Deep Kernel Principal Component Analysis for multi-level feature learning
NEURAL NETWORKS(2024)
摘要
Principal Component Analysis (PCA) and its nonlinear extension Kernel PCA (KPCA) are widely used across science and industry for data analysis and dimensionality reduction. Modern deep learning tools have achieved great empirical success, but a framework for deep principal component analysis is still lacking. Here we develop a deep kernel PCA methodology (DKPCA) to extract multiple levels of the most informative components of the data. Our scheme can effectively identify new hierarchical variables, called deep principal components, capturing the main characteristics of high-dimensional data through a simple and interpretable numerical optimization. We couple the principal components of multiple KPCA levels, theoretically showing that DKPCA creates both forward and backward dependency across levels, which has not been explored in kernel methods and yet is crucial to extract more informative features. Various experimental evaluations on multiple data types show that DKPCA finds more efficient and disentangled representations with higher explained variance in fewer principal components, compared to the shallow KPCA. We demonstrate that our method allows for effective hierarchical data exploration, with the ability to separate the key generative factors of the input data both for large datasets and when few training samples are available. Overall, DKPCA can facilitate the extraction of useful patterns from high-dimensional data by learning more informative features organized in different levels, giving diversified aspects to explore the variation factors in the data, while maintaining a simple mathematical formulation.
更多查看译文
关键词
Kernel Principal Component Analysis,Deep learning,Generative models,Manifold optimization
AI 理解论文
溯源树
样例
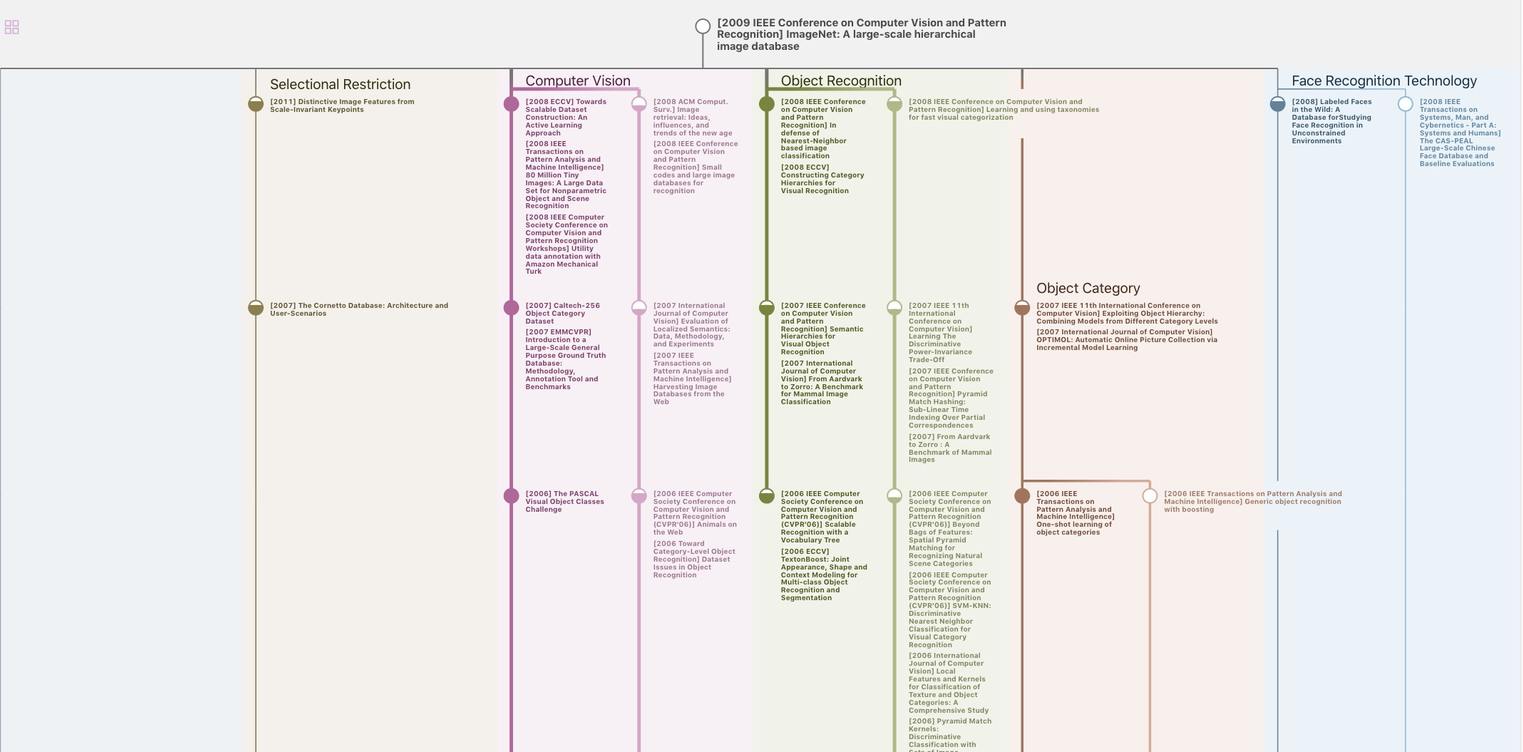
生成溯源树,研究论文发展脉络
Chat Paper
正在生成论文摘要