SEG: Segmentation Evaluation in absence of Ground truth labels.
biorxiv(2023)
摘要
Identifying individual cells or nuclei is often the first step in the analysis of multiplex tissue imaging (MTI) data. Recent efforts to produce plug-and-play, end-to-end MTI analysis tools such as MCMICRO1- though groundbreaking in their usability and extensibility - are often unable to provide users guidance regarding the most appropriate models for their segmentation task among an endless proliferation of novel segmentation methods. Unfortunately, evaluating segmentation results on a user's dataset without ground truth labels is either purely subjective or eventually amounts to the task of performing the original, time-intensive annotation. As a consequence, researchers rely on models pre-trained on other large datasets for their unique tasks. Here, we propose a methodological approach for evaluating MTI nuclei segmentation methods in absence of ground truth labels by scoring relatively to a larger ensemble of segmentations. To avoid potential sensitivity to collective bias from the ensemble approach, we refine the ensemble via weighted average across segmentation methods, which we derive from a systematic model ablation study. First, we demonstrate a proof-of-concept and the feasibility of the proposed approach to evaluate segmentation performance in a small dataset with ground truth annotation. To validate the ensemble and demonstrate the importance of our method-specific weighting, we compare the ensemble's detection and pixel-level predictions - derived without supervision - with the data's ground truth labels. Second, we apply the methodology to an unlabeled larger tissue microarray (TMA) dataset, which includes a diverse set of breast cancer phenotypes, and provides decision guidelines for the general user to more easily choose the most suitable segmentation methods for their own dataset by systematically evaluating the performance of individual segmentation approaches in the entire dataset.
更多查看译文
关键词
segmentation evaluation,seg,ground
AI 理解论文
溯源树
样例
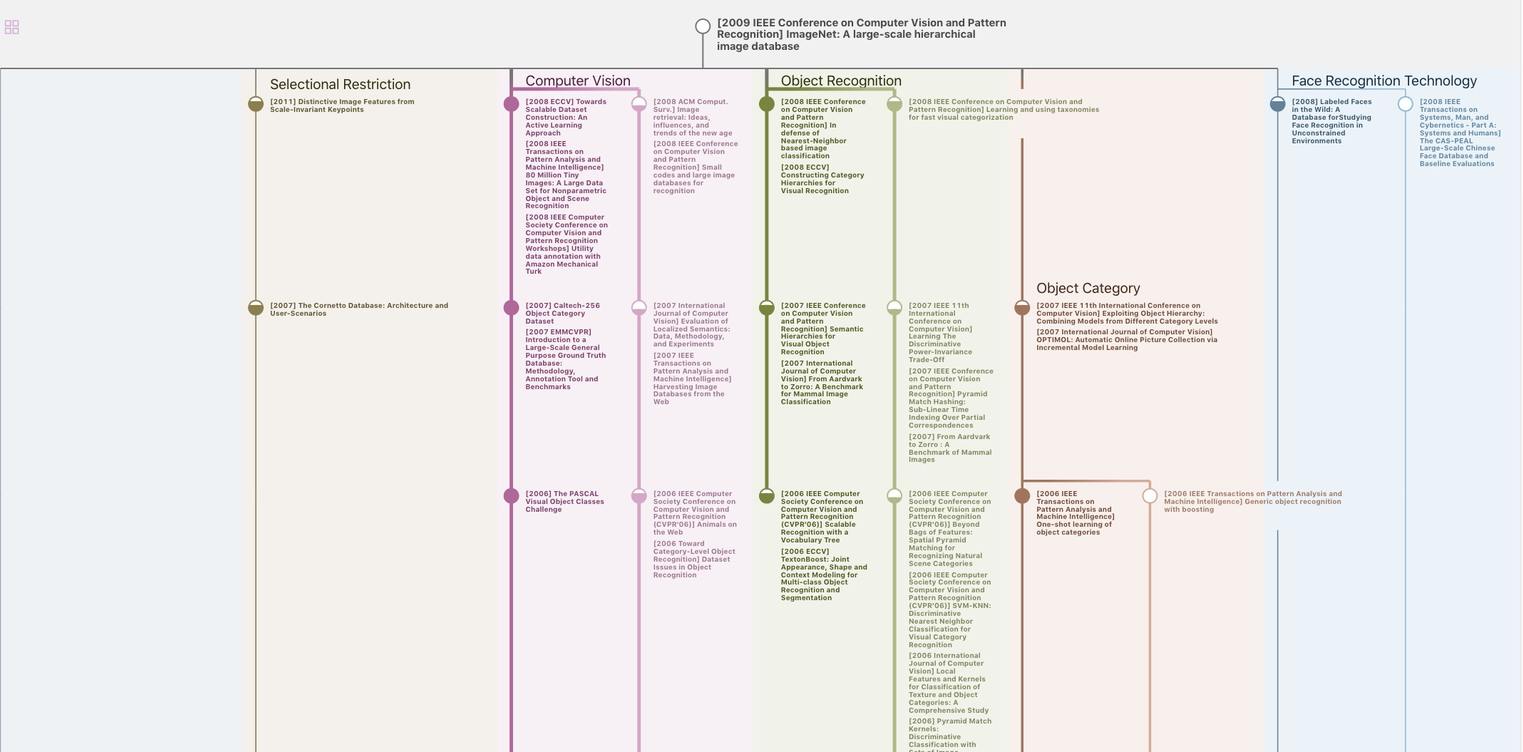
生成溯源树,研究论文发展脉络
Chat Paper
正在生成论文摘要