Neural Network Analytic Continuation for Monte Carlo: Improvement by Statistical Errors
arxiv(2023)
摘要
This study explores the use of neural network-based analytic continuation to extract spectra from Monte Carlo data. We apply this technique to both synthetic and Monte Carlo-generated data. The training sets for neural networks are carefully synthesized without ``data leakage". We found that the training set should match the input correlation functions in terms of statistical error properties, such as noise level, noise dependence on imaginary time, and imaginary time-displaced correlations. We have developed a systematic method to synthesize such training datasets. Our improved algorithm outperform the widely used maximum entropy method in highly noisy situations. As an example, our method successfully extracted the dynamic structure factor of the spin-1/2 Heisenberg chain from quantum Monte Carlo simulations.
更多查看译文
关键词
neural network,analytic continuation,quantum Monte Carlo
AI 理解论文
溯源树
样例
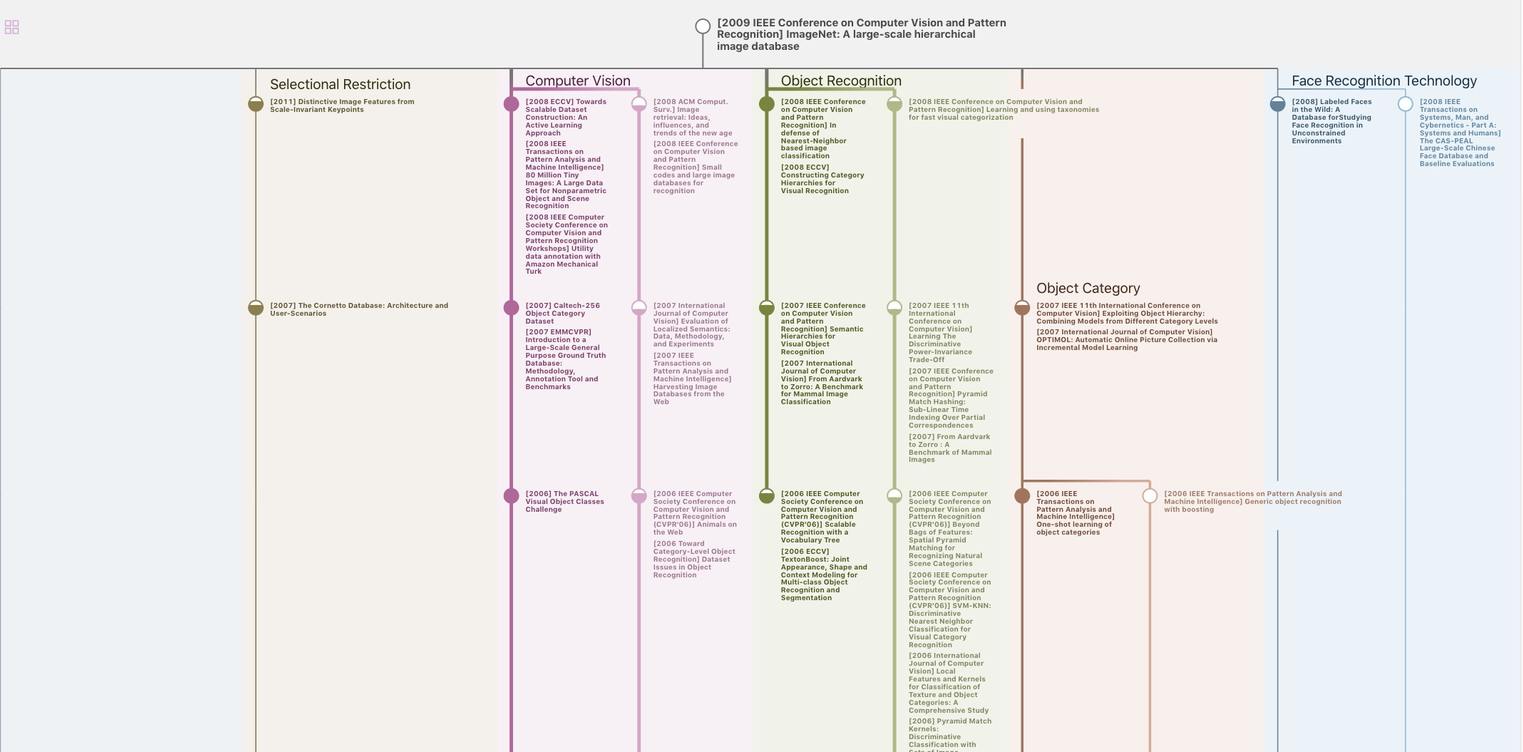
生成溯源树,研究论文发展脉络
Chat Paper
正在生成论文摘要