Machine Learning Techniques for Predicting the Short-Term Outcome of Resective Surgery in Lesional-Drug Resistance Epilepsy
CoRR(2023)
摘要
In this study, we developed and tested machine learning models to predict epilepsy surgical outcome using noninvasive clinical and demographic data from patients. Methods: Seven dif-ferent categorization algorithms were used to analyze the data. The techniques are also evaluated using the Leave-One-Out method. For precise evaluation of the results, the parameters accuracy, precision, recall and, F1-score are calculated. Results: Our findings revealed that a machine learning-based presurgical model of patients' clinical features may accurately predict the outcome of epilepsy surgery in patients with drug-resistant lesional epilepsy. The support vector machine (SVM) with the linear kernel yielded 76.1% in terms of accuracy could predict results in 96.7% of temporal lobe epilepsy (TLE) patients and 79.5% of extratemporal lobe epilepsy (ETLE) cases using ten clinical features. Significance: To predict the outcome of epilepsy surgery, this study recommends the use of a machine learning strategy based on supervised classification and se-lection of feature subsets data mining. Progress in the development of machine learning-based prediction models offers optimism for personalised medicine access.
更多查看译文
关键词
epilepsy,machine learning,resective surgery,short-term,lesional-drug
AI 理解论文
溯源树
样例
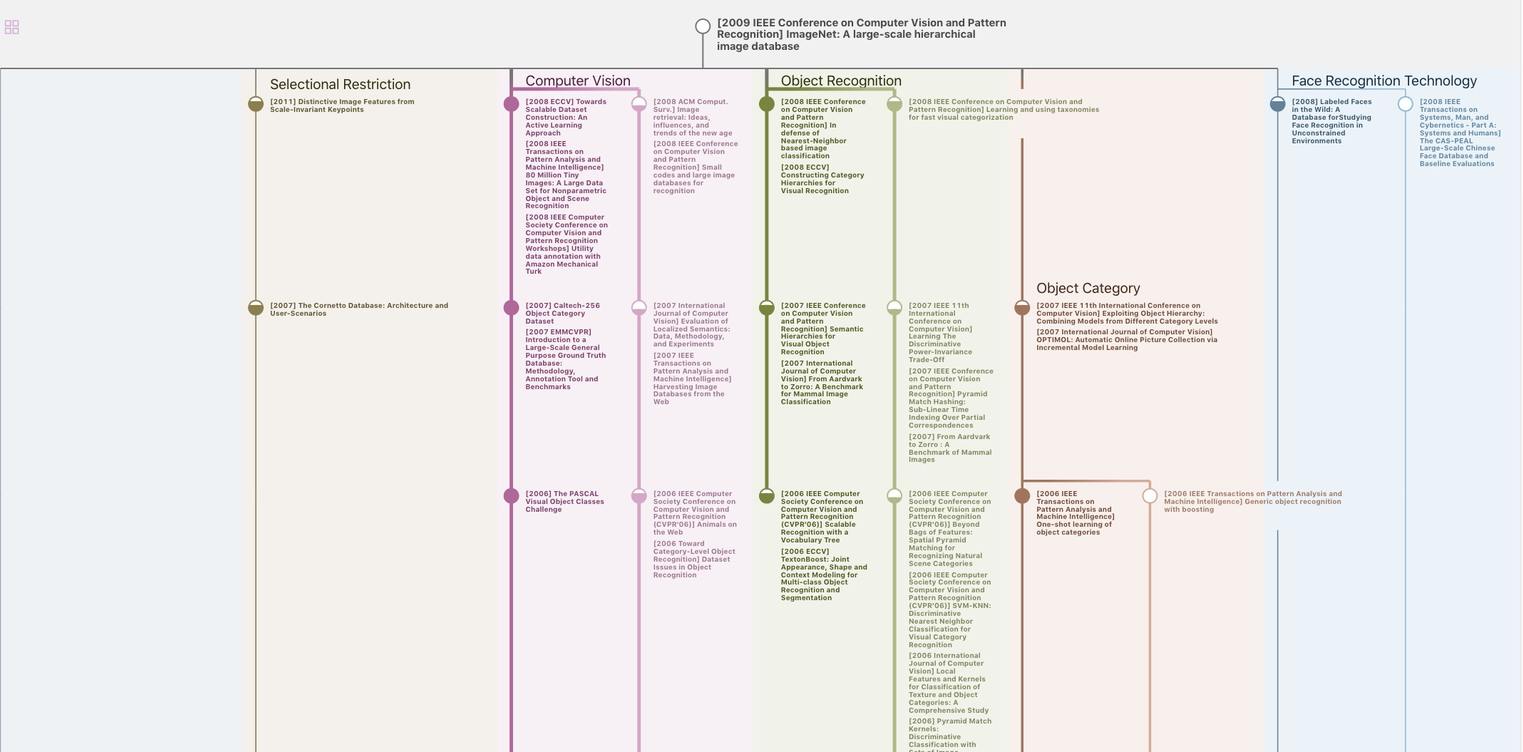
生成溯源树,研究论文发展脉络
Chat Paper
正在生成论文摘要