Semantic-fused multi-granularity cross-city traffic prediction
Transportation Research Part C: Emerging Technologies(2024)
摘要
Accurate traffic prediction is essential for effective urban management and the improvement of transportation efficiency. Recently, data-driven traffic prediction methods have been widely adopted, with better performance than traditional approaches. However, they often require large amounts of data for effective training, which becomes challenging given the prevalence of data scarcity in regions with inadequate sensing infrastructures. To address this issue, we propose a Semantic-Fused Multi-Granularity Transfer Learning (SFMGTL) model to achieve knowledge transfer across cities with fused semantics at different granularities. In detail, we design a semantic fusion module to fuse various semantics while conserving static spatial dependencies via reconstruction losses. Then, a fused graph is constructed based on node features through graph structure learning. Afterwards, we implement hierarchical node clustering to generate graphs with different granularity. To extract feasible meta-knowledge, we further introduce common and private memories and obtain domain-invariant features via adversarial training. It is worth noting that our work jointly addresses semantic fusion and multi-granularity issues in transfer learning. We conduct extensive experiments on six real-world datasets to verify the effectiveness of our SFMGTL model by comparing it with other state-of-the-art baselines. Afterwards, we also perform ablation and case studies, demonstrating that our model possesses substantially fewer parameters compared to baseline models. Moreover, we illustrate how knowledge transfer aids the model in accurately predicting demands, especially during peak hours. The codes can be found at https://github.com/zeonchen/SFMGTL.
更多查看译文
关键词
Traffic prediction,Transfer learning,Graph neural network,Multi-granularity
AI 理解论文
溯源树
样例
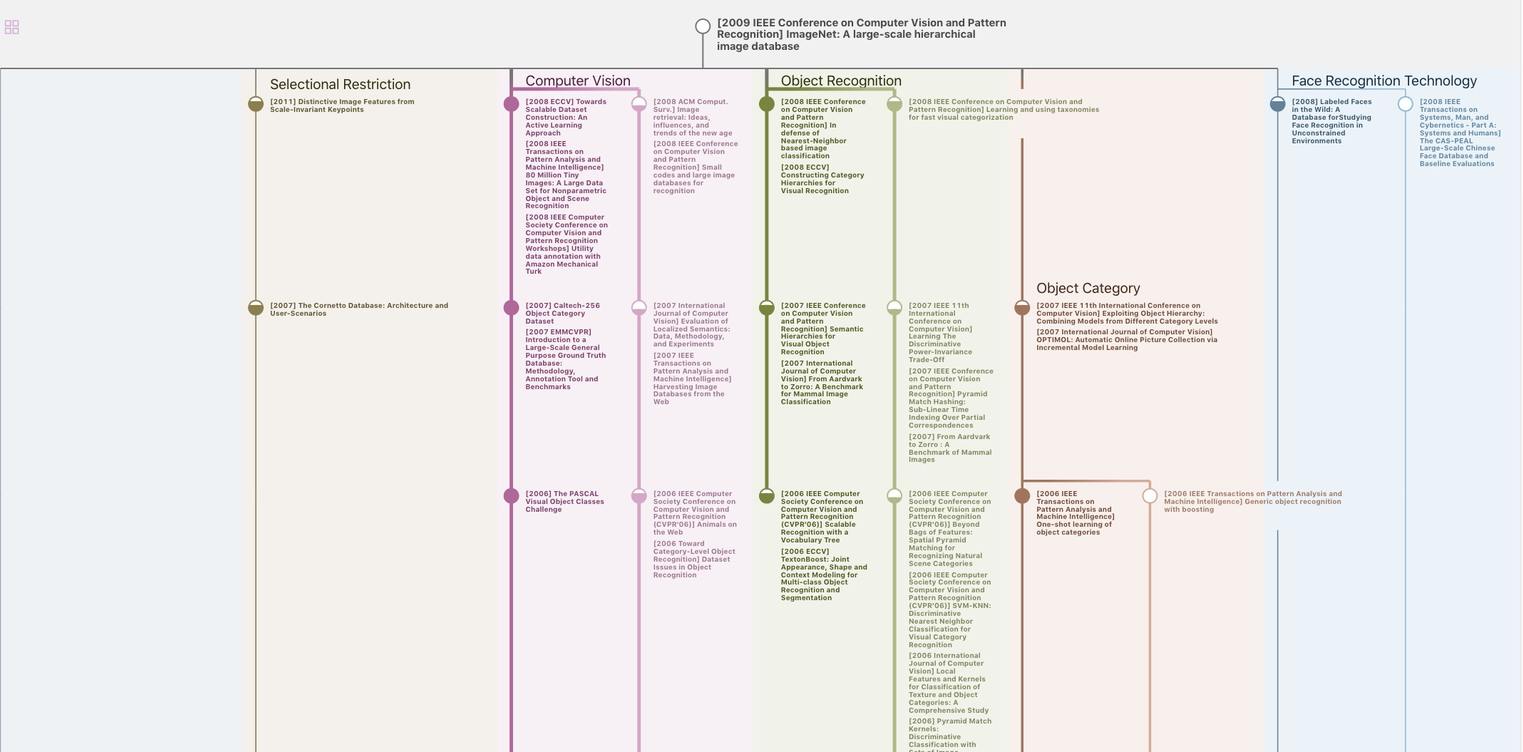
生成溯源树,研究论文发展脉络
Chat Paper
正在生成论文摘要