Active Reward Learning from Online Preferences
arxiv(2023)
摘要
Robot policies need to adapt to human preferences and/or new environments. Human experts may have the domain knowledge required to help robots achieve this adaptation. However, existing works often require costly offline re-training on human feedback, and those feedback usually need to be frequent and too complex for the humans to reliably provide. To avoid placing undue burden on human experts and allow quick adaptation in critical real-world situations, we propose designing and sparingly presenting easy-to-answer pairwise action preference queries in an online fashion. Our approach designs queries and determines when to present them to maximize the expected value derived from the queries' information. We demonstrate our approach with experiments in simulation, human user studies, and real robot experiments. In these settings, our approach outperforms baseline techniques while presenting fewer queries to human experts. Experiment videos, code and appendices are found on our website: http://tinyurl.com/online-active
更多查看译文
关键词
active reward learning,online preferences,pairwise action preference queries,robot experiments
AI 理解论文
溯源树
样例
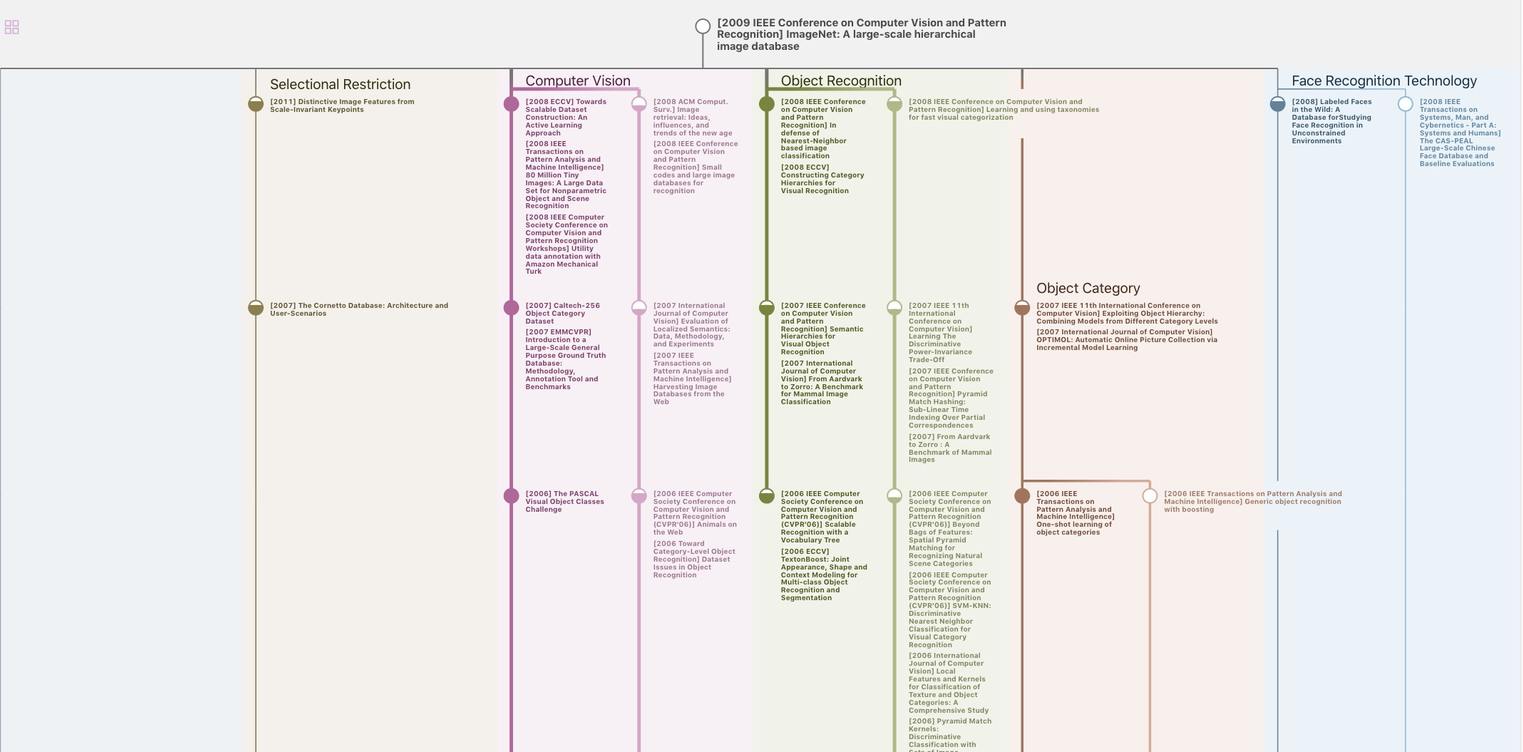
生成溯源树,研究论文发展脉络
Chat Paper
正在生成论文摘要