Deep residual neural network for predicting aerodynamic coefficient changes with ablation
Aerospace Science and Technology(2023)
Abstract
Data-driven methods for predicting aerodynamic coefficients of arbitrary shapes have received considerable attention due to their flexibility and scalability. This paper introduces a deep-learning framework based on a deep residual neural network and K-fold cross-validation for fast and accurate prediction of aerodynamic coefficients of a three-dimensional cone with shape changes due to ablation. The proposed neural network model is trained to learn the underlying relationship between shape transformations and the corresponding changes in aerodynamic coefficients. The shape transformations due to ablation are expressed as the difference between the nominal and ablated cones, measured in units of mesh coordinates. Multiple (K) models constructed based on the training process are combined to reduce the prediction variance effectively. The resulting ensemble model shows an improved prediction performance for various aerodynamic coefficients. To validate our methodology, we compare our model with the generic Multilayer Perceptron (MLP) with a varying number of neurons and the Gaussian process (GP) regression. The test results indicate that the proposed model predicts the aerodynamic coefficients more accurately than the baseline model (MLP/GP), indicating an improved generalization to unseen data.
MoreTranslated text
Key words
Deep neural network,Ablation,Aerodynamic coefficient,Change prediction,K-fold cross validation
AI Read Science
Must-Reading Tree
Example
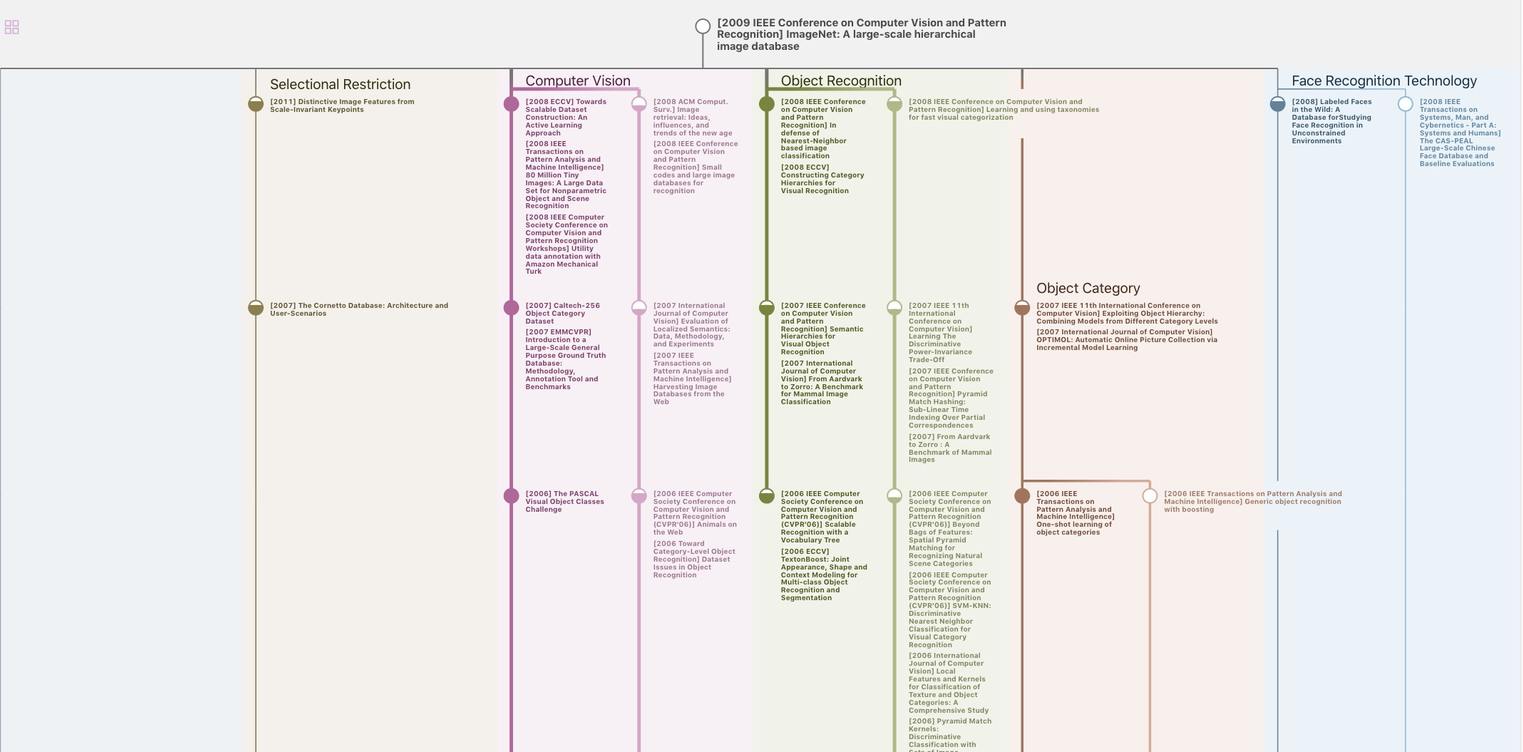
Generate MRT to find the research sequence of this paper
Chat Paper
Summary is being generated by the instructions you defined