Closed-Loop and Artificial Intelligence-Based Decision Support Systems.
DIABETES TECHNOLOGY & THERAPEUTICS(2023)
关键词
Continuous Glucose Monitoring,Diabetes,Type 1 Diabetes
AI 理解论文
溯源树
样例
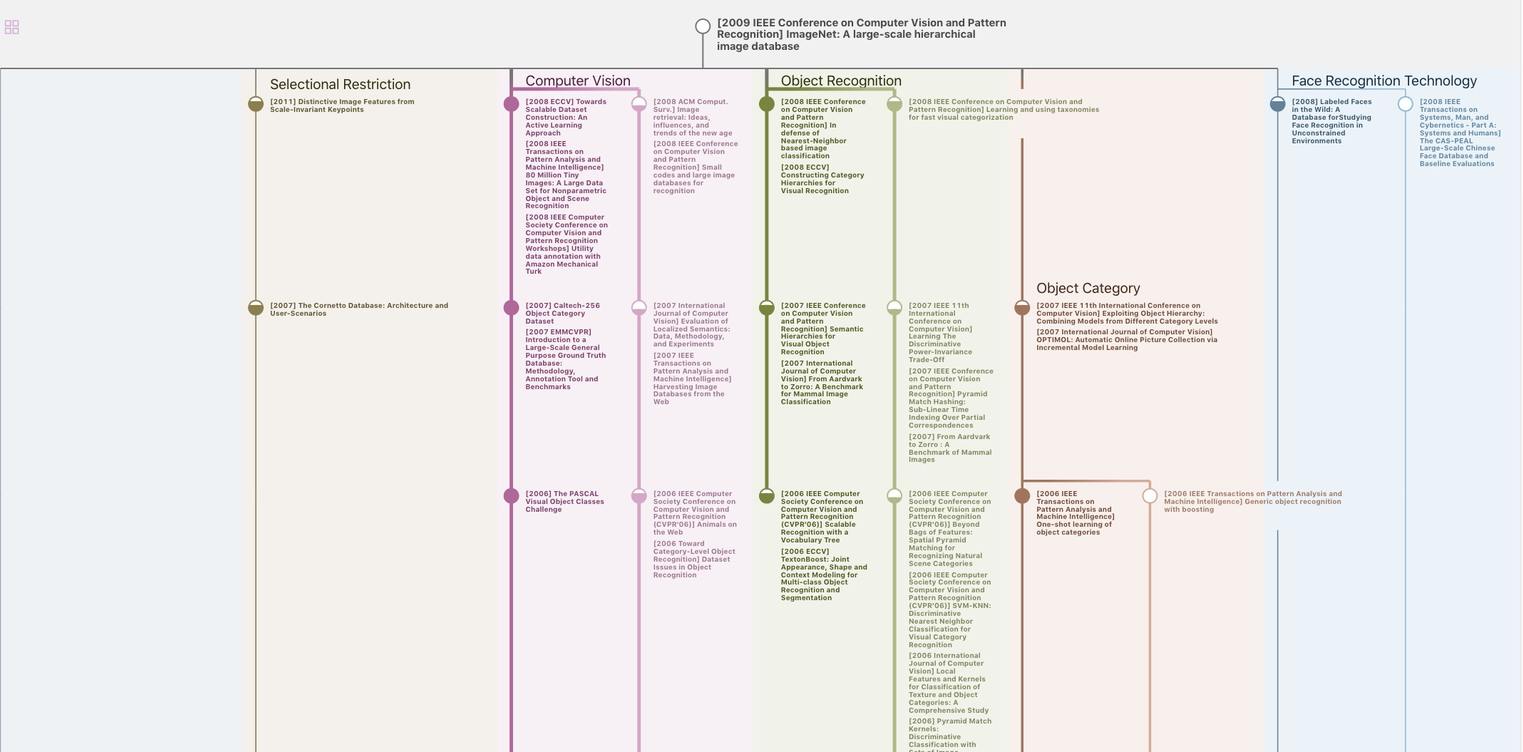
生成溯源树,研究论文发展脉络
Chat Paper
正在生成论文摘要
DIABETES TECHNOLOGY & THERAPEUTICS(2023)