Incipient Fault Detection in Power Distribution System: A Time-Frequency Embedded Deep Learning Based Approach
arxiv(2023)
摘要
Incipient fault detection in power distribution systems is crucial to improve the reliability of the grid. However, the non-stationary nature and the inadequacy of the training dataset due to the self-recovery of the incipient fault signal, make the incipient fault detection in power distribution systems a great challenge. In this paper, we focus on incipient fault detection in power distribution systems and address the above challenges. In particular, we propose an ADaptive Time-Frequency Memory(AD-TFM) cell by embedding wavelet transform into the Long Short-Term Memory (LSTM), to extract features in time and frequency domain from the non-stationary incipient fault signals.We make scale parameters and translation parameters of wavelet transform learnable to adapt to the dynamic input signals. Based on the stacked AD-TFM cells, we design a recurrent neural network with ATtention mechanism, named AD-TFM-AT model, to detect incipient fault with multi-resolution and multi-dimension analysis. In addition, we propose two data augmentation methods, namely phase switching and temporal sliding, to effectively enlarge the training datasets. Experimental results on two open datasets show that our proposed AD-TFM-AT model and data augmentation methods achieve state-of-the-art (SOTA) performance of incipient fault detection in power distribution system. We also disclose one used dataset logged at State Grid Corporation of China to facilitate future research.
更多查看译文
关键词
Attention mechanism,data augmentation,incipient fault detection,long short-term memory (LSTM),power distribution system,recurrent neural network (RNN),wavelet transform
AI 理解论文
溯源树
样例
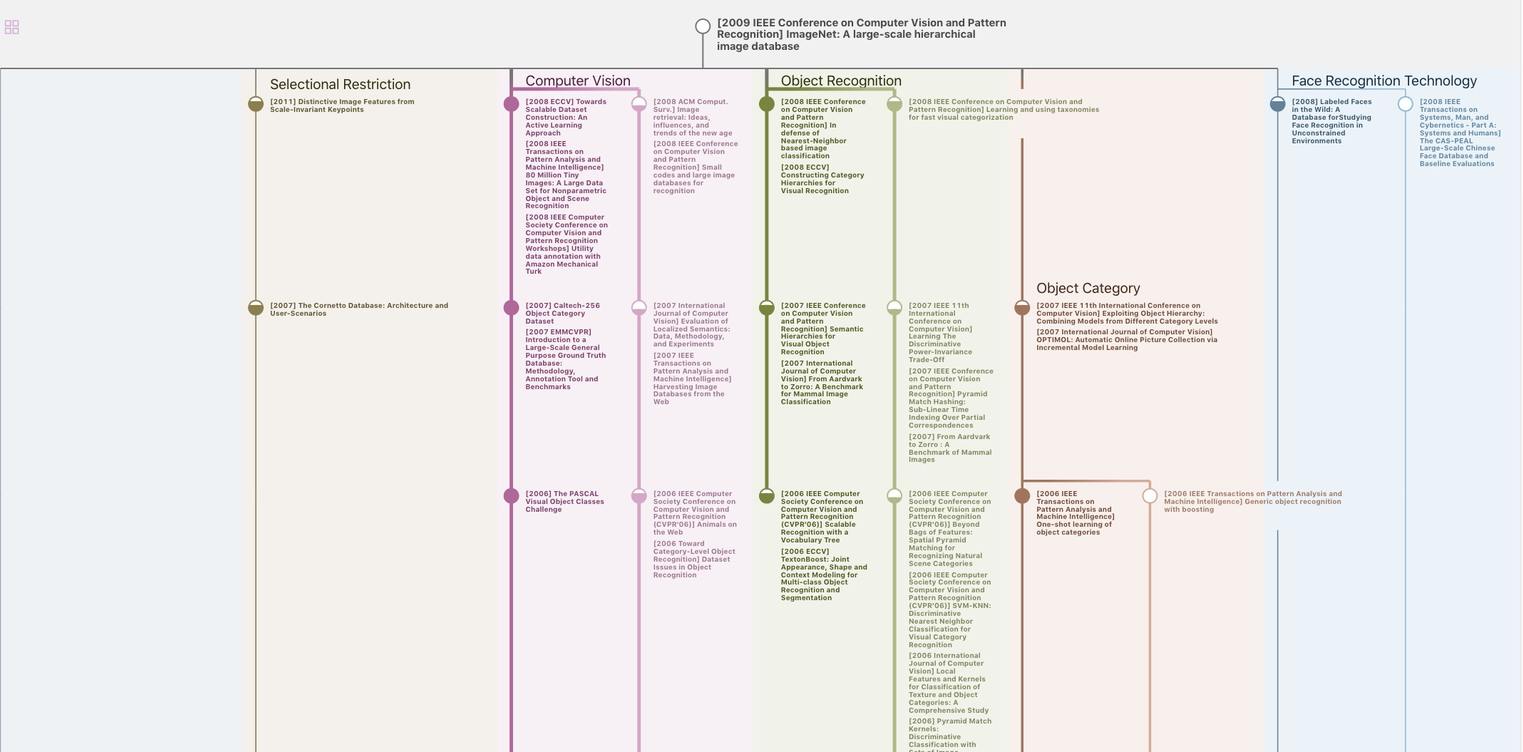
生成溯源树,研究论文发展脉络
Chat Paper
正在生成论文摘要