Adaptive Sparse Gaussian Process.
IEEE Transactions on Neural Networks and Learning Systems(2023)
Abstract
Adaptive learning is necessary for nonstationary environments where the learning machine needs to forget past data distribution. Efficient algorithms require a compact model update to not grow in computational burden with the incoming data and with the lowest possible computational cost for online parameter updating. Existing solutions only partially cover these needs. Here, we propose the first adaptive sparse Gaussian process (GP) able to address all these issues. We first reformulate a variational sparse GP (VSGP) algorithm to make it adaptive through a forgetting factor. Next, to make the model inference as simple as possible, we propose updating a single inducing point of the SGP model together with the remaining model parameters every time a new sample arrives. As a result, the algorithm presents a fast convergence of the inference process, which allows an efficient model update (with a single inference iteration) even in highly nonstationary environments. Experimental results demonstrate the capabilities of the proposed algorithm and its good performance in modeling the predictive posterior in mean and confidence interval estimation compared to state-of-the-art approaches.
MoreTranslated text
Key words
Adaptive learning,online learning,sparse Gaussian process (GP),variational learning
AI Read Science
Must-Reading Tree
Example
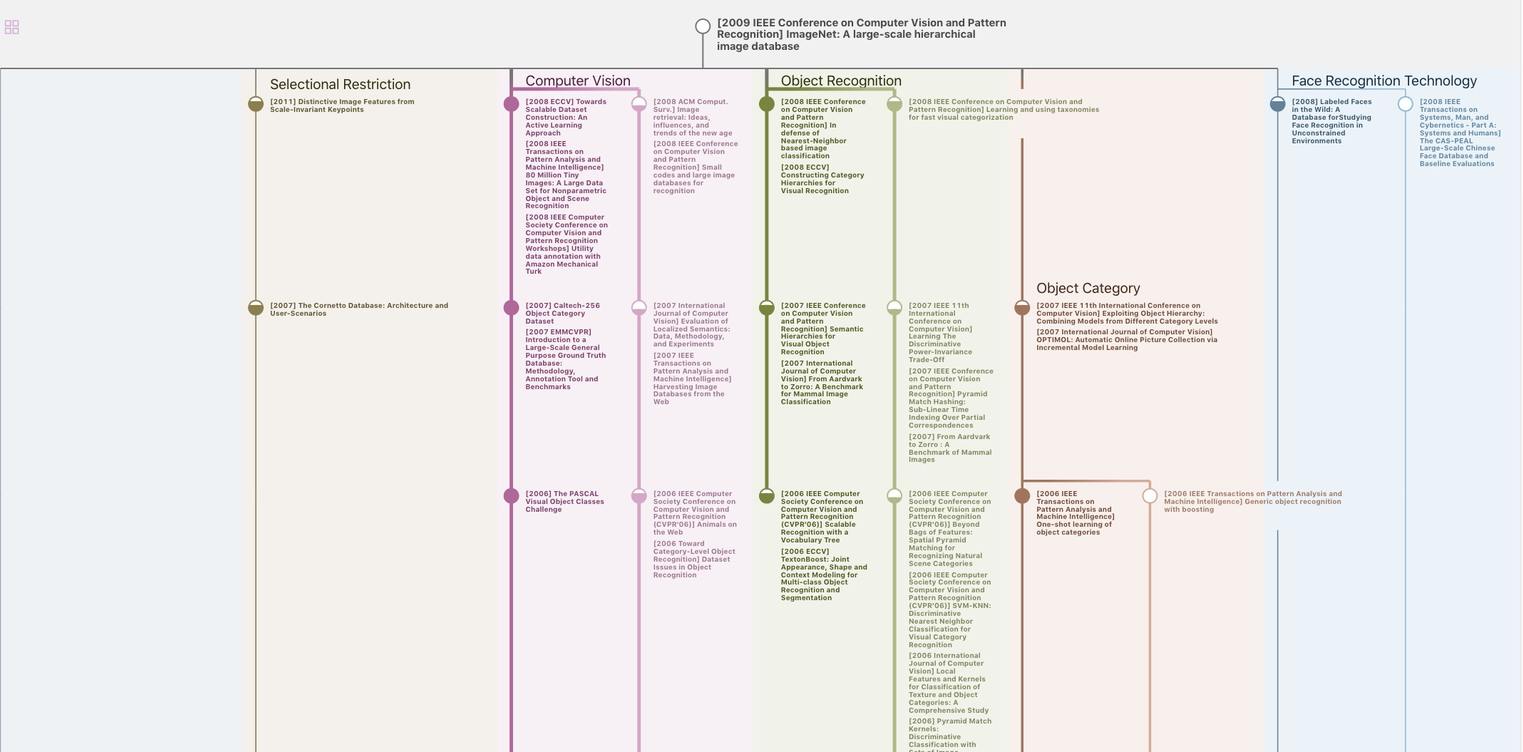
Generate MRT to find the research sequence of this paper
Chat Paper
Summary is being generated by the instructions you defined