One-Pot Multi-frame Denoising
International Journal of Computer Vision(2024)
摘要
The efficacy of learning-based denoising techniques is heavily reliant on the quality of clean supervision. Unfortunately, acquiring clean images in many scenarios is a challenging task. Conversely, capturing multiple noisy frames of the same field of view is feasible and natural in real-life scenarios. Thus, it is imperative to explore the potential of noisy data in model training and avoid the limitations imposed by clean labels. In this paper, we propose a novel unsupervised learning strategy called one-pot denoising (OPD), which is the first unsupervised multi-frame denoising method. OPD differs from traditional supervision schemes, such as supervised Noise2Clean, unsupervised Noise2Noise, and self-supervised Noise2Void, as it employs mutual supervision among all the multiple frames. This provides learning with more diverse supervision and allows models to better exploit the correlation among frames. Notably, we reveal that Noise2Noise is a special case of the proposed OPD. We provide two specific implementations, namely OPD-random coupling and OPD-alienation loss, to achieve OPD during model training based on data allocation and loss refine, respectively. Our experiments demonstrate that OPD outperforms other unsupervised denoising methods and is comparable to non-transformer-based supervised N2C methods for several classic noise patterns, including additive white Gaussian noise, signal-dependent Poisson noise, and multiplicative Bernoulli noise. Additionally, OPD shows remarkable performance in more challenging tasks such as mixed-blind denoising, denoising random-valued impulse noise, and text removal. The source code and pre-trained models are available at https://github.com/LujiaJin/One-Pot_Multi-Frame_Denoising .
更多查看译文
关键词
One-pot denoising,Multi-frame denoising,Unsupervised learning,Random coupling,Alienation loss
AI 理解论文
溯源树
样例
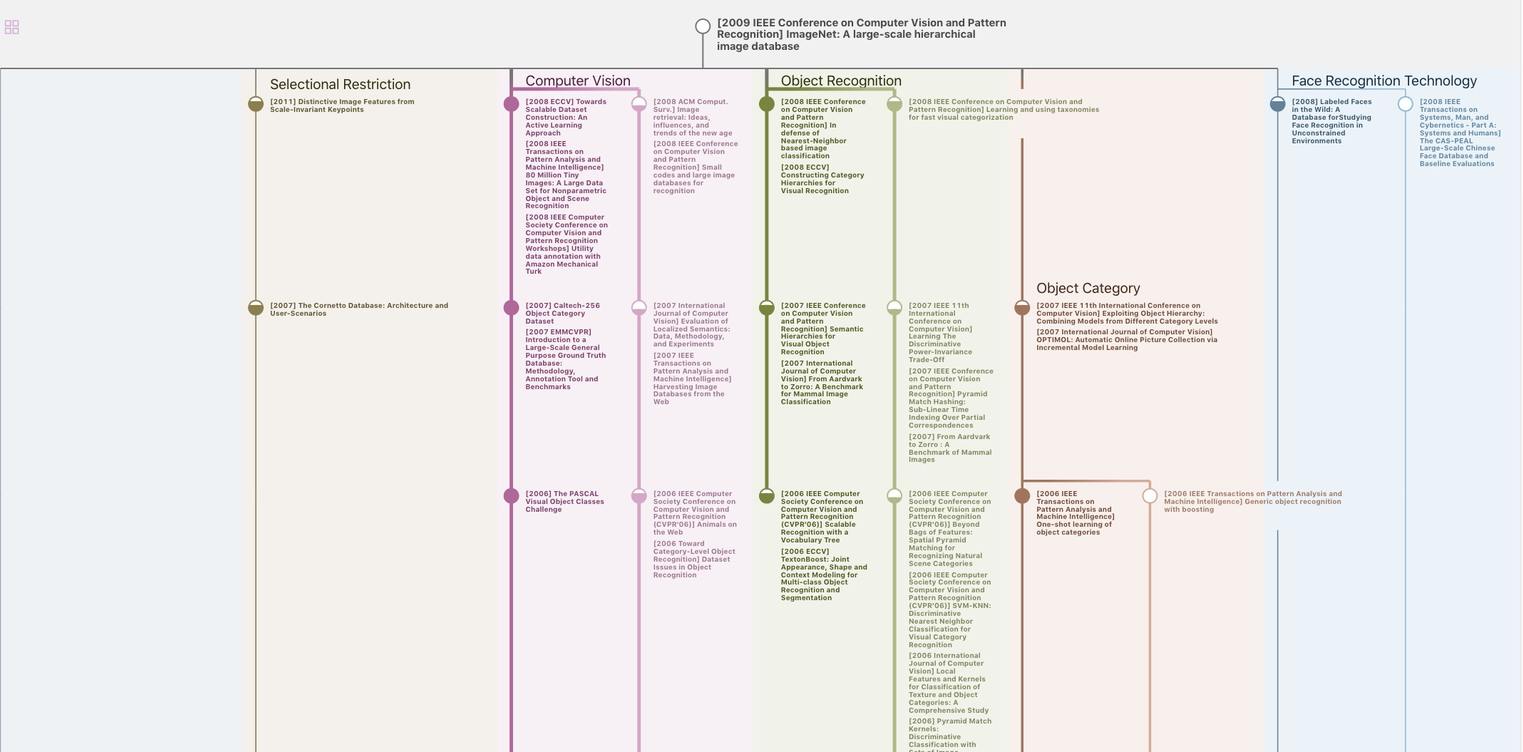
生成溯源树,研究论文发展脉络
Chat Paper
正在生成论文摘要