Partially Collaborative Edge Caching Based on Federated Deep Reinforcement Learning.
IEEE Trans. Veh. Technol.(2023)
摘要
In this paper, edge caching is investigated in fog radio access networks subject to dynamic content popularity. In order to improve the long-term cache-hit-ratio (CHR), a federated deep reinforcement learning (FDRL) framework is proposed, in which multiple fog access points (FAPs) adjust their caching strategies under the coordination of a central server (CS). Owing to the non-i.i.d data acquired over different FAPs, the traditional federated learning (FL) method may suffer from performance loss due to maintaining a global model at the CS. To address this shortcoming, a partially collaborative caching (PCC) algorithm is proposed by switching the data training between two models that are maintained at each FAP, to achieve a balance between the users' specific local characteristics and holistic global characteristics. Experiments on a real-world dataset demonstrate that significant performance gains are achieved by the proposed FDRL in terms of CHR. Furthermore, with an appropriate switching factor, the proposed PCC algorithm outperforms FL with full collaboration among FAPs.
更多查看译文
关键词
Collaboration,Data models,Training,Switches,Reinforcement learning,Computational modeling,Systematics,Cache-hit-ratio,collaborative edge caching,deep rein-forcement learning,federated learning
AI 理解论文
溯源树
样例
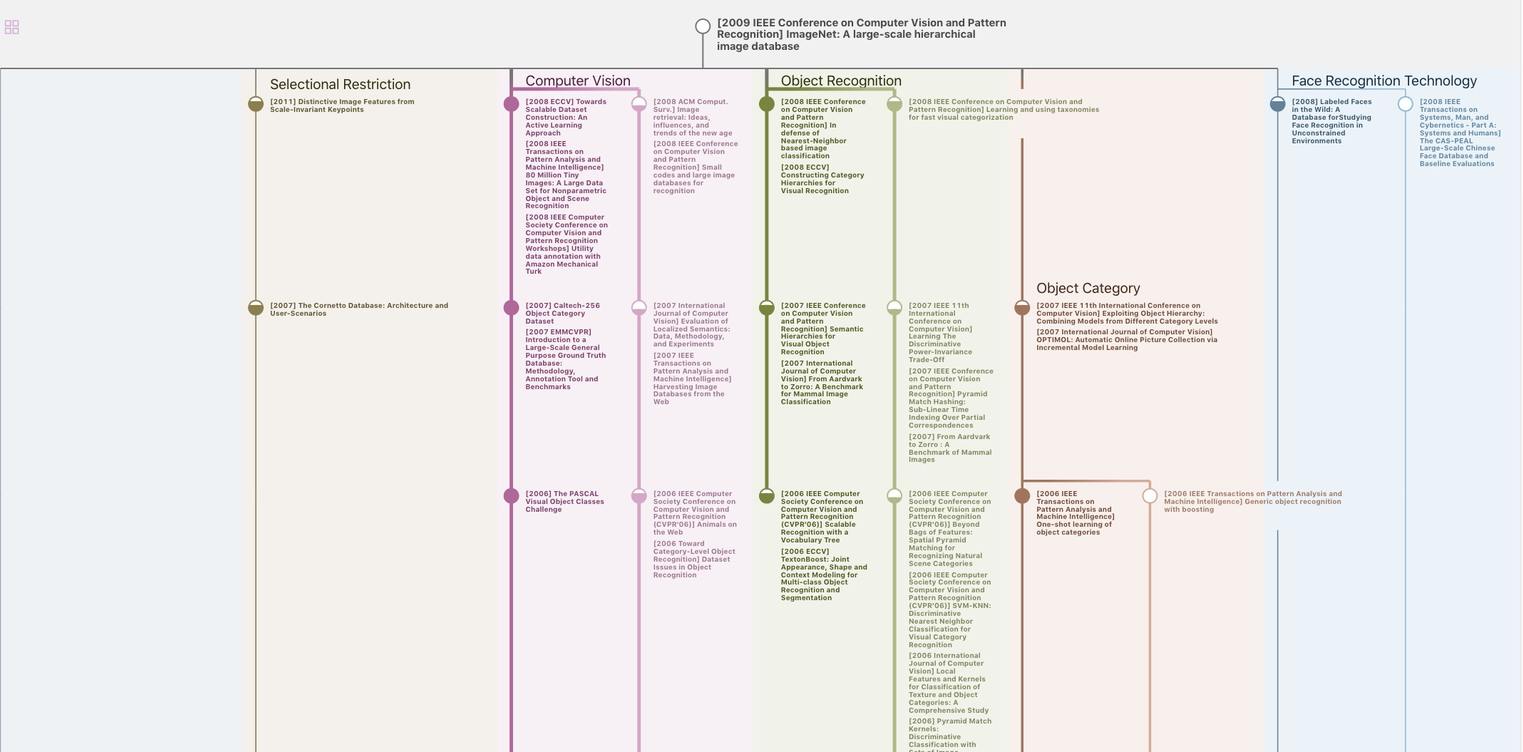
生成溯源树,研究论文发展脉络
Chat Paper
正在生成论文摘要