Speech Privacy Leakage from Shared Gradients in Distributed Learning
arxiv(2023)
摘要
Distributed machine learning paradigms, such as federated learning, have been recently adopted in many privacy-critical applications for speech analysis. However, such frameworks are vulnerable to privacy leakage attacks from shared gradients. Despite extensive efforts in the image domain, the exploration of speech privacy leakage from gradients is quite limited. In this paper, we explore methods for recovering private speech/speaker information from the shared gradients in distributed learning settings. We conduct experiments on a keyword spotting model with two different types of speech features to quantify the amount of leaked information by measuring the similarity between the original and recovered speech signals. We further demonstrate the feasibility of inferring various levels of side-channel information, including speech content and speaker identity, under the distributed learning framework without accessing the user's data.
更多查看译文
关键词
shared gradients,privacy,speech,learning
AI 理解论文
溯源树
样例
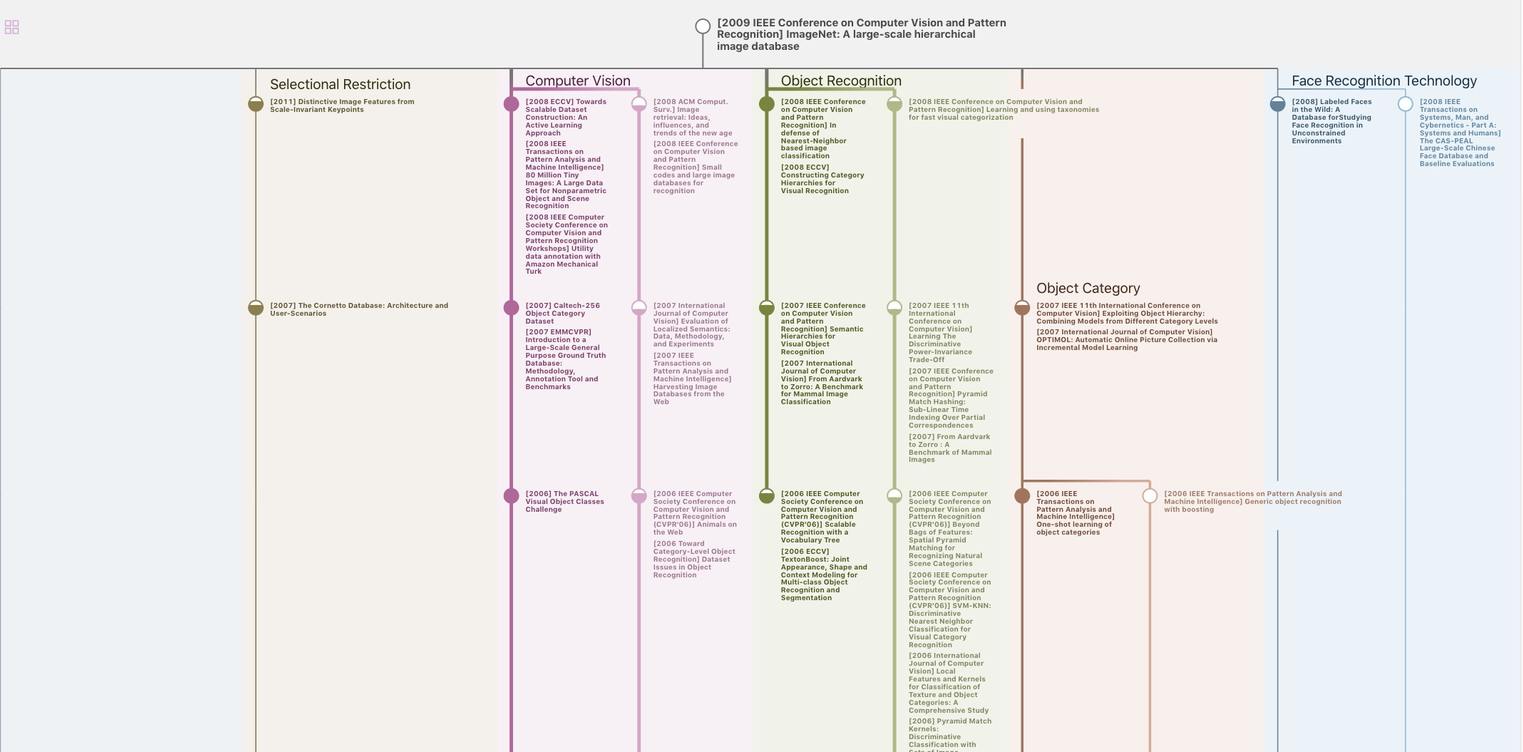
生成溯源树,研究论文发展脉络
Chat Paper
正在生成论文摘要