Reproducibility of deep learning in digital pathology whole slide image analysis.
PLOS digital health(2022)
摘要
For a method to be widely adopted in medical research or clinical practice, it needs to be reproducible so that clinicians and regulators can have confidence in its use. Machine learning and deep learning have a particular set of challenges around reproducibility. Small differences in the settings or the data used for training a model can lead to large differences in the outcomes of experiments. In this work, three top-performing algorithms from the Camelyon grand challenges are reproduced using only information presented in the associated papers and the results are then compared to those reported. Seemingly minor details were found to be critical to performance and yet their importance is difficult to appreciate until the actual reproduction is attempted. We observed that authors generally describe the key technical aspects of their models well but fail to maintain the same reporting standards when it comes to data preprocessing which is essential to reproducibility. As an important contribution of the present study and its findings, we introduce a reproducibility checklist that tabulates information that needs to be reported in histopathology ML-based work in order to make it reproducible.
更多查看译文
关键词
whole slide image analysis,deep learning,digital pathology,image analysis
AI 理解论文
溯源树
样例
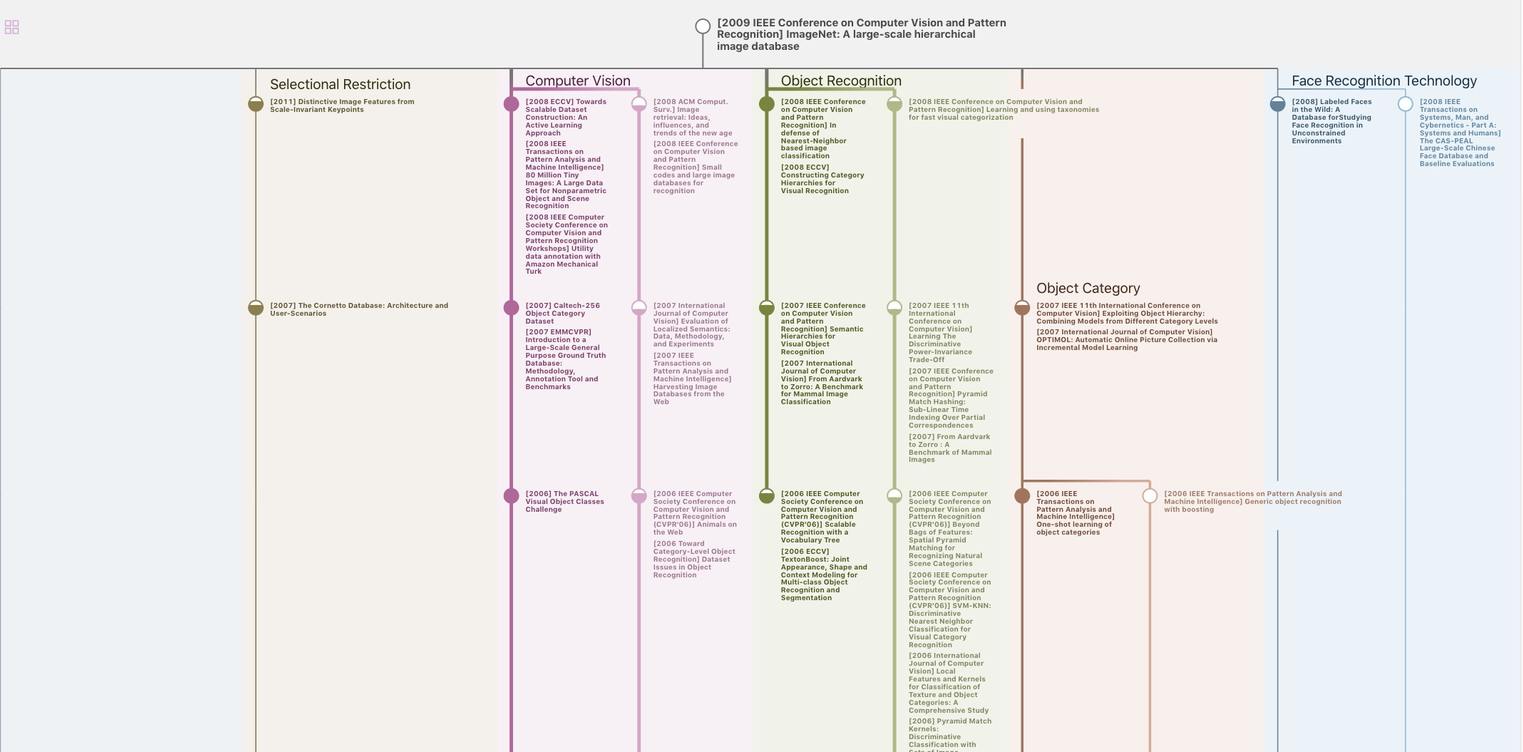
生成溯源树,研究论文发展脉络
Chat Paper
正在生成论文摘要