DyBit: Dynamic Bit-Precision Numbers for Efficient Quantized Neural Network Inference
IEEE Transactions on Computer-Aided Design of Integrated Circuits and Systems(2023)
摘要
To accelerate the inference of deep neural networks (DNNs), quantization with
low-bitwidth numbers is actively researched. A prominent challenge is to
quantize the DNN models into low-bitwidth numbers without significant accuracy
degradation, especially at very low bitwidths (< 8 bits). This work targets an
adaptive data representation with variable-length encoding called DyBit. DyBit
can dynamically adjust the precision and range of separate bit-field to be
adapted to the DNN weights/activations distribution. We also propose a
hardware-aware quantization framework with a mixed-precision accelerator to
trade-off the inference accuracy and speedup. Experimental results demonstrate
that the inference accuracy via DyBit is 1.997
state-of-the-art at 4-bit quantization, and the proposed framework can achieve
up to 8.1x speedup compared with the original model.
更多查看译文
关键词
Deep Neural Networks,Quantization,Accelerator,FPGAs,Machine Learning
AI 理解论文
溯源树
样例
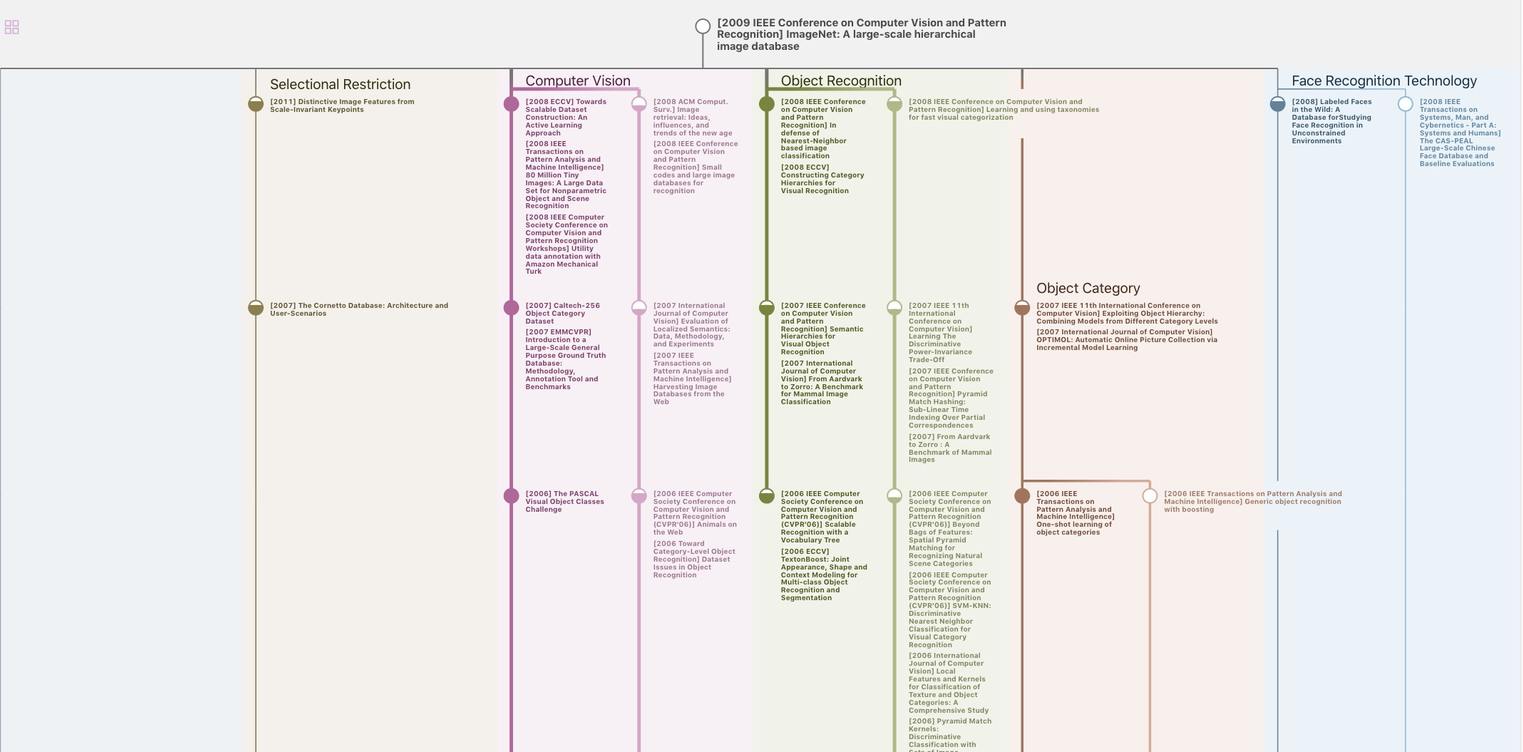
生成溯源树,研究论文发展脉络
Chat Paper
正在生成论文摘要