Learning to Simulate Daily Activities via Modeling Dynamic Human Needs
WWW 2023(2023)
摘要
Daily activity data that records individuals' various types of activities in daily life are widely used in many applications such as activity scheduling, activity recommendation, and policymaking. Though with high value, its accessibility is limited due to high collection costs and potential privacy issues. Therefore, simulating human activities to produce massive high-quality data is of great importance to benefit practical applications. However, existing solutions, including rule-based methods with simplified assumptions of human behavior and data-driven methods directly fitting real-world data, both cannot fully qualify for matching reality. In this paper, motivated by the classic psychological theory, Maslow's need theory describing human motivation, we propose a knowledge-driven simulation framework based on generative adversarial imitation learning. To enhance the fidelity and utility of the generated activity data, our core idea is to model the evolution of human needs as the underlying mechanism that drives activity generation in the simulation model. Specifically, this is achieved by a hierarchical model structure that disentangles different need levels, and the use of neural stochastic differential equations that successfully captures piecewise-continuous characteristics of need dynamics. Extensive experiments demonstrate that our framework outperforms the state-of-the-art baselines in terms of data fidelity and utility. Besides, we present the insightful interpretability of the need modeling. The code is available at https://github.com/tsinghua-fib-lab/SAND.
更多查看译文
AI 理解论文
溯源树
样例
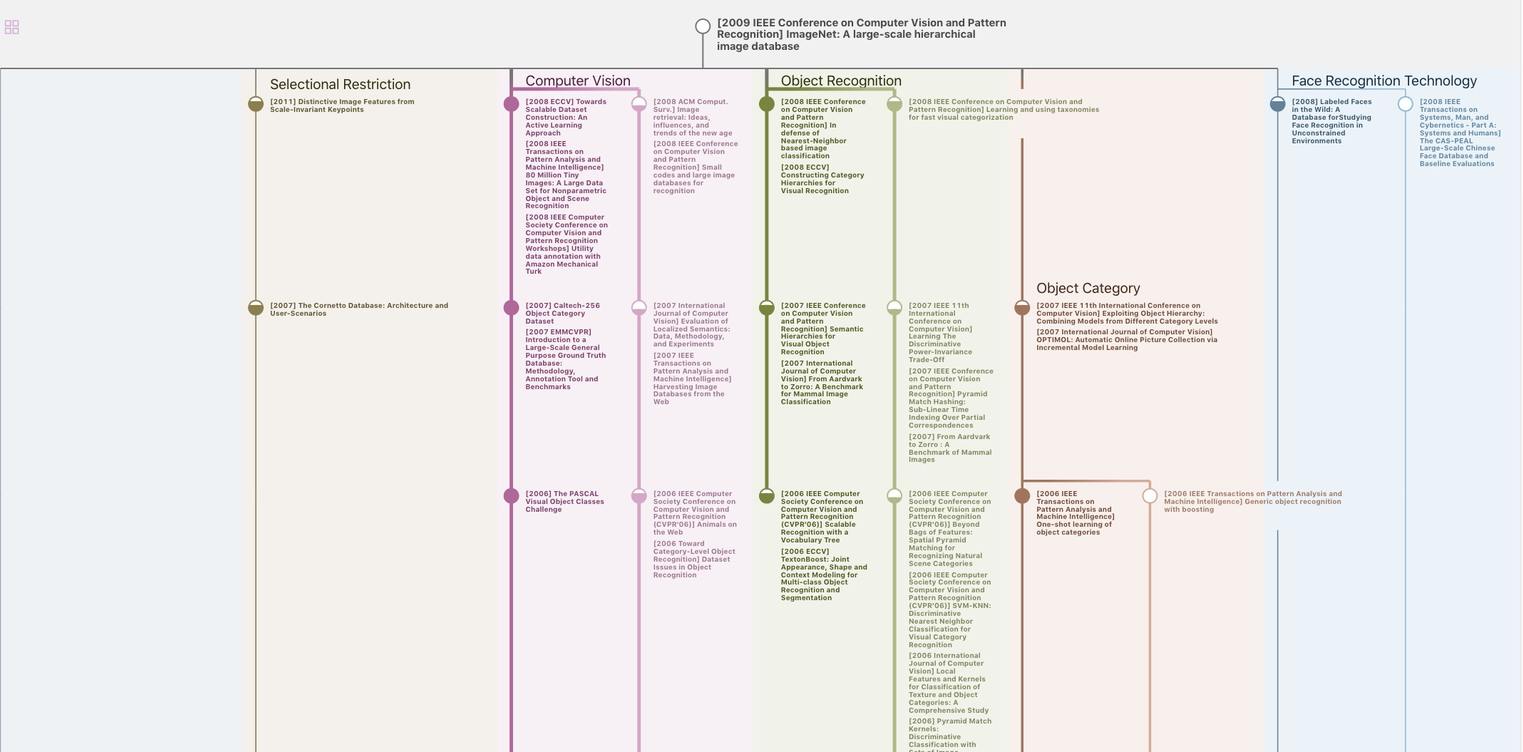
生成溯源树,研究论文发展脉络
Chat Paper
正在生成论文摘要