The DetectDeviatingCells algorithm was a useful addition to the toolkit for cellwise error detection in observational data.
Journal of clinical epidemiology(2023)
摘要
OBJECTIVES:We evaluated the error detection performance of the DetectDeviatingCells (DDC) algorithm which flags data anomalies at observation (casewise) and variable (cellwise) level in continuous variables. We compared its performance to other approaches in a simulated dataset.
STUDY DESIGN AND SETTING:We simulated height and weight data for hypothetical individuals aged 2-20 years. We changed a proportion of height values according to predetermined error patterns. We applied the DDC algorithm and other error-detection approaches (descriptive statistics, plots, fixed-threshold rules, classic, and robust Mahalanobis distance) and we compared error detection performance with sensitivity, specificity, likelihood ratios, predictive values, and receiver operating characteristic (ROC) curves.
RESULTS:At our chosen thresholds error detection specificity was excellent across all scenarios for all methods and sensitivity was higher for multivariable and robust methods. The DDC algorithm performance was similar to other robust multivariable methods. Analysis of ROC curves suggested that all methods had comparable performance for gross errors (e.g., wrong measurement unit), but the DDC algorithm outperformed the others for more complex error patterns (e.g., transcription errors that are still plausible, although extreme).
CONCLUSIONS:The DDC algorithm has the potential to improve error detection processes for observational data.
更多查看译文
AI 理解论文
溯源树
样例
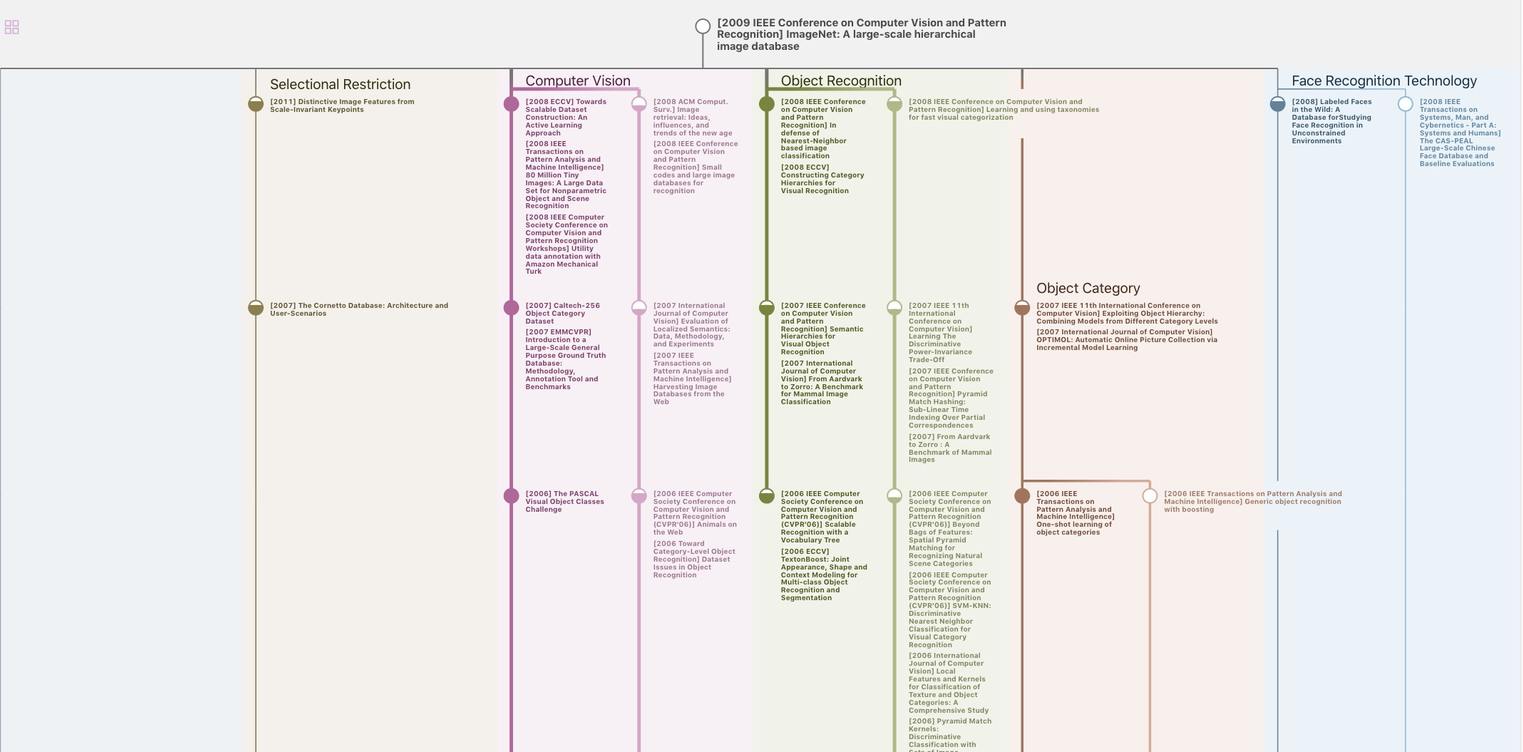
生成溯源树,研究论文发展脉络
Chat Paper
正在生成论文摘要