Ergodic characterization of non-ergodic anomalous diffusion processes
arXiv (Cornell University)(2023)
摘要
Canonical characterization techniques that rely upon mean squared displacement ($\mathrm{MSD}$) break down for non-ergodic processes, making it challenging to characterize anomalous diffusion from an individual time-series measurement. Non-ergodicity reigns when the time-averaged mean square displacement $\mathrm{TA}$-$\mathrm{MSD}$ differs from the ensemble-averaged mean squared displacement $\mathrm{EA}$-$\mathrm{MSD}$ even in the limit of long measurement series. In these cases, the typical theoretical results for ensemble averages cannot be used to understand and interpret data acquired from time averages. The difficulty then lies in obtaining statistical descriptors of the measured diffusion process that are not non-ergodic. We show that linear descriptors such as the standard deviation ($SD$), coefficient of variation ($CV$), and root mean square ($RMS$) break ergodicity in proportion to non-ergodicity in the diffusion process. In contrast, time series of descriptors addressing sequential structure and its potential nonlinearity: multifractality change in a time-independent way and fulfill the ergodic assumption, largely independent of the time series' non-ergodicity. We show that these findings follow the multiplicative cascades underlying these diffusion processes. Adding fractal and multifractal descriptors to typical linear descriptors would improve the characterization of anomalous diffusion processes. Two particular points bear emphasis here. First, as an appropriate formalism for encoding the nonlinearity that might generate non-ergodicity, multifractal modeling offers descriptors that can behave ergodically enough to meet the needs of linear modeling. Second, this capacity to describe non-ergodic processes in ergodic terms offers the possibility that multifractal modeling could unify several disparate non-ergodic diffusion processes into a common framework.
更多查看译文
关键词
ergodic characterization,diffusion,processes,non-ergodic
AI 理解论文
溯源树
样例
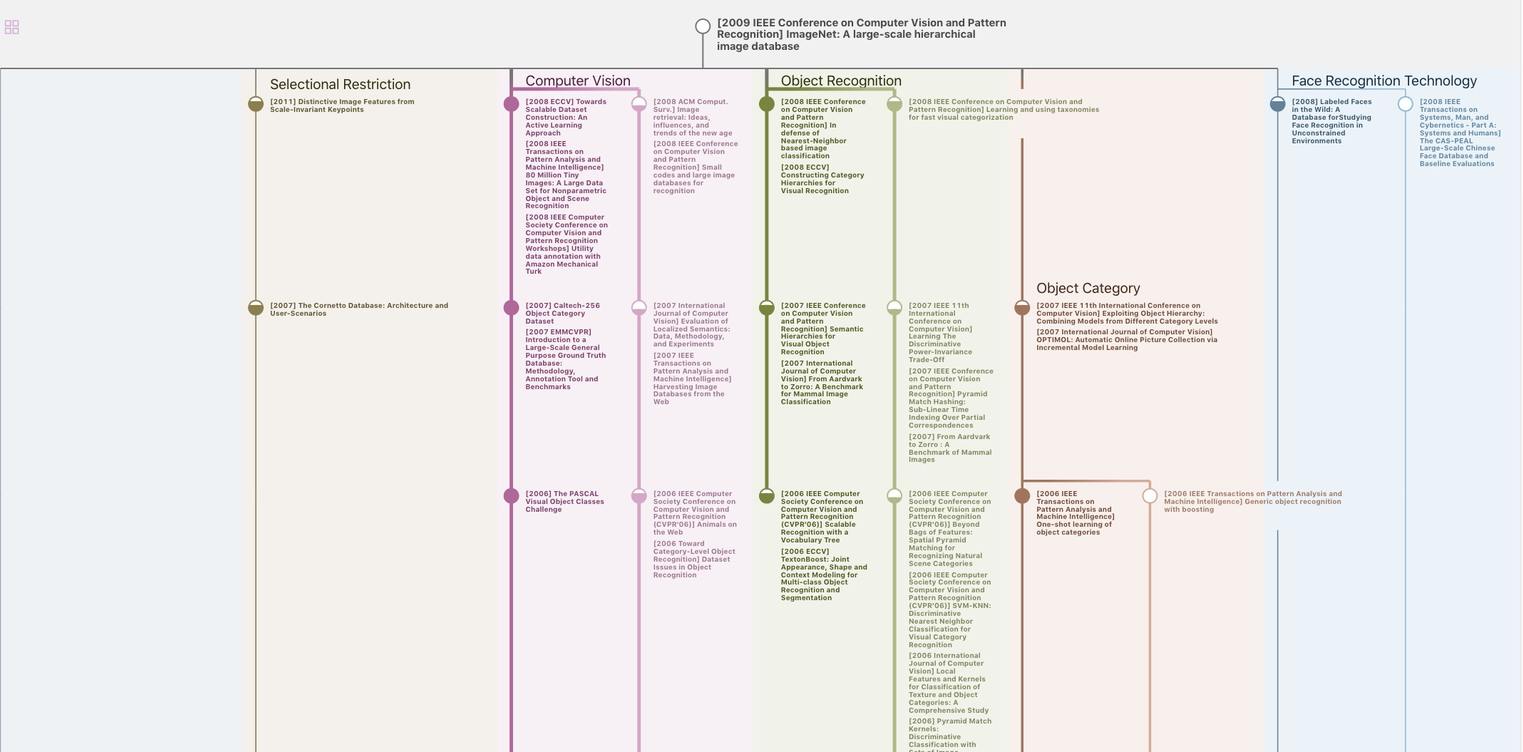
生成溯源树,研究论文发展脉络
Chat Paper
正在生成论文摘要