Deciphering Alloy Composition in Superconducting Single-Layer FeSe1-xSx on SrTiO3(001) Substrates by Machine Learning of STM/S Data.
ACS applied materials & interfaces(2023)
Abstract
Scanning tunneling microscopy (STM) is a powerful technique for imaging atomic structure and inferring information on local elemental composition, chemical bonding, and electronic excitations. However, a plain visual analysis of STM images can be challenging for such determination in multicomponent alloys, particularly beyond the diluted limit due to chemical disorder and electronic inhomogeneity. One viable solution is to use machine learning to analyze STM data and identify hidden patterns and correlations. Here, we apply this approach to determine the Se/S concentration in superconducting single-layer FeSe1-xSx alloys epitaxially grown on SrTiO3(001) substrates via molecular beam epitaxy. First, the K-means clustering method is applied to identify defect-related dI/dV tunneling spectra taken by current imaging tunneling spectroscopy. Then, the Se/S ratio is calculated by analyzing the remaining spectra based on the singular value decomposition method. Such analysis provides an efficient and reliable determination of alloy composition and further reveals the correlations of nanoscale chemical inhomogeneity to superconductivity in single-layer iron chalcogenide films.
MoreTranslated text
Key words
alloy composition, single -layer iron chalcogenides, MBE, STM, S, machine learning
AI Read Science
Must-Reading Tree
Example
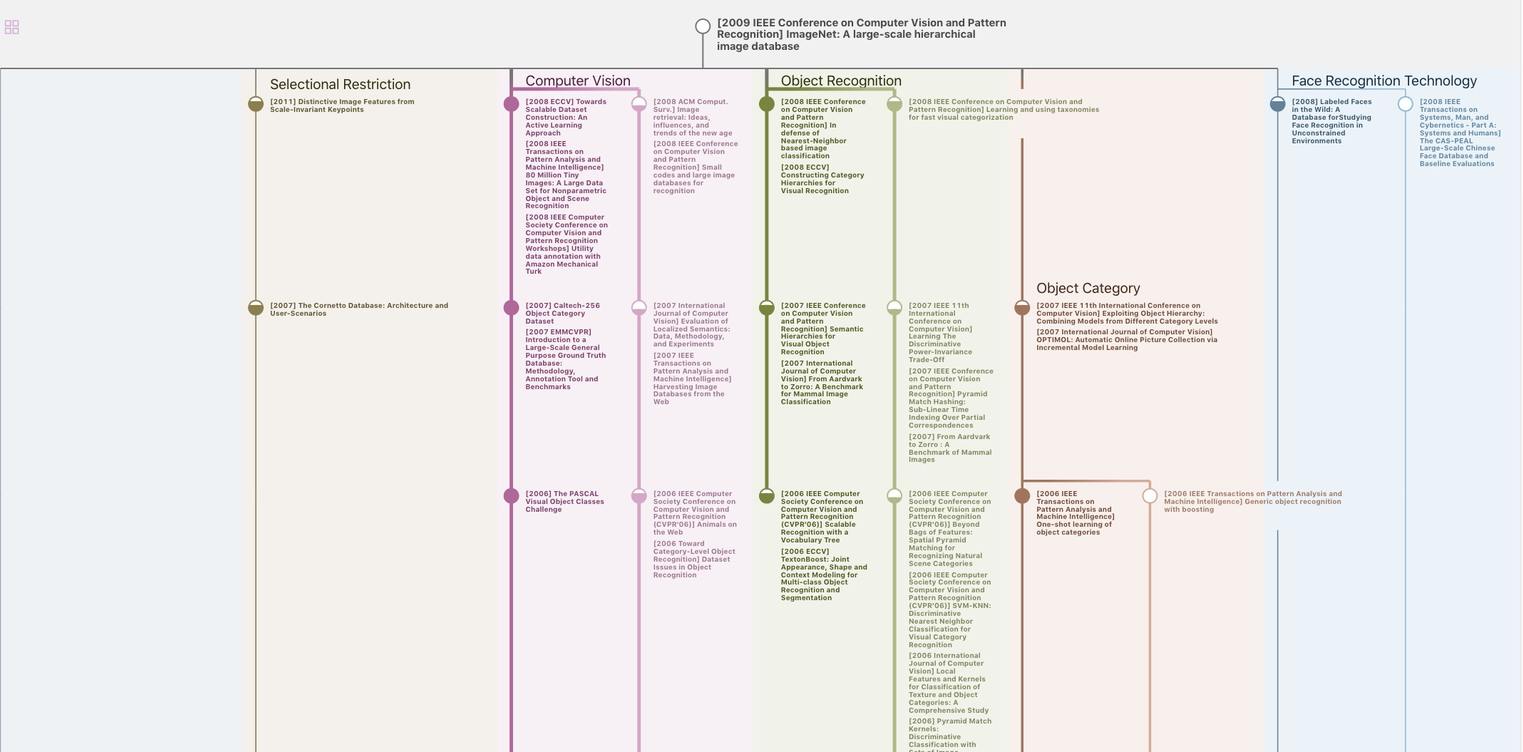
Generate MRT to find the research sequence of this paper
Chat Paper
Summary is being generated by the instructions you defined