A hybrid Artificial Immune optimization for high-dimensional feature selection
Knowledge-Based Systems(2023)
Abstract
For high-dimensional data, the traditional feature selection method is slightly inadequate. At present, most of the existing hybrid search methods have problems of high computational cost and unsatisfactory feature reduction rate. In this paper, a hybrid feature selection method based on artificial immune algorithm optimization (HFSIA) is proposed to solve the feature reduction problem of high-dimensional data. This method combines the filter method with the metaheuristic-based search strategy more effectively. Inspired by biological research results, the method introduces a lethal mutation mechanism and a Cauchy mutation operator with adaptive adjustment factors to improve the search performance of the algorithm. In addition, this method introduces an adaptive adjustment factor in the population update stage to improve the problem of insufficient diversity of the original algorithm. The effective combination of these mechanisms enables the algorithm to obtain better search capability at a lower computational cost. Experimental comparisons with 23 state-of-the-art feature selection methods are conducted on 22 high-dimensional benchmark datasets. The results show that the computational cost of HFSIA is comparable to 5 classical feature selection methods known for their speed. Moreover, it achieves a higher average classification accuracy than 18 hybrid feature selection methods reported in the latest literature with the best feature reduction rate.
MoreTranslated text
Key words
Feature selection,Artificial immune algorithm,Hybrid search,High-dimensional data,Classification
AI Read Science
Must-Reading Tree
Example
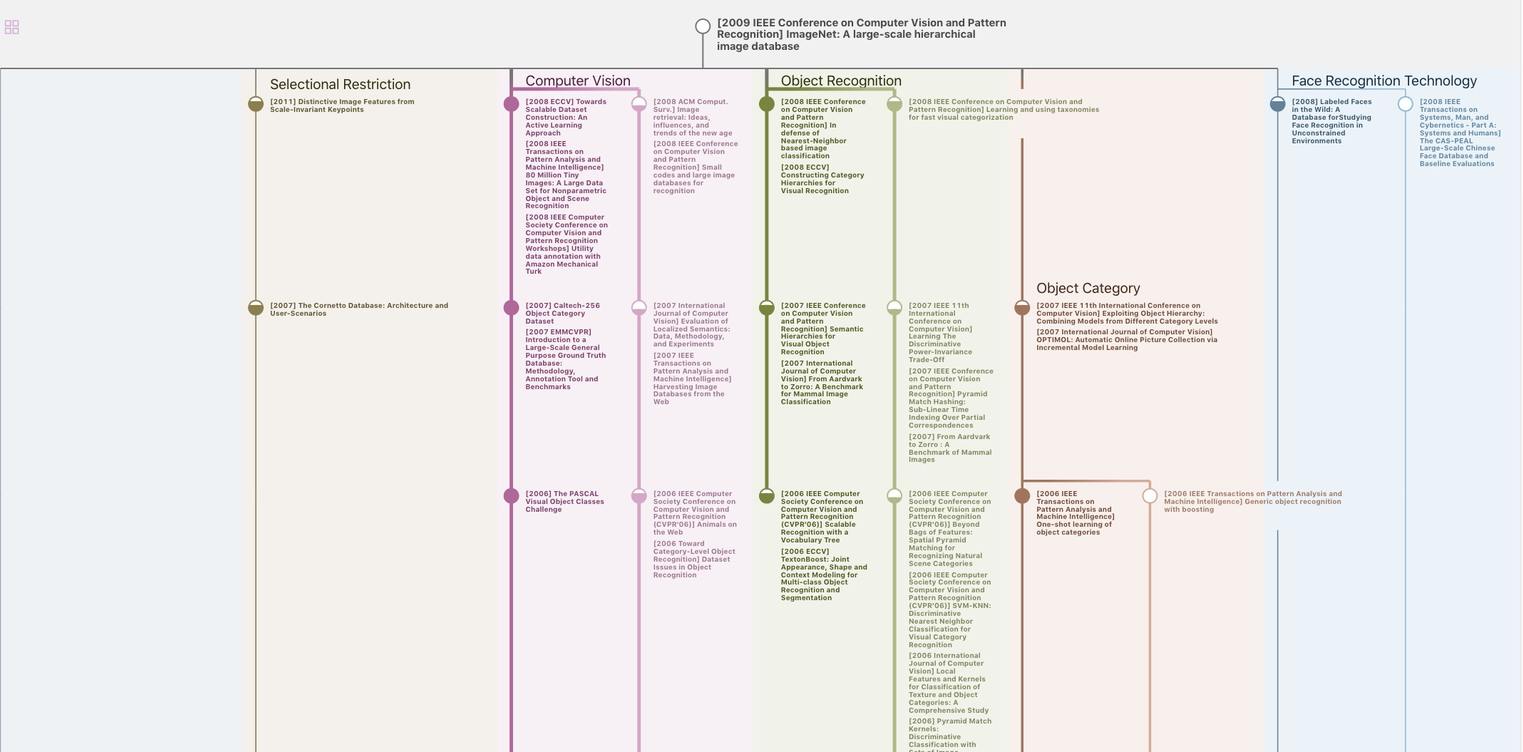
Generate MRT to find the research sequence of this paper
Chat Paper
Summary is being generated by the instructions you defined