A hybrid rough set shuffled frog leaping knowledge inference system for diagnosis of lung cancer disease.
Computers in biology and medicine(2023)
Abstract
Abundant medical data are generated in the digital world every second. However, gathering helpful information from these data is difficult. Gathering useful information from the dataset is very advantageous and demanding. Besides, such data also contain many extraneous features that do not influence the foreboding accuracy while diagnosing a disease. The data must eliminate these extraneous features to get a better diagnosis. Ultimately, the minimized information system will lead to a better diagnosis. In this paper, we have introduced an incremental rough set shuffled frog leaping algorithm for knowledge inference. The proposed algorithm helps find minimum features from an information system while handling complex databases with uncertainty and incompleteness. The proposed rough set shuffled frog leaping knowledge inference model works in two phases. In the initial phase, the incremental rough set shuffled frog leaping algorithm is used to get the most relevant features. Identifying the relevant features is carried out using a fitness function, which uses the rough degree of dependency. The use of the fitness function identifies the much information with the minimum number of features. The purpose of feature selection is to identify a feature subset from an original set of features without reducing the predictive accuracy and to scale back the computation overhead in the data processing. In the second phase, a rough set is utilized for knowledge discovery in perception with rule generation. The selection of decision rules is carried out based on the accuracy of the decision rule and a predefined threshold value. An empirical analysis of the lung disease information system and a comparative study is conducted. Experimental outcomes exhibit that hybrid techniques express the feasibility of the proposed model while achieving better classification accuracy.
MoreTranslated text
Key words
Approximation,Classification,Feature selection,Knowledge discovery,Positive region,Rule generation,Shuffled frog leaping
AI Read Science
Must-Reading Tree
Example
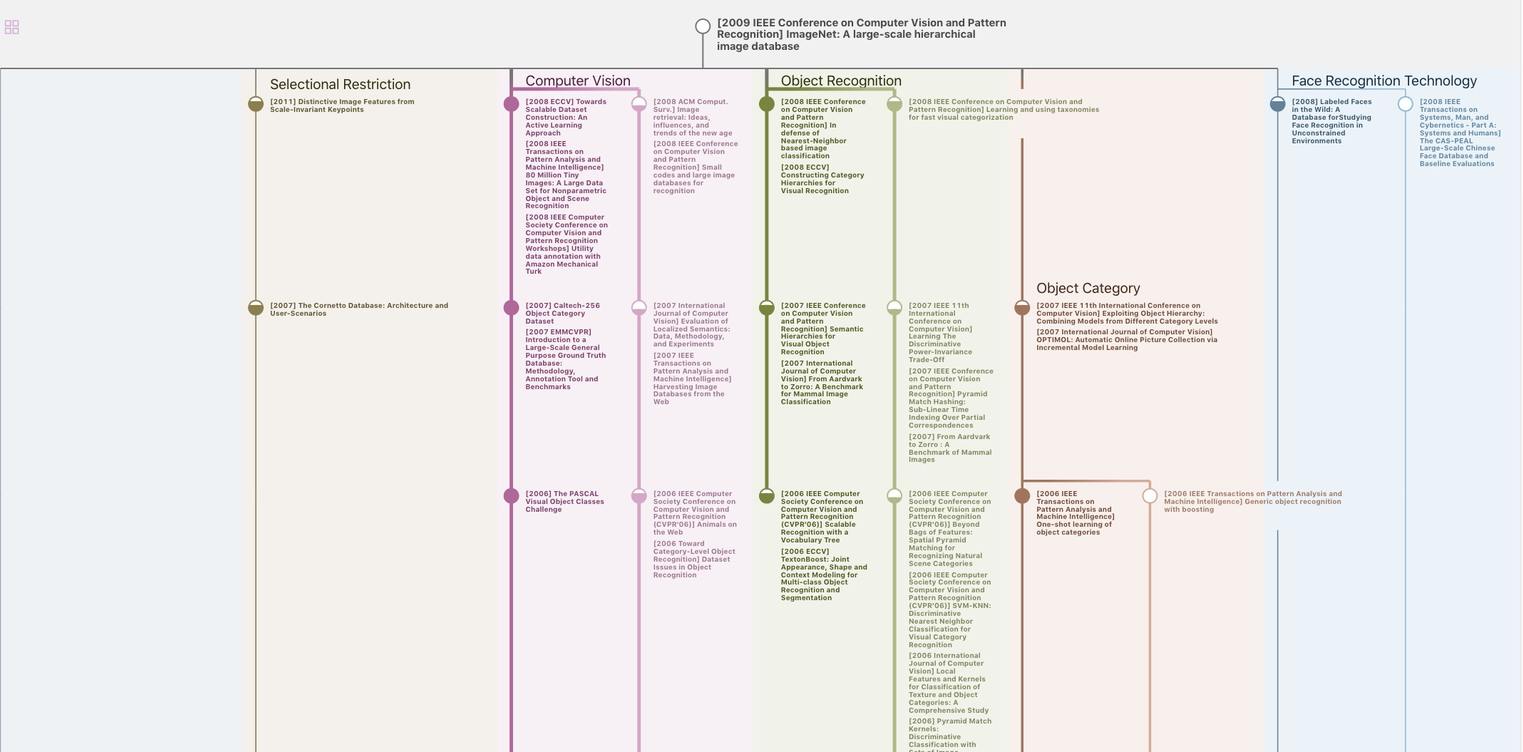
Generate MRT to find the research sequence of this paper
Chat Paper
Summary is being generated by the instructions you defined