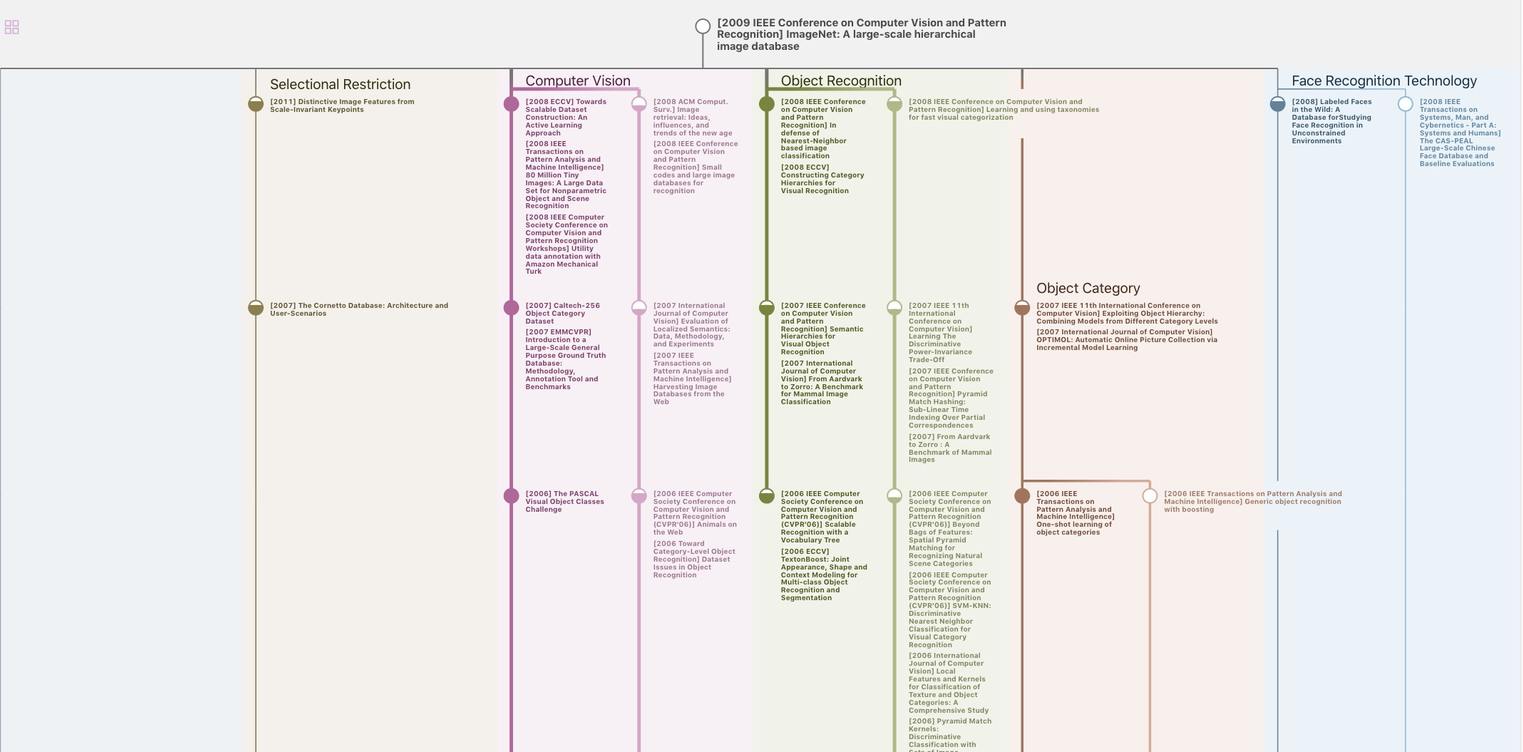
Generate MRT to find the research sequence of this paper
Chat Paper
Summary is being generated by the instructions you defined