Deep Neural Networks for Encrypted Inference with TFHE.
IACR Cryptol. ePrint Arch.(2023)
摘要
Fully homomorphic encryption (FHE) is an encryption method that allows to perform computation on encrypted data, without decryption. FHE preserves the privacy of the users of online services that handle sensitive data, such as health data, biometrics, credit scores and other personal information. A common way to provide a valuable service on such data is through machine learning and, at this time, Neural Networks are the dominant machine learning model for unstructured data. In this work we show how to construct Deep Neural Networks (DNN) that are compatible with the constraints of TFHE, an FHE scheme that allows arbitrary depth computation circuits. We discuss the constraints and show the architecture of DNNs for two computer vision tasks. We benchmark the architectures using the Concrete stack, an open-source implementation of TFHE.
更多查看译文
关键词
encrypted inference,neural networks,tfhe
AI 理解论文
溯源树
样例
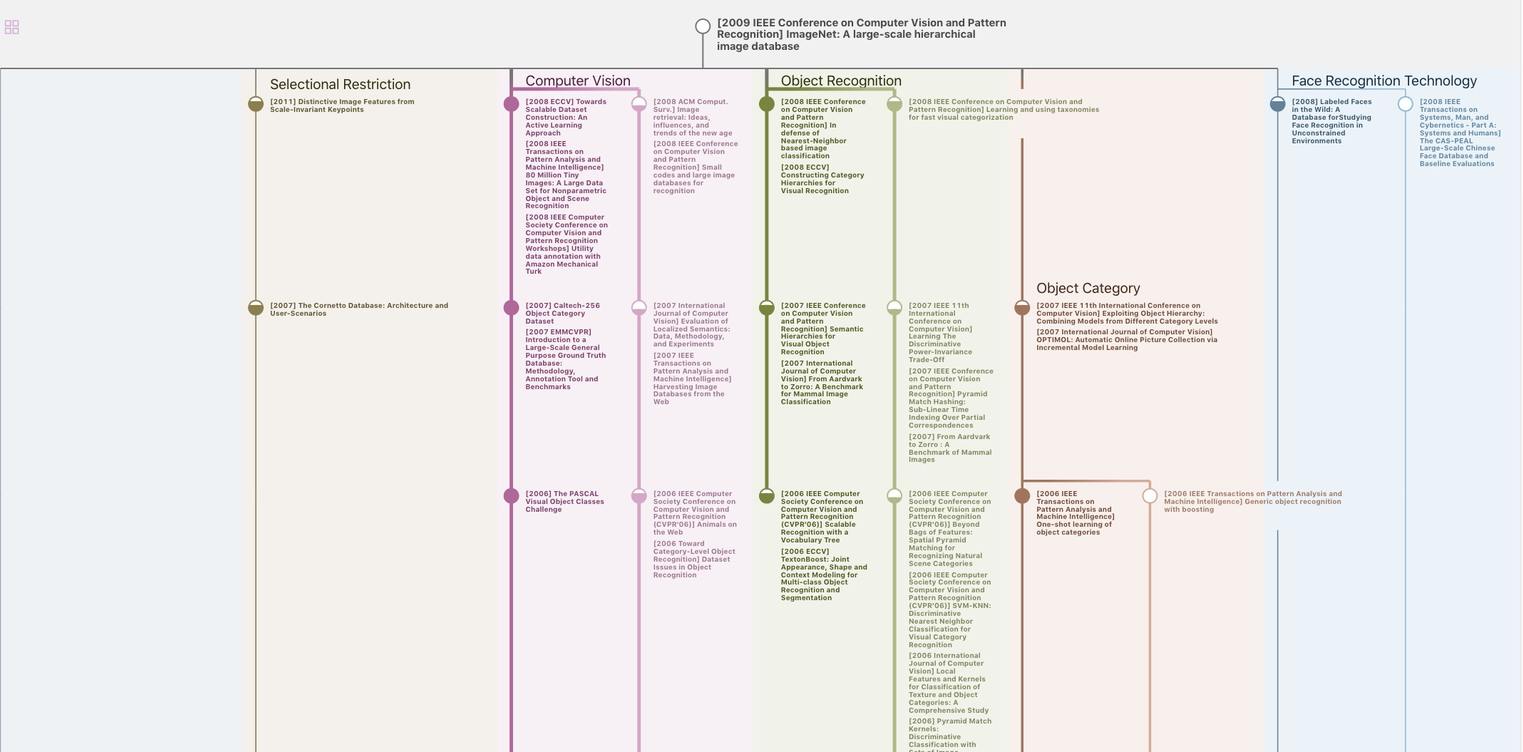
生成溯源树,研究论文发展脉络
Chat Paper
正在生成论文摘要