A multigrid method for PDE-constrained optimization with uncertain inputs
arxiv(2023)
摘要
We present a multigrid algorithm to solve efficiently the large saddle-point systems of equations that typically arise in PDE-constrained optimization under uncertainty. The algorithm is based on a collective smoother that at each iteration sweeps over the nodes of the computational mesh, and solves a reduced saddle-point system whose size depends on the number $N$ of samples used to discretized the probability space. We show that this reduced system can be solved with optimal $O(N)$ complexity. We test the multigrid method on three problems: a linear-quadratic problem for which the multigrid method is used to solve directly the linear optimality system; a nonsmooth problem with box constraints and $L^1$-norm penalization on the control, in which the multigrid scheme is used within a semismooth Newton iteration; a risk-adverse problem with the smoothed CVaR risk measure where the multigrid method is called within a preconditioned Newton iteration. In all cases, the multigrid algorithm exhibits very good performances and robustness with respect to all parameters of interest.
更多查看译文
关键词
multigrid method,optimization,pde-constrained
AI 理解论文
溯源树
样例
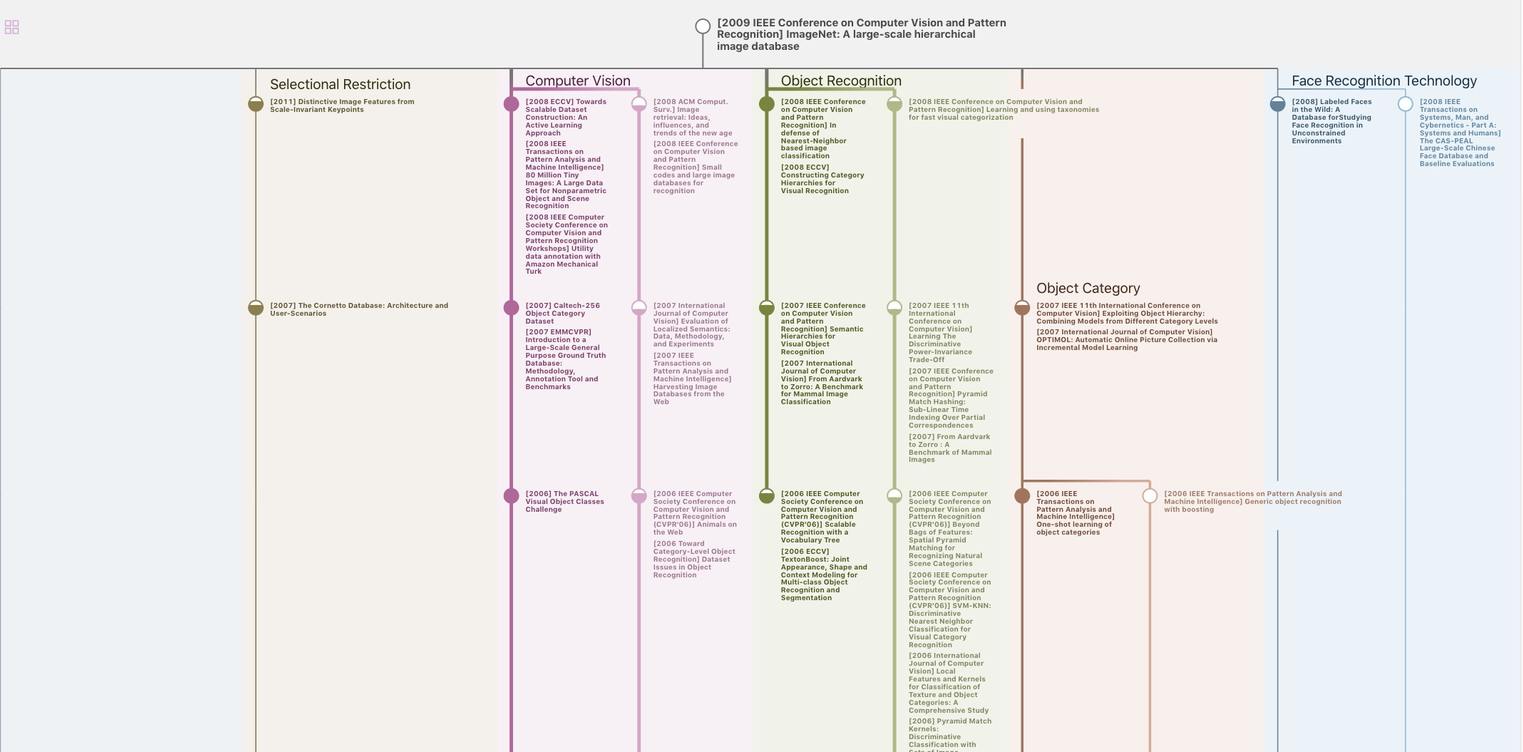
生成溯源树,研究论文发展脉络
Chat Paper
正在生成论文摘要