Towards estimating marine wildlife abundance using aerial surveys and deep learning with hierarchical classifications subject to error
biorxiv(2023)
摘要
Aerial count surveys of wildlife populations are a prominent monitoring method for many wildlife species. Traditionally, these surveys utilize human observers to detect, count, and classify observations to species. However, given recent technological advances, many research groups are exploring the combined use of remote sensing and deep learning methods to replace human observers in order to improve data quality and reproducibility, reduce disturbance to wildlife, and increase aircrew safety. Given that deep learning detection and classification are not perfect and that statistical inference from ecological models is generally very sensitive to misclassification, we require study designs and statistical models to accommodate these observation errors.
As part of an ongoing effort by the U.S. Fish and Wildlife Service, Bureau of Ocean Energy Management, and U.S. Geological Survey to survey marine birds and other marine wildlife using digital aerial imagery and deep learning object detection and classification, we developed a general hierarchical model for estimating species-specific abundance that accommodates object-level errors in classification. We consider hierarchical deep learning classification at multiple taxonomic levels subject to misclassification, hierarchically-structured human validation data subject to partial and erroneous misclassification, and an image censoring process leading to preferential sampling. We demonstrate that this model can estimate species-specific abundance and habitat relationships without bias when the assumptions are met, and we discuss the plausibility of these assumptions in practice for this study and others like it.
Finally, we use this model to demonstrate the relevance of the features of the ecological systems under study to the classification task itself. In models that couple the ecological and classification processes into a single, hierarchical model, the true classes are treated as latent variables to be estimated and are informed by both the classification probability parameters and the ecological parameters that determine the expected frequencies of each class at the level the data are being modeled (e.g., site or site by occasion). We show that ignoring the expected frequencies of each class (when they are imbalanced) can cause correction for misclassification to produce biased parameter estimates, but coupling the ecological and classification models allows for the variability in relative class frequency across space and time due to ecological and sampling conditions to be accommodated with spatial or temporal covariates. As a result, bias is removed, classification is more accurate, and uncertainty is propagated between the ecological and classification models. We therefore argue that ability of deep learning classifiers, and classifiers more generally, to produce reliable ecological inference depends, in part, on the ecological system under study.
### Competing Interest Statement
The authors have declared no competing interest.
更多查看译文
关键词
marine wildlife abundance,aerial surveys,deep learning
AI 理解论文
溯源树
样例
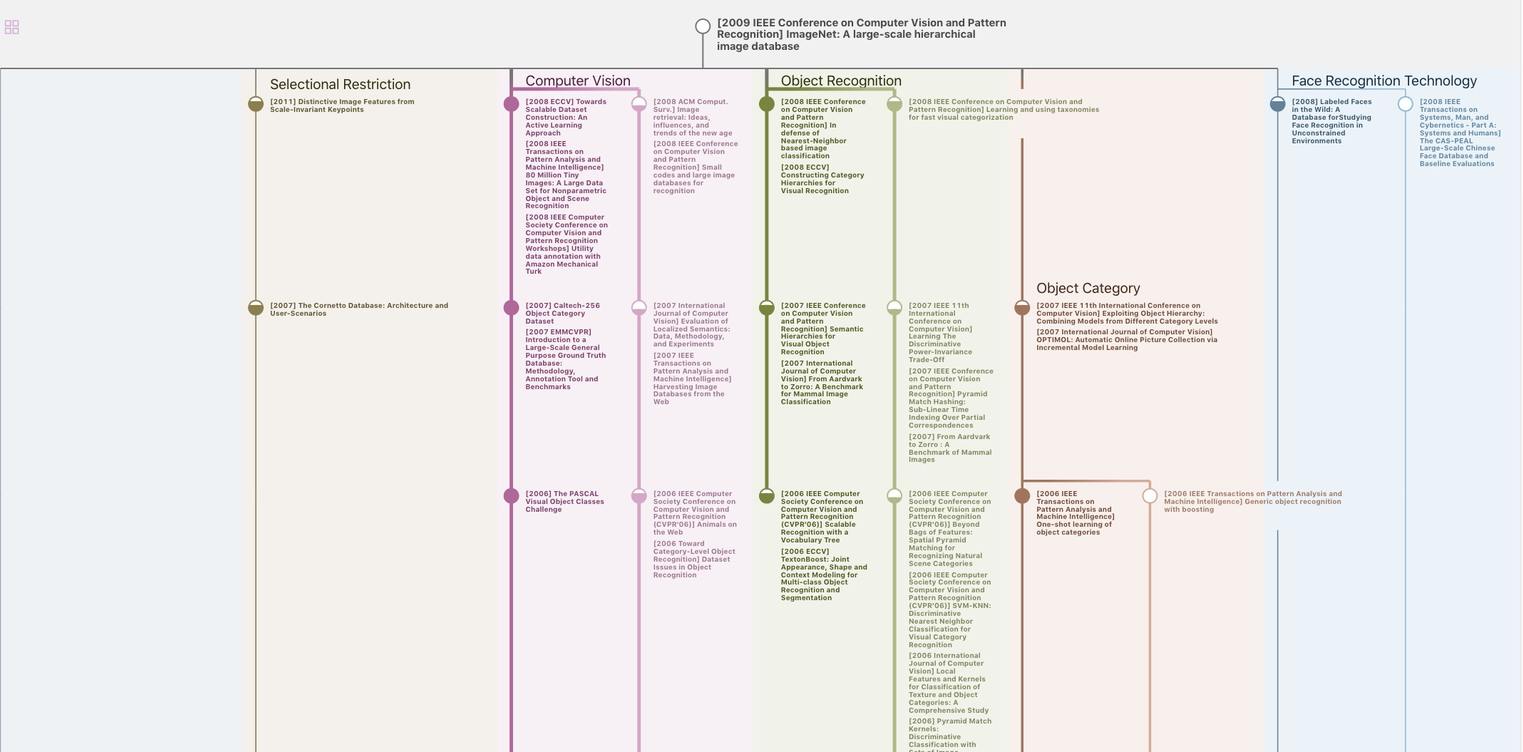
生成溯源树,研究论文发展脉络
Chat Paper
正在生成论文摘要