AlpaServe: Statistical Multiplexing with Model Parallelism for Deep Learning Serving
arxiv(2023)
摘要
Model parallelism is conventionally viewed as a method to scale a single large deep learning model beyond the memory limits of a single device. In this paper, we demonstrate that model parallelism can be additionally used for the statistical multiplexing of multiple devices when serving multiple models, even when a single model can fit into a single device. Our work reveals a fundamental trade-off between the overhead introduced by model parallelism and the opportunity to exploit statistical multiplexing to reduce serving latency in the presence of bursty workloads. We explore the new trade-off space and present a novel serving system, AlpaServe, that determines an efficient strategy for placing and parallelizing collections of large deep learning models across a distributed cluster. Evaluation results on production workloads show that AlpaServe can process requests at up to 10x higher rates or 6x more burstiness while staying within latency constraints for more than 99% of requests.
更多查看译文
关键词
statistical multiplexing,model parallelism,deep
AI 理解论文
溯源树
样例
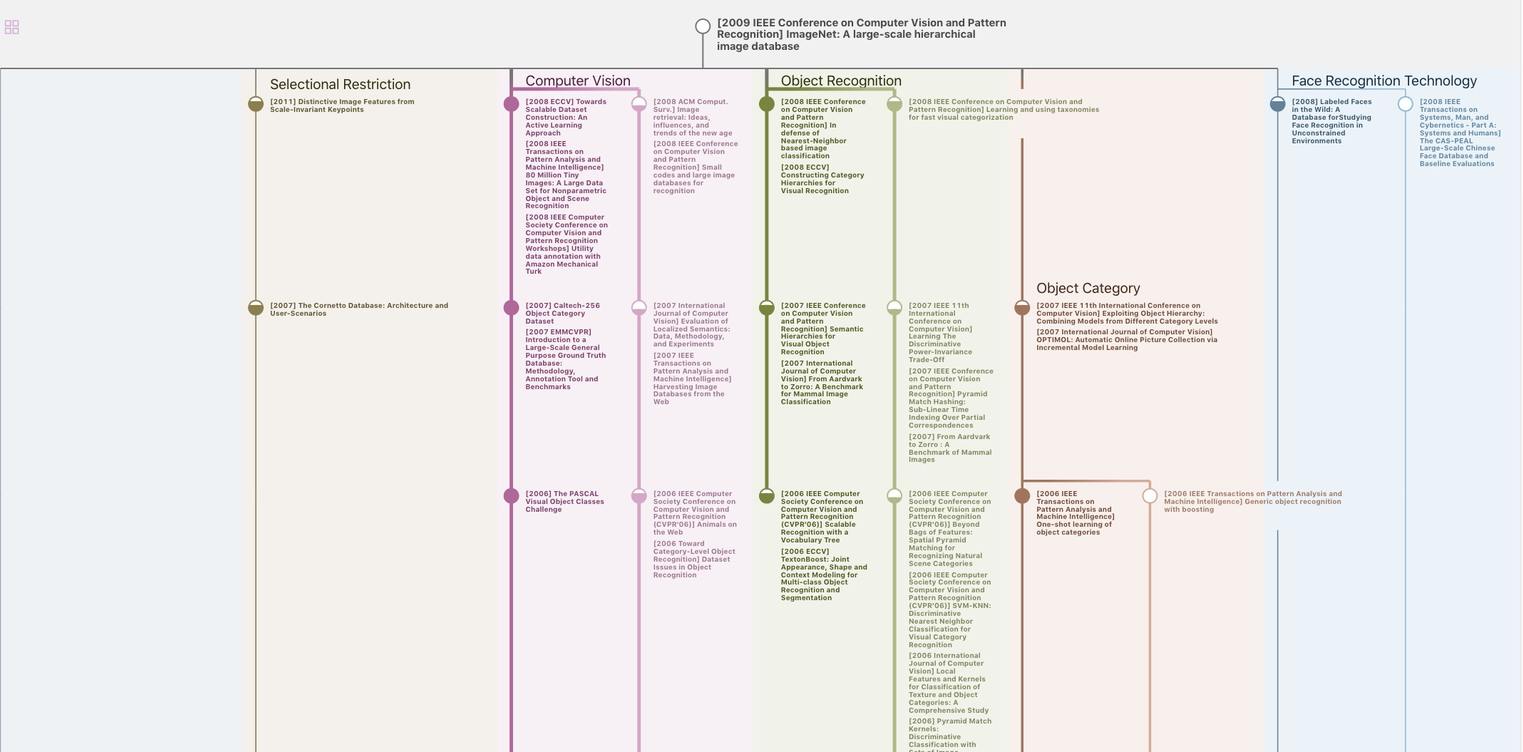
生成溯源树,研究论文发展脉络
Chat Paper
正在生成论文摘要