An anatomy-based V1 model: Extraction of Low-level Features, Reduction of distortion and a V1-inspired SOM
arxiv(2023)
摘要
We present a model of the primary visual cortex V1, guided by anatomical experiments. Unlike most machine learning systems our goal is not to maximize accuracy but to realize a system more aligned to biological systems. Our model consists of the V1 layers 4, 2/3, and 5, with inter-layer connections between them in accordance with the anatomy. We further include the orientation selectivity of the V1 neurons and lateral influences in each layer. Our V1 model, when applied to the BSDS500 ground truth images (indicating LGN contour detection before V1), can extract low-level features from the images and perform a significant amount of distortion reduction. As a follow-up to our V1 model, we propose a V1-inspired self-organizing map algorithm (V1-SOM), where the weight update of each neuron gets influenced by its neighbors. V1-SOM can tolerate noisy inputs as well as noise in the weight updates better than SOM and shows a similar level of performance when trained with high dimensional data such as the MNIST dataset. Finally, when we applied V1 processing to the MNIST dataset to extract low-level features and trained V1-SOM with the modified MNIST dataset, the quantization error was significantly reduced. Our results support the hypothesis that the ventral stream performs gradual untangling of input spaces.
更多查看译文
关键词
v1 model,features,anatomy-based,low-level
AI 理解论文
溯源树
样例
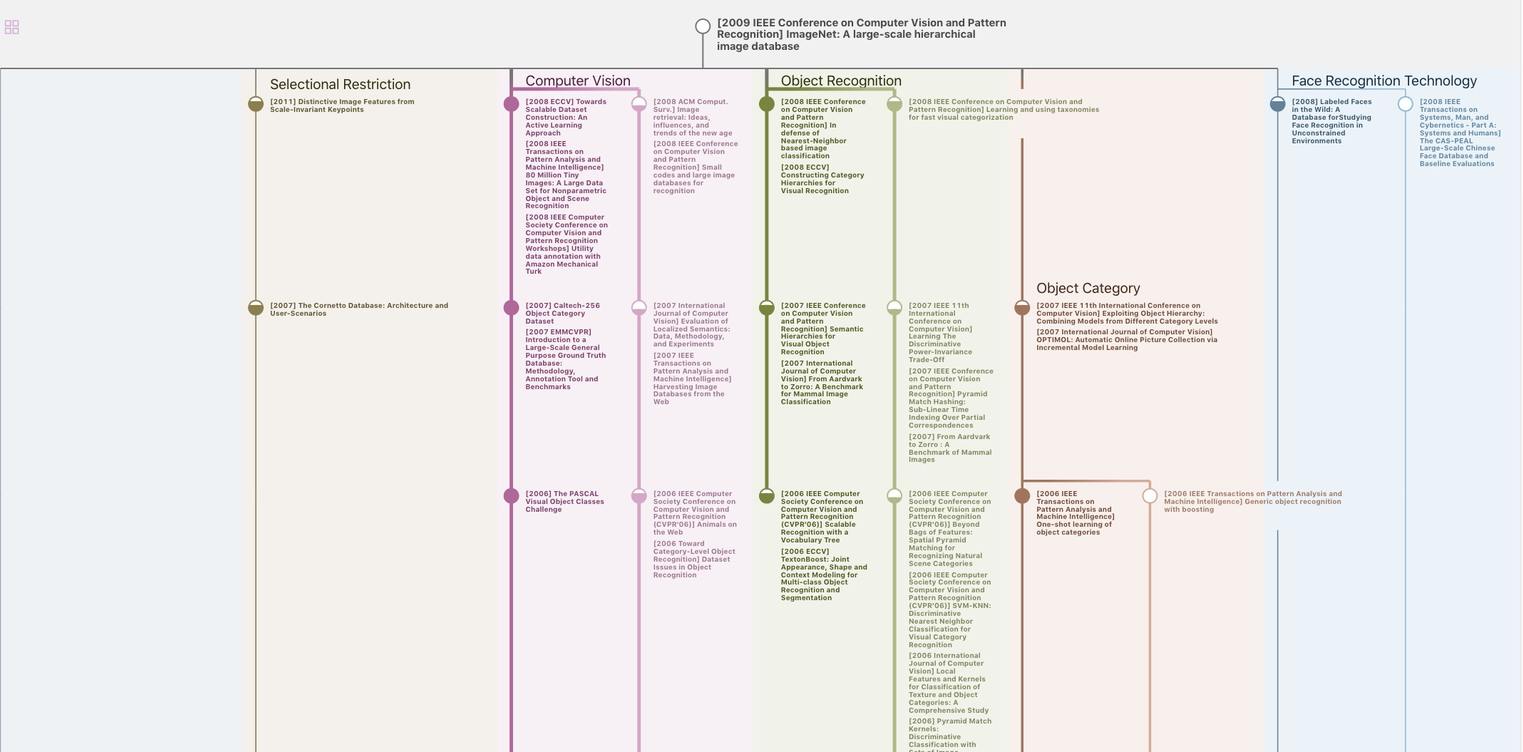
生成溯源树,研究论文发展脉络
Chat Paper
正在生成论文摘要