Gaussian Switch Sampling: A Second Order Approach to Active Learning
arxiv(2023)
摘要
In active learning, acquisition functions define informativeness directly on the representation position within the model manifold. However, for most machine learning models (in particular neural networks) this representation is not fixed due to the training pool fluctuations in between active learning rounds. Therefore, several popular strategies are sensitive to experiment parameters (e.g. architecture) and do not consider model robustness to out-of-distribution settings. To alleviate this issue, we propose a grounded second-order definition of information content and sample importance within the context of active learning. Specifically, we define importance by how often a neural network "forgets" a sample during training - artifacts of second order representation shifts. We show that our definition produces highly accurate importance scores even when the model representations are constrained by the lack of training data. Motivated by our analysis, we develop Gaussian Switch Sampling (GauSS). We show that GauSS is setup agnostic and robust to anomalous distributions with exhaustive experiments on three in-distribution benchmarks, three out-of-distribution benchmarks, and three different architectures. We report an improvement of up to 5% when compared against four popular query strategies.
更多查看译文
关键词
Active learning,example forgetting,learning dynamics
AI 理解论文
溯源树
样例
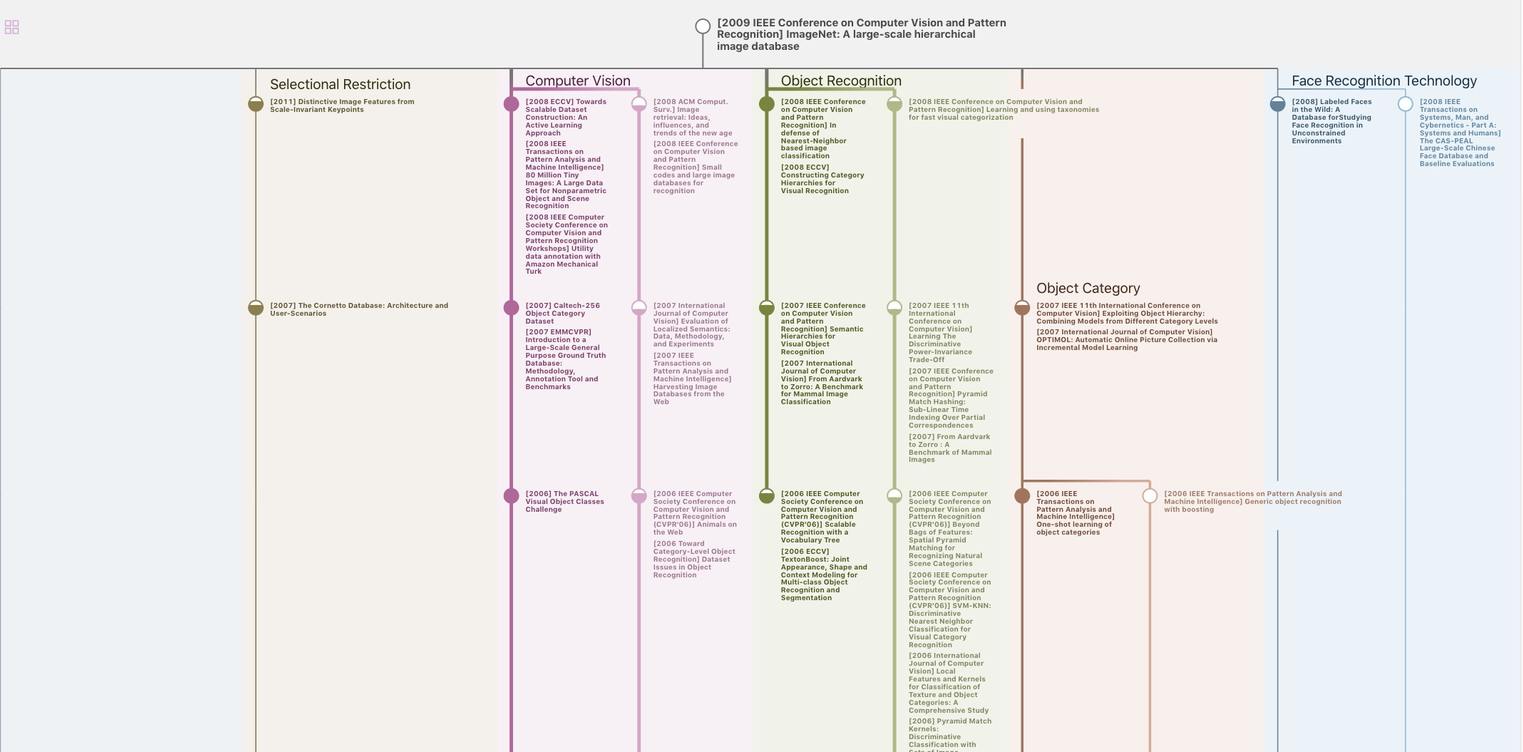
生成溯源树,研究论文发展脉络
Chat Paper
正在生成论文摘要