Ensemble knowledge distillation of self-supervised speech models
CoRR(2023)
Abstract
Distilled self-supervised models have shown competitive performance and efficiency in recent years. However, there is a lack of experience in jointly distilling multiple self-supervised speech models. In our work, we performed Ensemble Knowledge Distillation (EKD) on various self-supervised speech models such as HuBERT, RobustHuBERT, and WavLM. We tried two different aggregation techniques, layerwise-average and layerwise-concatenation, to the representations of different teacher models and found that the former was more effective. On top of that, we proposed a multiple prediction head method for student models to predict different layer outputs of multiple teacher models simultaneously. The experimental results show that our method improves the performance of the distilled models on four downstream speech processing tasks, Phoneme Recognition, Speaker Identification, Emotion Recognition, and Automatic Speech Recognition in the hidden-set track of the SUPERB benchmark.
MoreTranslated text
Key words
ensemble knowledge distillation,models,speech,self-supervised
AI Read Science
Must-Reading Tree
Example
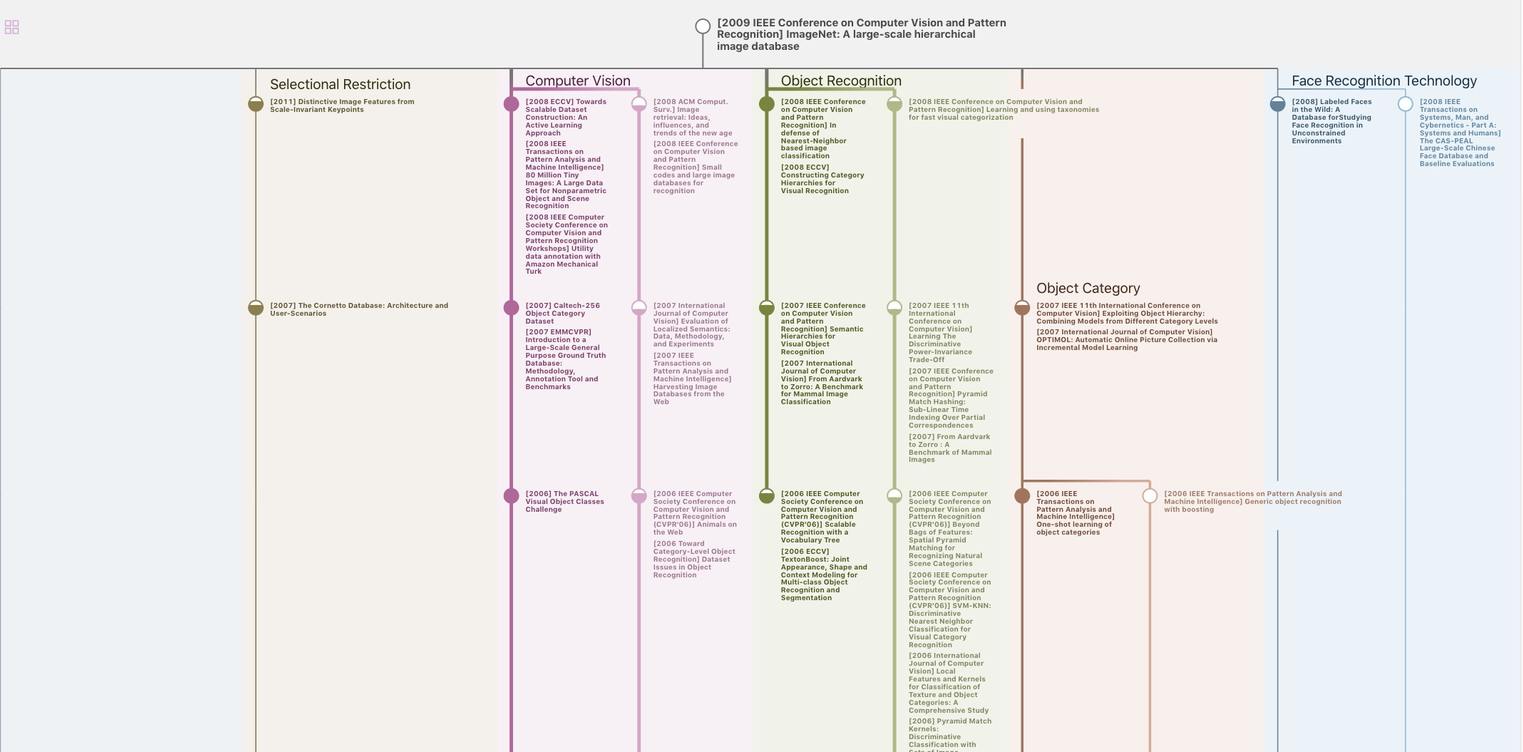
Generate MRT to find the research sequence of this paper
Chat Paper
Summary is being generated by the instructions you defined