Brain-constrained neural modeling explains fast mapping of words to meaning
Cerebral cortex (New York, N.Y. : 1991)(2023)
摘要
Although teaching animals a few meaningful signs is usually time-consuming, children acquire words easily after only a few exposures, a phenomenon termed "fast-mapping." Meanwhile, most neural network learning algorithms fail to achieve reliable information storage quickly, raising the question of whether a mechanistic explanation of fast-mapping is possible. Here, we applied brain-constrained neural models mimicking fronto-temporal-occipital regions to simulate key features of semantic associative learning. We compared networks (i) with prior encounters with phonological and conceptual knowledge, as claimed by fast-mapping theory, and (ii) without such prior knowledge. Fast-mapping simulations showed word-specific representations to emerge quickly after 1-10 learning events, whereas direct word learning showed word-meaning mappings only after 40-100 events. Furthermore, hub regions appeared to be essential for fast-mapping, and attention facilitated it, but was not strictly necessary. These findings provide a better understanding of the critical mechanisms underlying the human brain's unique ability to acquire new words rapidly.
更多查看译文
关键词
Hebbian learning,biologically neural networks,distributed neural assemblies,fast mapping,language acquisition,semantic grounding
AI 理解论文
溯源树
样例
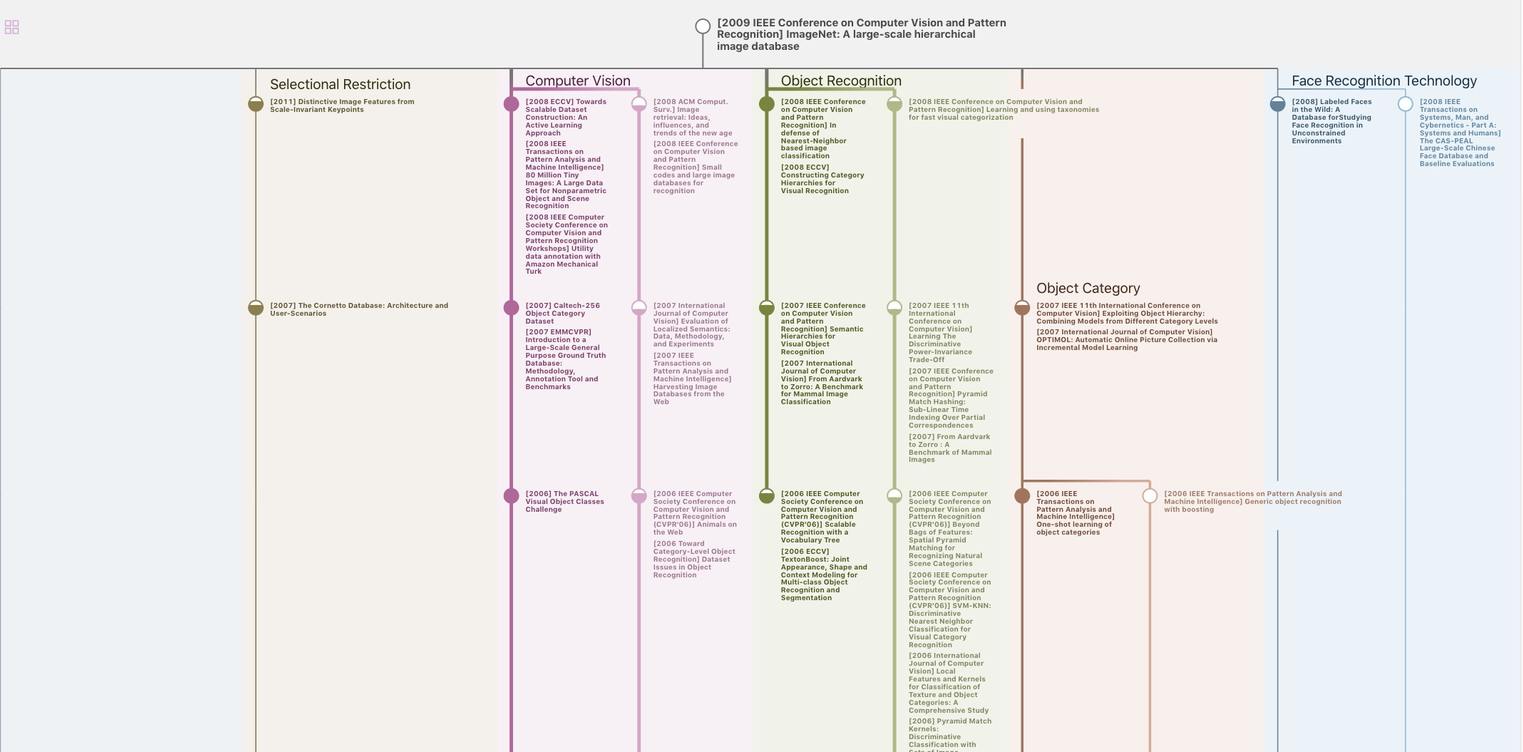
生成溯源树,研究论文发展脉络
Chat Paper
正在生成论文摘要