Contrast-PLC: Contrastive Learning for Packet Loss Concealment
arxiv(2023)
摘要
Packet loss concealment (PLC) is challenging in concealing missing contents both plausibly and naturally when there are only limited available context to use. Recently deep-learning based PLC algorithms have demonstrated their superiority over traditional counterparts; but their concealment ability is still mostly limited to a maximum of 120ms loss. Even with strong GAN-based generative models, it is still very challenging to predict long burst losses that could happen within/in-between phonemes. In this paper, we propose to use contrastive learning to learn a loss-robust semantic representation for PLC. A hybrid neural PLC architecture combining the semantic prediction and GAN-based generative model is designed to verify its effectiveness. Results on the blind test set of Interspeech2022 PLC Challenge show its superiority over commonly used UNet-style framework and the one without contrastive learning, especially for the longer burst loss at (120, 220] ms.
更多查看译文
关键词
contrastive learning,contrast-plc
AI 理解论文
溯源树
样例
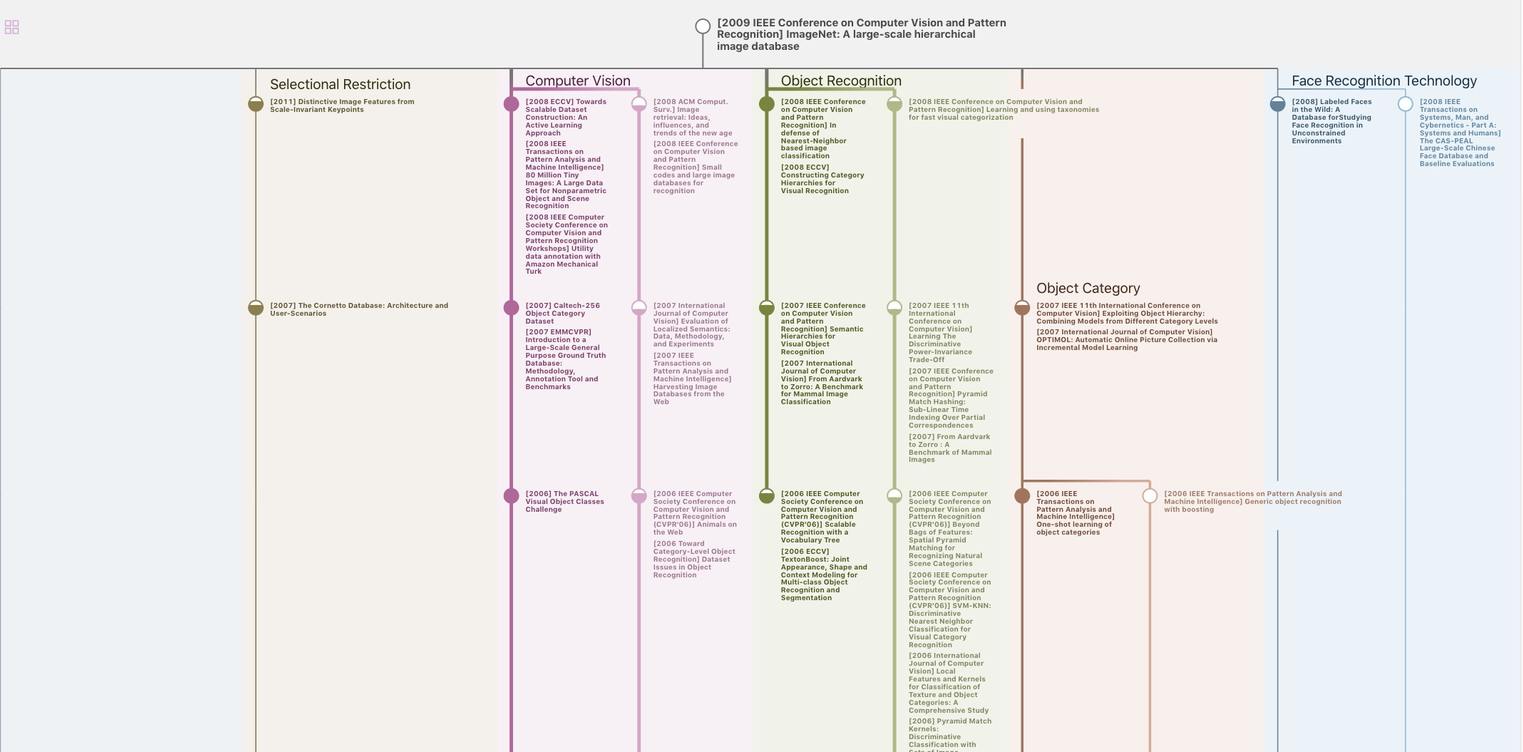
生成溯源树,研究论文发展脉络
Chat Paper
正在生成论文摘要