DependableHD: A Hyperdimensional Learning Framework for Edge-oriented Voltage-scaled Circuits
2023 28th Asia and South Pacific Design Automation Conference (ASP-DAC)(2023)
摘要
Voltage scaling is one of the most promising approaches for energy efficiency improvement but also brings challenges to fully guaran-teeing the stable operation in modern VLSI. To tackle such issues, we propose DependableHD, a learning framework based on Hy-perDimensional Computing (HDC), which supports the systems to tolerate bit-level memory failure in the low voltage region with high robustness. For the first time, DependableHD introduces the concept of margin enhancement for model retraining and utilizes noise in-jection to improve the robustness, which is capable of application in most state-of-the-art HDC algorithms. Our experiment shows that under 10% memory error, DependableHD exhibits a 1.22% accuracy loss on average, which achieves an 11.2x improvement compared to the baseline HDC solution. The hardware evaluation shows that De-pendableHD supports the systems to reduce the supply voltage from 400mV to 300mV, which provides a 50.41% energy consumption reduction while maintaining competitive accuracy performance.
更多查看译文
关键词
Hyperdimensional Computing,Voltage Scaling,Memory Failure,Noise Injection,Margin Enhancement
AI 理解论文
溯源树
样例
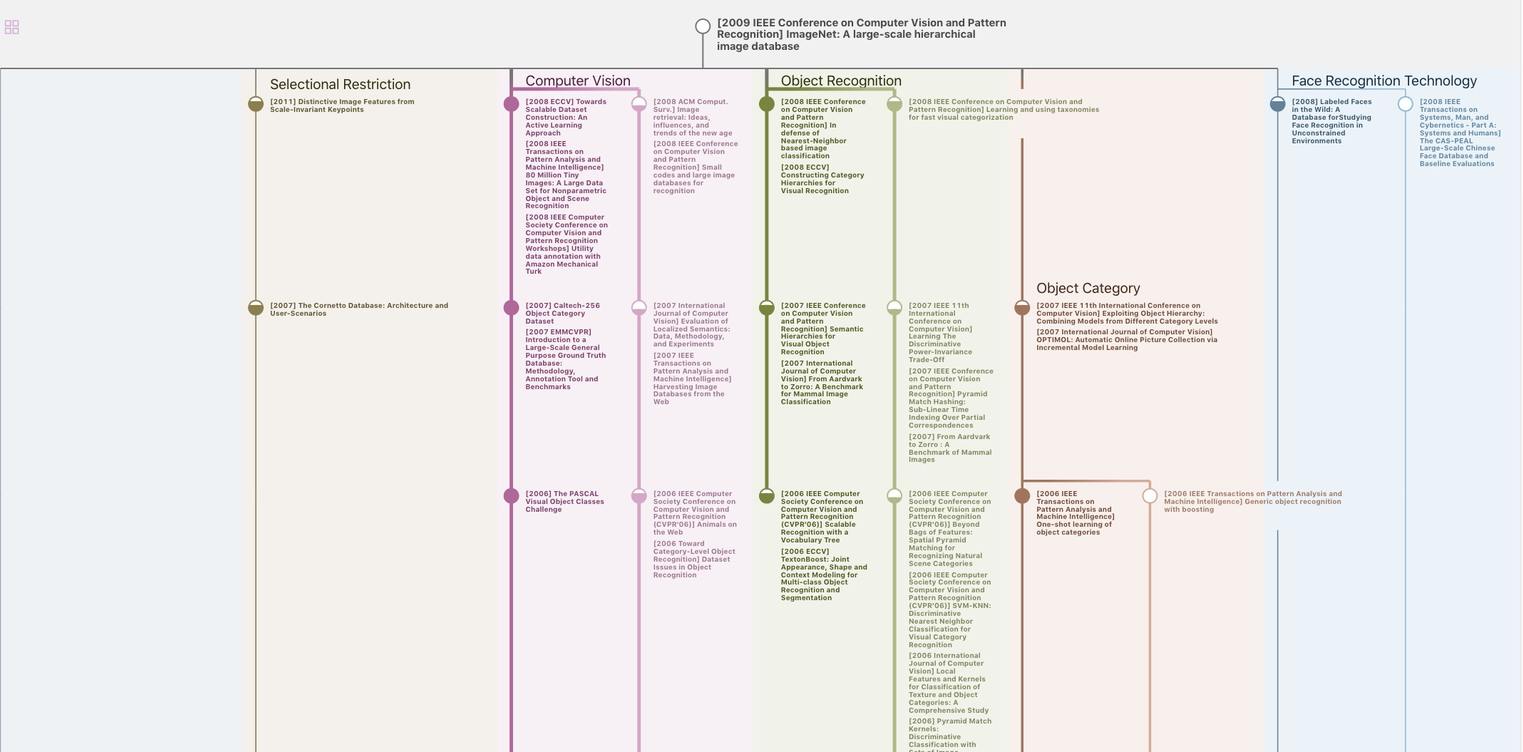
生成溯源树,研究论文发展脉络
Chat Paper
正在生成论文摘要