Variable selection in linear regression models: Choosing the best subset is not always the best choice
BIOMETRICAL JOURNAL(2024)
摘要
We consider the question of variable selection in linear regressions, in the sense of identifying the correct direct predictors (those variables that have nonzero coefficients given all candidate predictors). Best subset selection (BSS) is often considered the "gold standard," with its use being restricted only by its NP-hard nature. Alternatives such as the least absolute shrinkage and selection operator (Lasso) or the Elastic net (Enet) have become methods of choice in high-dimensional settings. A recent proposal represents BSS as a mixed-integer optimization problem so that large problems have become computationally feasible. We present an extensive neutral comparison assessing the ability to select the correct direct predictors of BSS compared to forward stepwise selection (FSS), Lasso, and Enet. The simulation considers a range of settings that are challenging regarding dimensionality (number of observations and variables), signal-to-noise ratios, and correlations between predictors. As fair measure of performance, we primarily used the best possible F1-score for each method, and results were confirmed by alternative performance measures and practical criteria for choosing the tuning parameters and subset sizes. Surprisingly, it was only in settings where the signal-to-noise ratio was high and the variables were uncorrelated that BSS reliably outperformed the other methods, even in low-dimensional settings. Furthermore, FSS performed almost identically to BSS. Our results shed new light on the usual presumption of BSS being, in principle, the best choice for selecting the correct direct predictors. Especially for correlated variables, alternatives like Enet are faster and appear to perform better in practical settings.
更多查看译文
关键词
best subset selection,Lasso,linear regression,mixed-integer optimization,variable selection
AI 理解论文
溯源树
样例
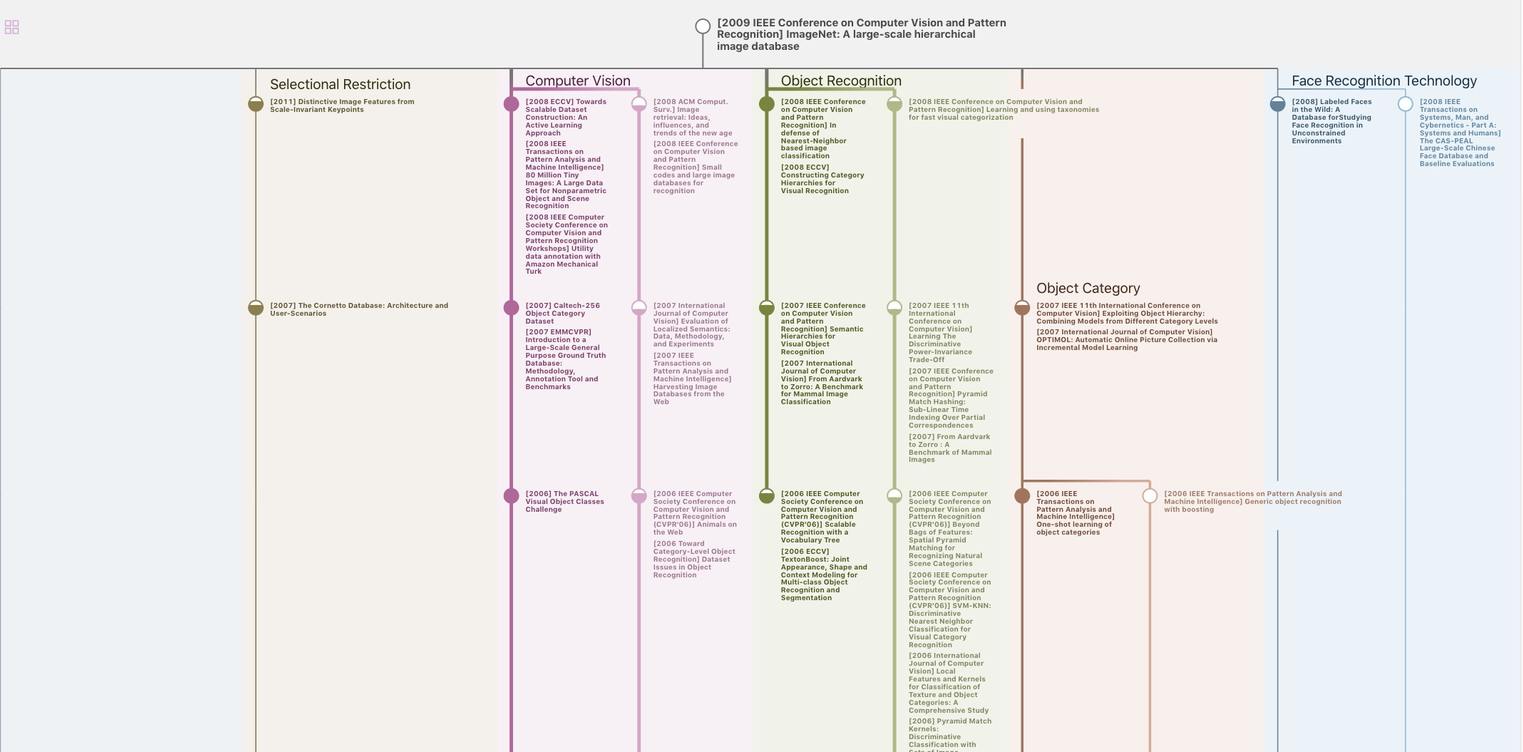
生成溯源树,研究论文发展脉络
Chat Paper
正在生成论文摘要