RECLAIM: Renewable Energy Based Demand-Side Management Using Machine Learning Models.
IEEE Access(2023)
摘要
The diesel generators sets (DGs) and battery storage systems (BSS) are the essential energy sources in a modern high-rise buildings. In this paper DG, BSS and Photovoltaic system (PV) has been considered to minimize the grid power injection using a centralized Energy Management System (EMS). Machine Learning (ML) techniques are used to predict the performance of various regression models by comparing grid power and load curves. It includes Artificial Neural Network (ANN), Wide Neural Network (WNN), Linear Regression (LR), Linear Regression Interaction (LR-I), Linear Regression Stepwise (LR-S), Regression Fine Tree (RF-T), Regression Coarse Tree (RC-T) and Gaussian Process Regression (GPR) based techniques. The Demand Side Management (DSM) techniques such as peak shaving and valley filling is integrated with ML technique in a Hybrid energy source (HS) system.The comparative analysis of results depicts the effective reshaping of the grid profile without scheduling or disconnecting the loads. Matlab simulation software is used to validate the results.
更多查看译文
关键词
Artificial neural network,regression tree,linear regression,demand side management,machine learning
AI 理解论文
溯源树
样例
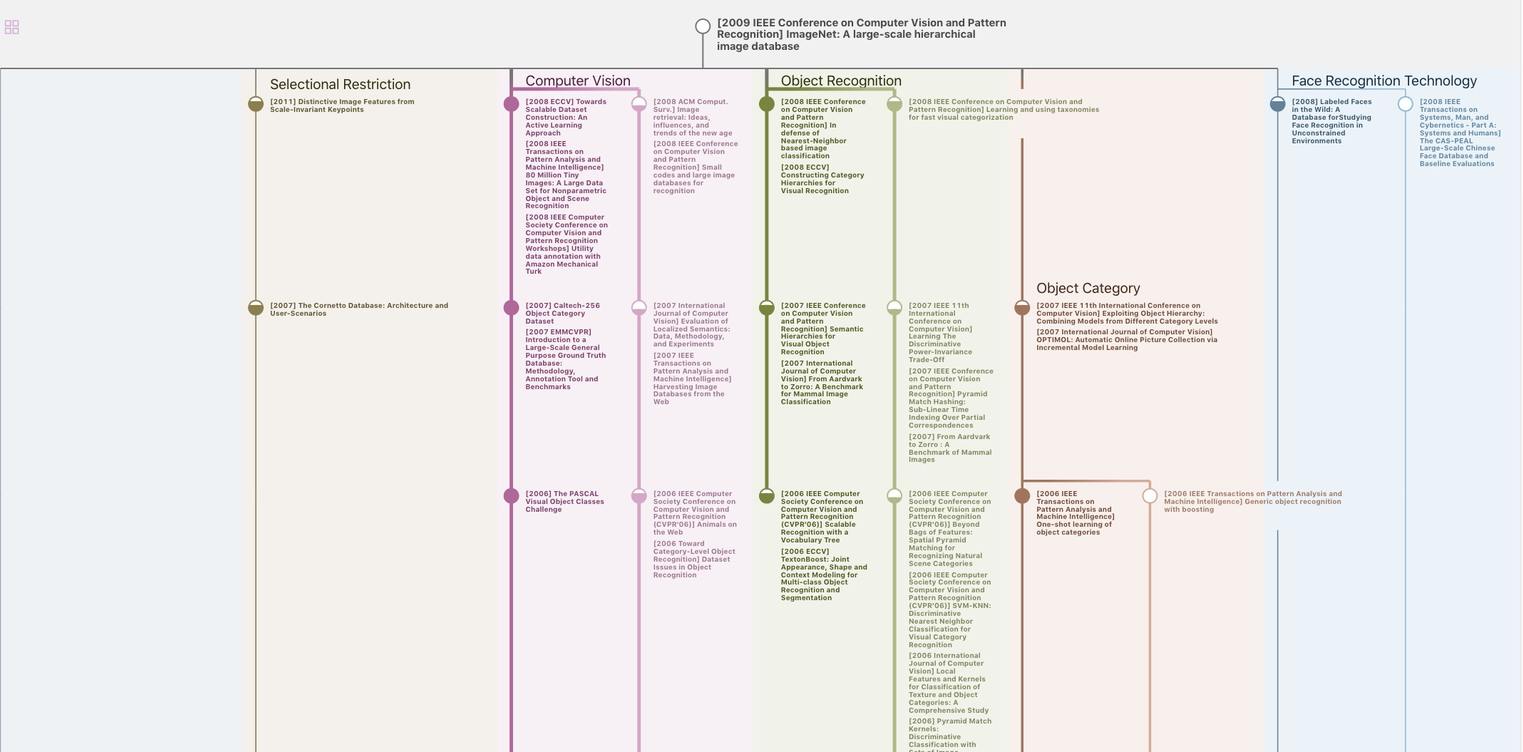
生成溯源树,研究论文发展脉络
Chat Paper
正在生成论文摘要