InOR-Net: Incremental 3-D Object Recognition Network for Point Cloud Representation
arxiv(2023)
摘要
3-D object recognition has successfully become an appealing research topic in the real world. However, most existing recognition models unreasonably assume that the categories of 3-D objects cannot change over time in the real world. This unrealistic assumption may result in significant performance degradation for them to learn new classes of 3-D objects consecutively due to the catastrophic forgetting on old learned classes. Moreover, they cannot explore which 3-D geometric characteristics are essential to alleviate the catastrophic forgetting on old classes of 3-D objects. To tackle the above challenges, we develop a novel Incremental 3-D Object Recognition Network (i.e., InOR-Net), which could recognize new classes of 3-D objects continuously by overcoming the catastrophic forgetting on old classes. Specifically, category-guided geometric reasoning is proposed to reason local geometric structures with distinctive 3-D characteristics of each class by leveraging intrinsic category information. We then propose a novel critic-induced geometric attention mechanism to distinguish which 3-D geometric characteristics within each class are beneficial to overcome the catastrophic forgetting on old classes of 3-D objects while preventing the negative influence of useless 3-D characteristics. In addition, a dual adaptive fairness compensations’ strategy is designed to overcome the forgetting brought by class imbalance by compensating biased weights and predictions of the classifier. Comparison experiments verify the state-of-the-art performance of the proposed InOR-Net model on several public point cloud datasets.
更多查看译文
关键词
Point cloud compression,Object recognition,Solid modeling,Feature extraction,Task analysis,Adaptation models,Training,3-D object recognition,catastrophic forgetting,class-incremental learning,point cloud representation
AI 理解论文
溯源树
样例
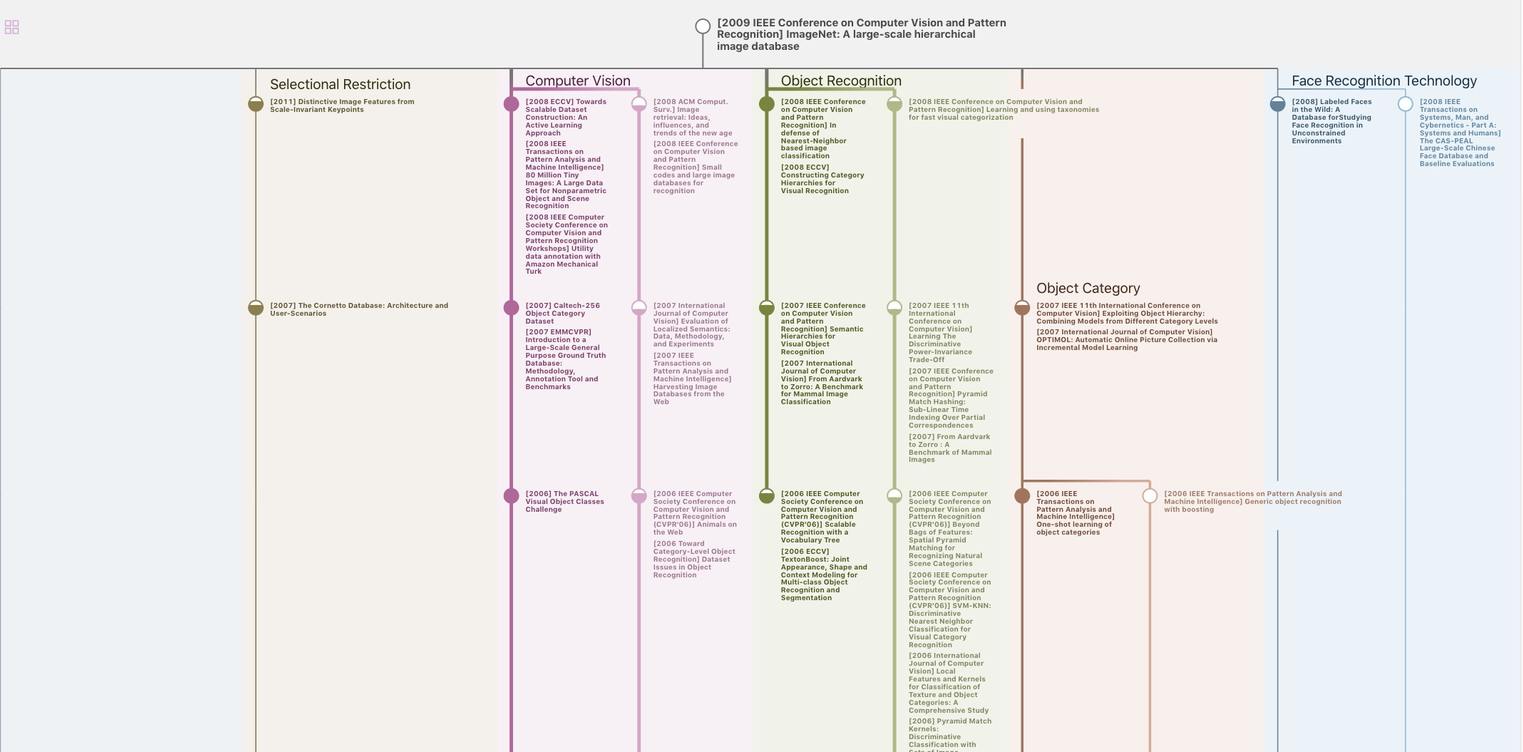
生成溯源树,研究论文发展脉络
Chat Paper
正在生成论文摘要