DeepCPG Policies for Robot Locomotion
IEEE TRANSACTIONS ON COGNITIVE AND DEVELOPMENTAL SYSTEMS(2023)
摘要
Central pattern generators (CPGs) form the neural basis of the observed rhythmic behaviors for locomotion in legged animals. The CPG dynamics organized into networks allow the emergence of complex locomotor behaviors. In this work, we take this inspiration for developing walking behaviors in multilegged robots. We present novel DeepCPG policies that embed CPGs as a layer in a larger neural network and facilitate end-to-end learning of locomotion behaviors in deep reinforcement learning (DRL) setup. We demonstrate the effectiveness of this approach on physics engine-based insectoid robots. We show that, compared to traditional approaches, DeepCPG policies allow sample-efficient end-to-end learning of effective locomotion strategies even in the case of high-dimensional sensor spaces (vision). We scale the DeepCPG policies using a modular robot configuration and multiagent DRL. Our results suggest that gradual complexification with embedded priors of these policies in a modular fashion could achieve nontrivial sensor and motor integration on a robot platform. These results also indicate the efficacy of bootstrapping more complex intelligent systems from simpler ones based on biological principles. Finally, we present the experimental results for a proof-of-concept insectoid robot system for which DeepCPG learned policies initially using the simulation engine and these were afterward transferred to real-world robots without any additional fine-tuning.
更多查看译文
关键词
Central pattern generator (CPG),deep neu26 ral networks,developmental robotics,,locomotion,reinforcement 27 learning (RL)
AI 理解论文
溯源树
样例
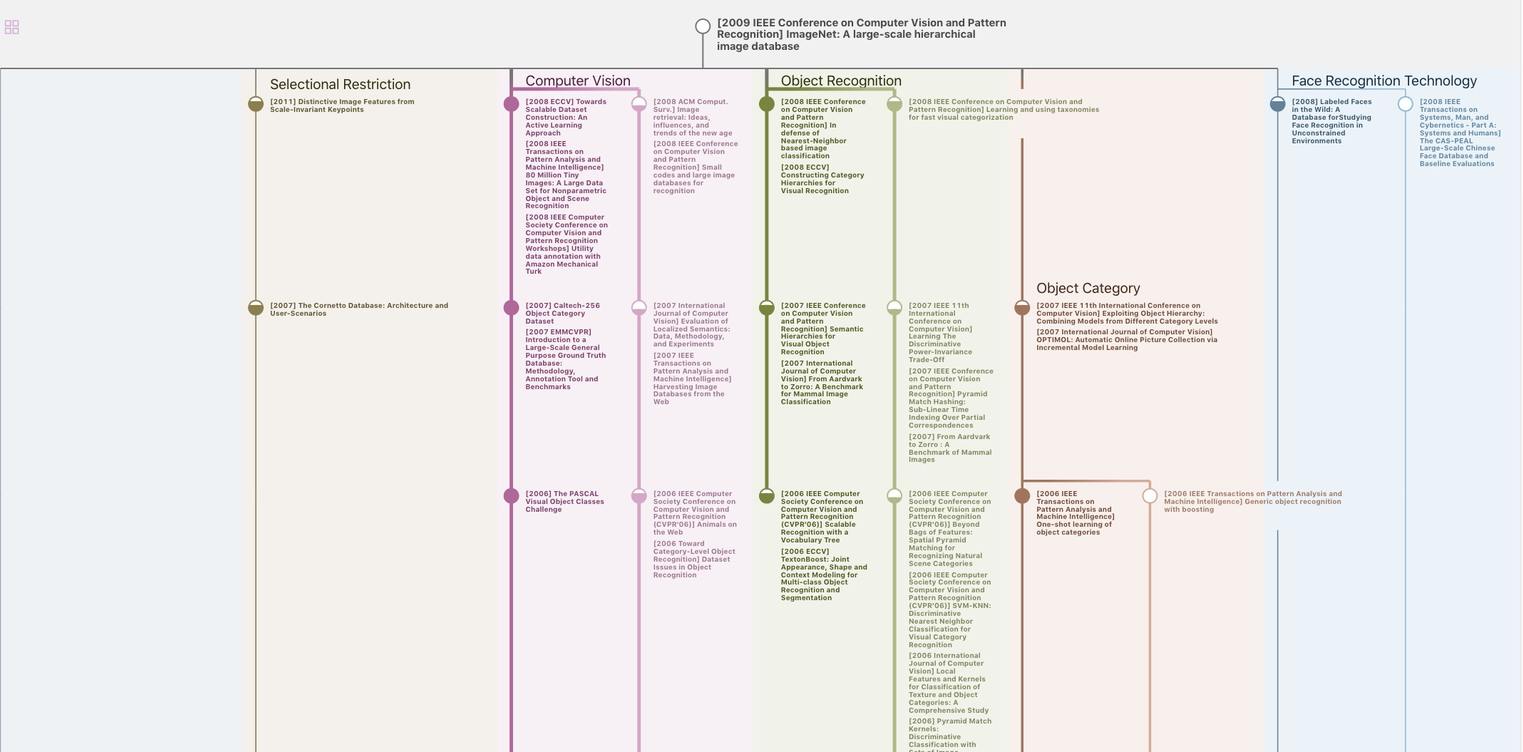
生成溯源树,研究论文发展脉络
Chat Paper
正在生成论文摘要