Output Feedback Stochastic MPC with Hard Input Constraints
2023 AMERICAN CONTROL CONFERENCE, ACC(2023)
摘要
We present an output feedback stochastic model predictive controller (SMPC) for constrained linear time-invariant systems. The system is perturbed by additive Gaussian disturbances on state and additive Gaussian measurement noise on output. A Kalman filter is used for state estimation and an SMPC is designed to satisfy chance constraints on states and hard constraints on actuator inputs. The proposed SMPC constructs bounded sets for the state evolution and uses a tube-based constraint tightening strategy where the tightened constraints are time-invariant. We prove that the proposed SMPC can guarantee an infeasibility rate below a user-specified tolerance. We numerically compare our method with a classical output feedback SMPC with simulation results which highlight the efficacy of the proposed algorithm.
更多查看译文
AI 理解论文
溯源树
样例
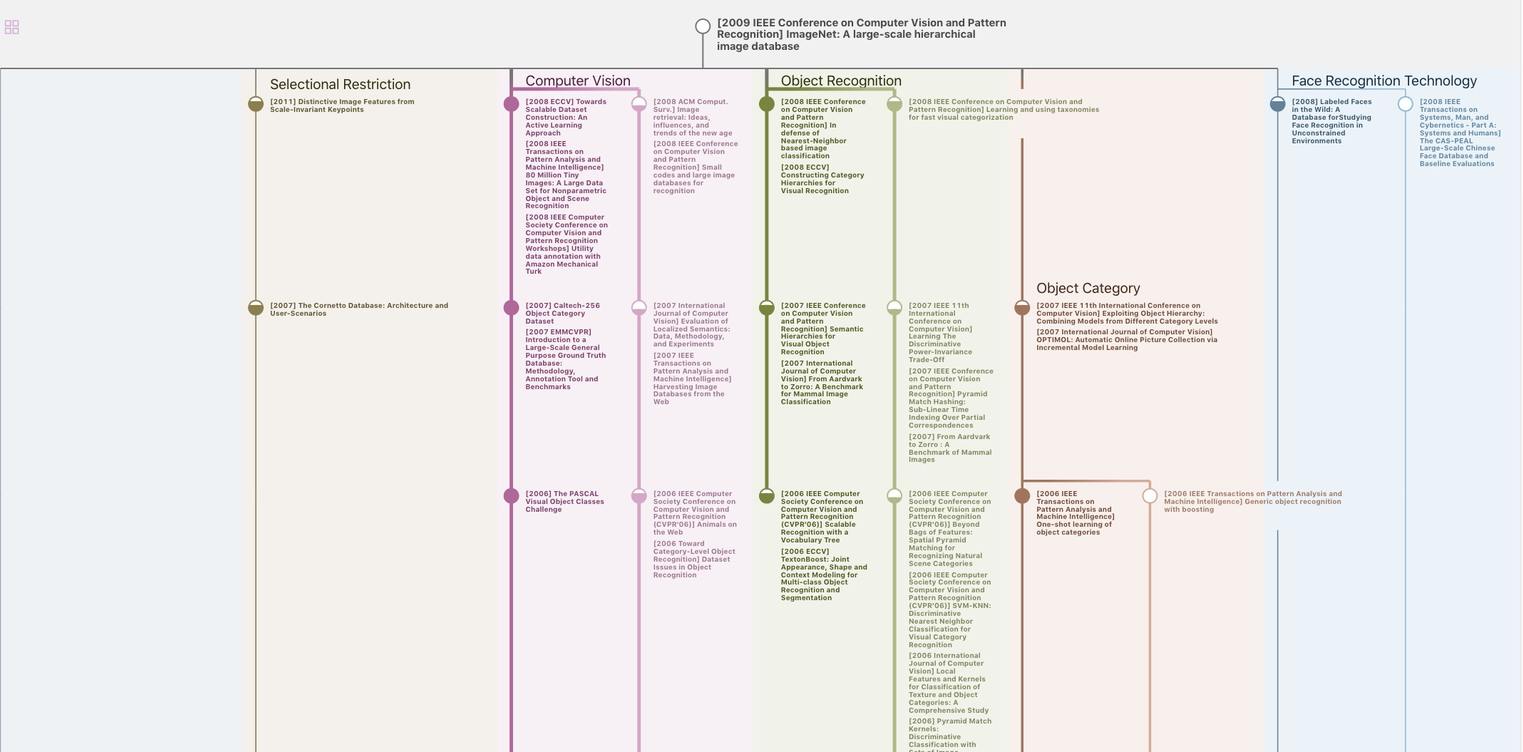
生成溯源树,研究论文发展脉络
Chat Paper
正在生成论文摘要