A Two-Stage Feature Selection Approach for Fruit Recognition Using Camera Images With Various Machine Learning Classifiers.
IEEE Access(2022)
摘要
Fruit and vegetable identification and classification system is always necessary and advantageous for the agriculture business, the food processing sector, as well as the convenience shops and hypermarkets where these products are sold. Therefore, it is necessary to build an effective automated tool to meet the needs of the market by boosting the outcome, in order to improve economic efficiency. In this paper, a two-stage model is proposed to recognize fruits using camera images. We employed a Densnet121 to get the features from the fruits dataset in the first module. In the second stage, we utilize a feature subset selection method to choose the most significant features for recognizing fruits from the images of the fruits. In this study, Adaptive particle - Grey Wolf Optimization (APGWO) has been applied for choosing the most pertinent features. The final subset feature has been used for recognizing fruits using several machine learning classifiers, namely K-Nearest Neighbor (KNN), Support Vector Machine (SVM), Decision Tree (DT), Random Forest (RF), and Multilayer Perceptron (MLP). The proposed research's experimental results are highly effective; the training time of proposed models is reduced to over 50%, and the classification accuracy reaches 99%.
更多查看译文
关键词
Wrapper,feature selection,adaptive particle grey wolf optimization,feature extraction,fruits recognition
AI 理解论文
溯源树
样例
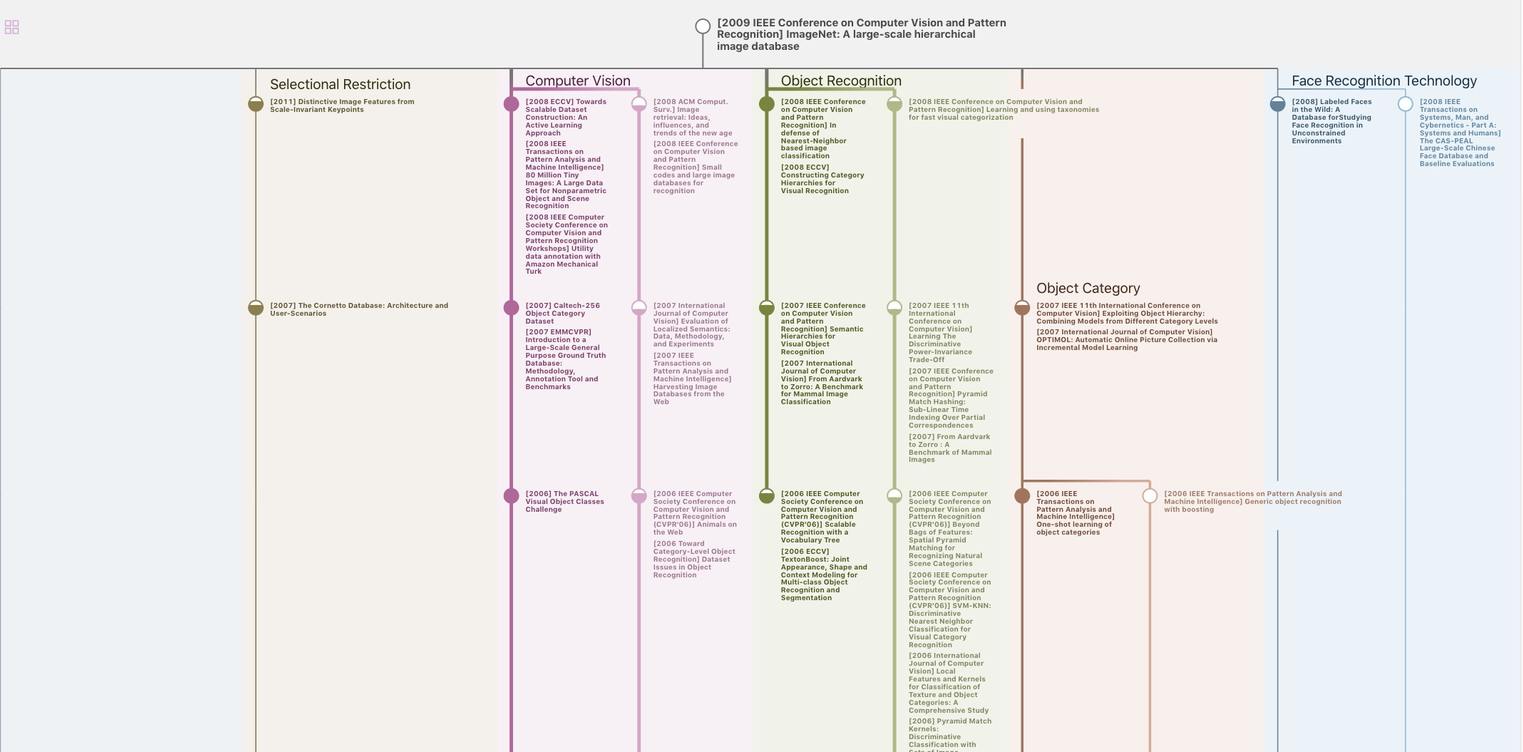
生成溯源树,研究论文发展脉络
Chat Paper
正在生成论文摘要