Toward Energy-Efficient Sparse Matrix-Vector Multiplication with Near STT-MRAM Computing Architecture
2023 28th Asia and South Pacific Design Automation Conference (ASP-DAC)(2023)
摘要
Sparse Matrix-Vector Multiplication (SpMV) is one of the vital computational primitives used in modern workloads. SpMV performs memory access, leading to unnecessary data transmission, massive data access, and redundant multiplicative accumulators. Therefore, we propose the near spin-transfer torque magnetic random access memory (STT-MRAM) processing architecture from three optimization perspectives. These optimizations include (1) the NMP controller receives the instruction through the AXI4 bus to implement the SpMV operation in the following steps, identifies valid data, and encodes the index depending on the kernel size, (2) the NMP controller uses high-level synthesis dataflow in the shared buffer for achieving better performance throughput while do not consume bus bandwidth, and (3) the configurable MACs are implemented in the NMP core without matching step entirely during the multiplication. Using these optimizations, the NMP architecture can access the pipelined STT-MRAM (read bandwidth is 26.7GB/s). The experimental simulation results show that this design achieves up to 66x and 28x speedup compared with state-of-the-art ones and 69x speedup without sparse optimization.
更多查看译文
关键词
energy-efficient,matrix-vector,stt-mram
AI 理解论文
溯源树
样例
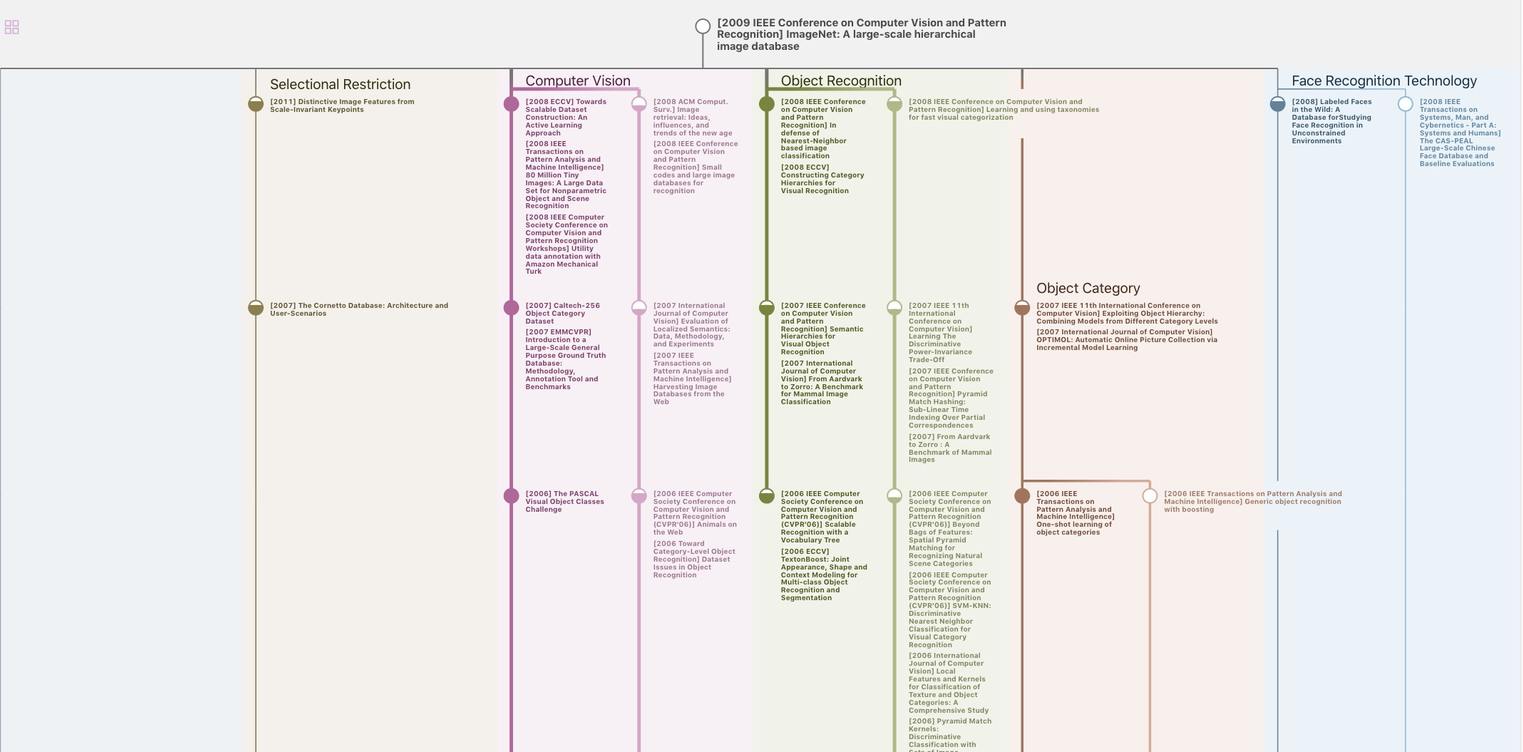
生成溯源树,研究论文发展脉络
Chat Paper
正在生成论文摘要