Tell Model Where to Attend: Improving Interpretability of Aspect-Based Sentiment Classification via Small Explanation Annotations
ICASSP(2023)
Abstract
Gradient-based explanation methods play an important role in the field of interpreting complex deep neural networks for NLP models. However, the existing work has shown that the gradients of a model are unstable and easily manipulable, which impacts the model's reliability largely. According to our preliminary analyses, we also find the interpretability of gradient-based methods is limited for complex tasks, such as aspect-based sentiment classification (ABSC). In this paper, we propose an \textbf{I}nterpretation-\textbf{E}nhanced \textbf{G}radient-based framework for \textbf{A}BSC via a small number of explanation annotations, namely \texttt{{IEGA}}. Particularly, we first calculate the word-level saliency map based on gradients to measure the importance of the words in the sentence towards the given aspect. Then, we design a gradient correction module to enhance the model's attention on the correct parts (e.g., opinion words). Our model is model agnostic and task agnostic so that it can be integrated into the existing ABSC methods or other tasks. Comprehensive experimental results on four benchmark datasets show that our \texttt{IEGA} can improve not only the interpretability of the model but also the performance and robustness.
MoreTranslated text
Key words
Interpretability,aspect-based sentiment classification,gradient-based
AI Read Science
Must-Reading Tree
Example
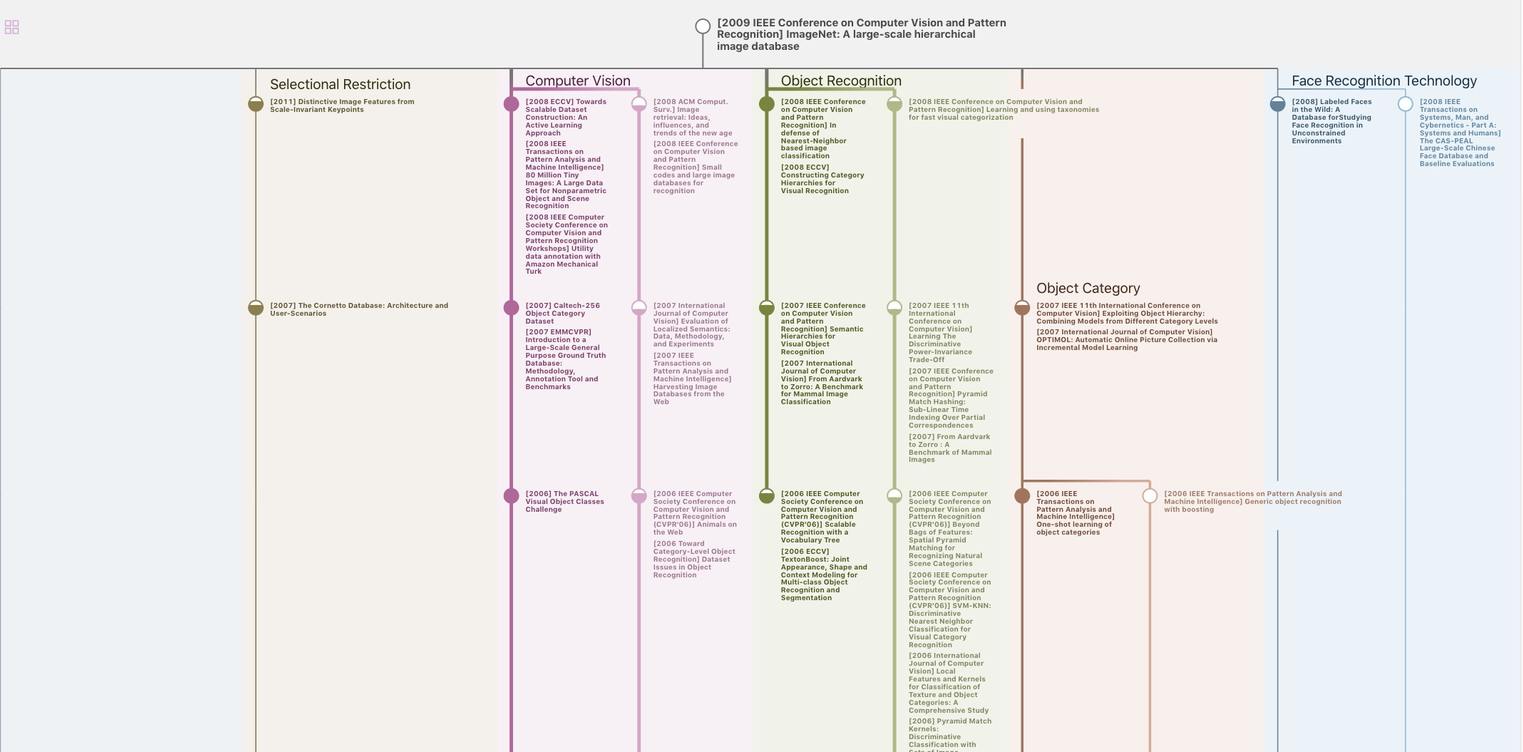
Generate MRT to find the research sequence of this paper
Chat Paper
Summary is being generated by the instructions you defined