Accurate Prediction of Soil Heavy Metal Pollution Using an Improved Machine Learning Method: A Case Study in the Pearl River Delta, China.
Environmental science & technology(2023)
摘要
In traditional soil heavy metal (HM) pollution assessment, spatial interpolation analysis is often carried out on the limited sampling points in the study area to get the overall status of heavy metal pollution. Unfortunately, in many machine learning spatial information enhancement algorithms, the additional spatial information introduced fails to reflect the hierarchical heterogeneity of the study area. Therefore, we designed hierarchical regionalization labels based on three interpolation techniques (inverse distance weight, ordinary kriging, and trend surface interpolation) as new spatial covariates for a machine learning (ML) model. It was demonstrated that regional spatial information improved the prediction performance of the model ( > 0.7). On the basis of the prediction results, the status of HM pollution in the Pearl River Delta (PRD) region was evaluated: cadmium (Cd) and copper (Cu) were the most serious pollutants in the PRD (the point overstandard rates are 18.77% and 12.95%, respectively). The analysis of index importance and bivariate local indicators of spatial association (LISA) shows that the key factors affecting the spatial distribution of heavy metals are geographical and climatic conditions [namely, altitude, humidity index, and normalized vegetation difference index (NDVI)] and some industrial activities (such as metal processing, printing and dyeing, and electronics industry). This study develops a novel approach to improve existing spatial interpolation techniques, which will enable more precise and scientific soil environmental management.
更多查看译文
关键词
Accurate prediction,Heavy metals,LISA,Machine learning,Spatial heterogeneity
AI 理解论文
溯源树
样例
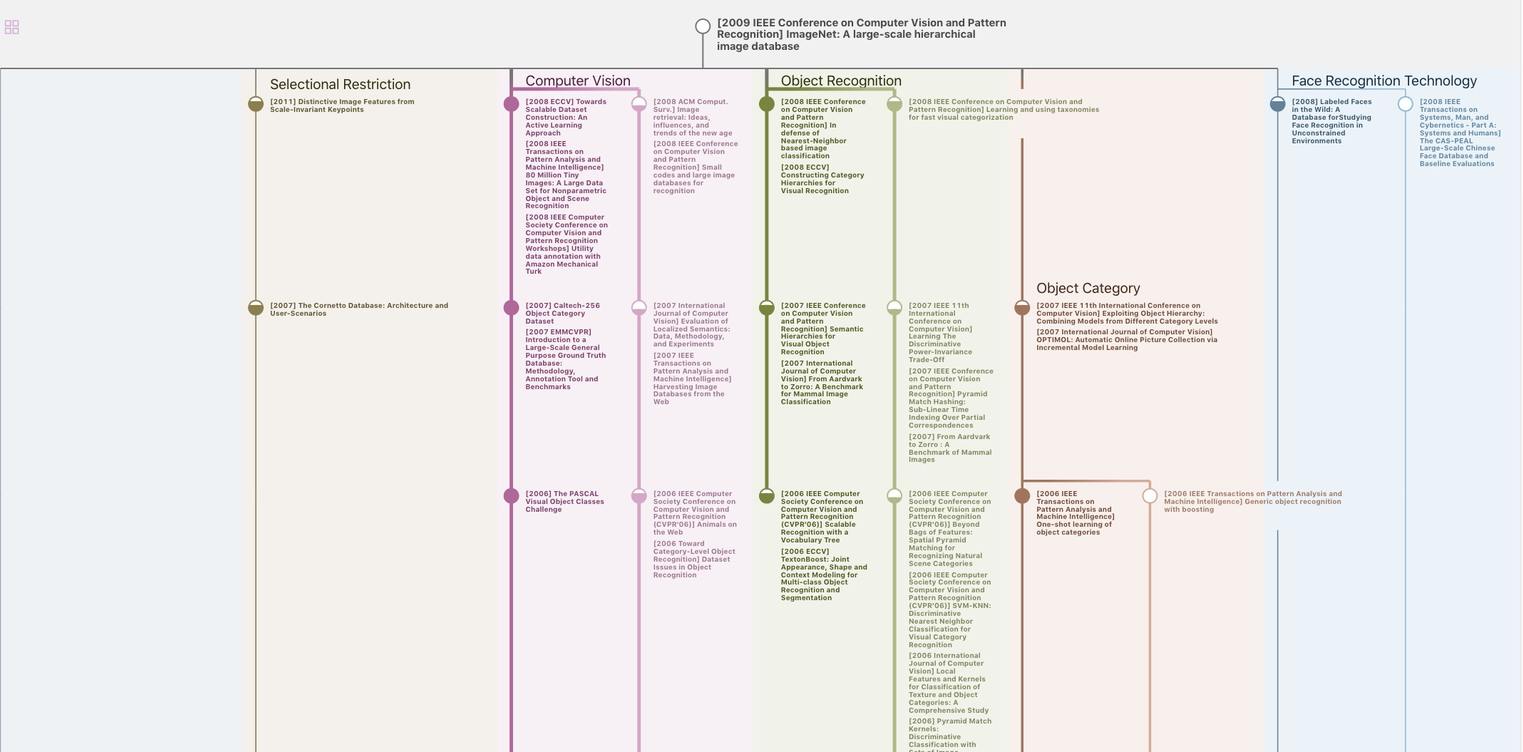
生成溯源树,研究论文发展脉络
Chat Paper
正在生成论文摘要