A novel incremental attribute reduction by using quantitative dominance-based neighborhood self-information
Knowledge-Based Systems(2023)
摘要
Incremental attribute reduction aims to improve the efficiency of obtaining reduct from the dynamic data, which has attracted more and more attention. Nevertheless, the existing incremental attribute reduction methods for the dynamic ordered data with time-evolving objects ignore the updating strategies caused by selecting necessary (or removing redundant) attributes. To tackle this issue, we investigate a novel incremental attribute reduction based on quantitative dominance-based neighborhood self-information (QD-NSI) for dynamic hybrid ordered decision system. First, the measure QD-NSI is proposed by combining the quantitative dominance-based neighborhood rough set and the self-information. Different from the dependency commonly used in attribute reduction, the QD-NSI considers both deterministic and possible classification information. Based on the proposed measure, the corresponding matrix calculation method and heuristic attribute reduction algorithm are designed. Subsequently, the incremental attribute reduction mechanisms and algorithms for dynamic hybrid ordered decision system with time-evolving objects are explored. It is worth mentioning that the proposed incremental algorithms consider the update strategies caused by selecting necessary (or removing redundant) attributes. Finally, comparison experiments are performed on nine public datasets to illustrate the performance of the proposed incremental algorithms. Experimental results show that the QD-NSI based attribute reduction algorithm can effectively delete irrelevant or redundant attributes and the efficiency of the proposed incremental algorithms is better than that of the existing related incremental algorithms.
更多查看译文
关键词
Incremental attribute reduction,Quantitative dominance-based neighborhood rough set,Self-information,Hybrid ordered decision system
AI 理解论文
溯源树
样例
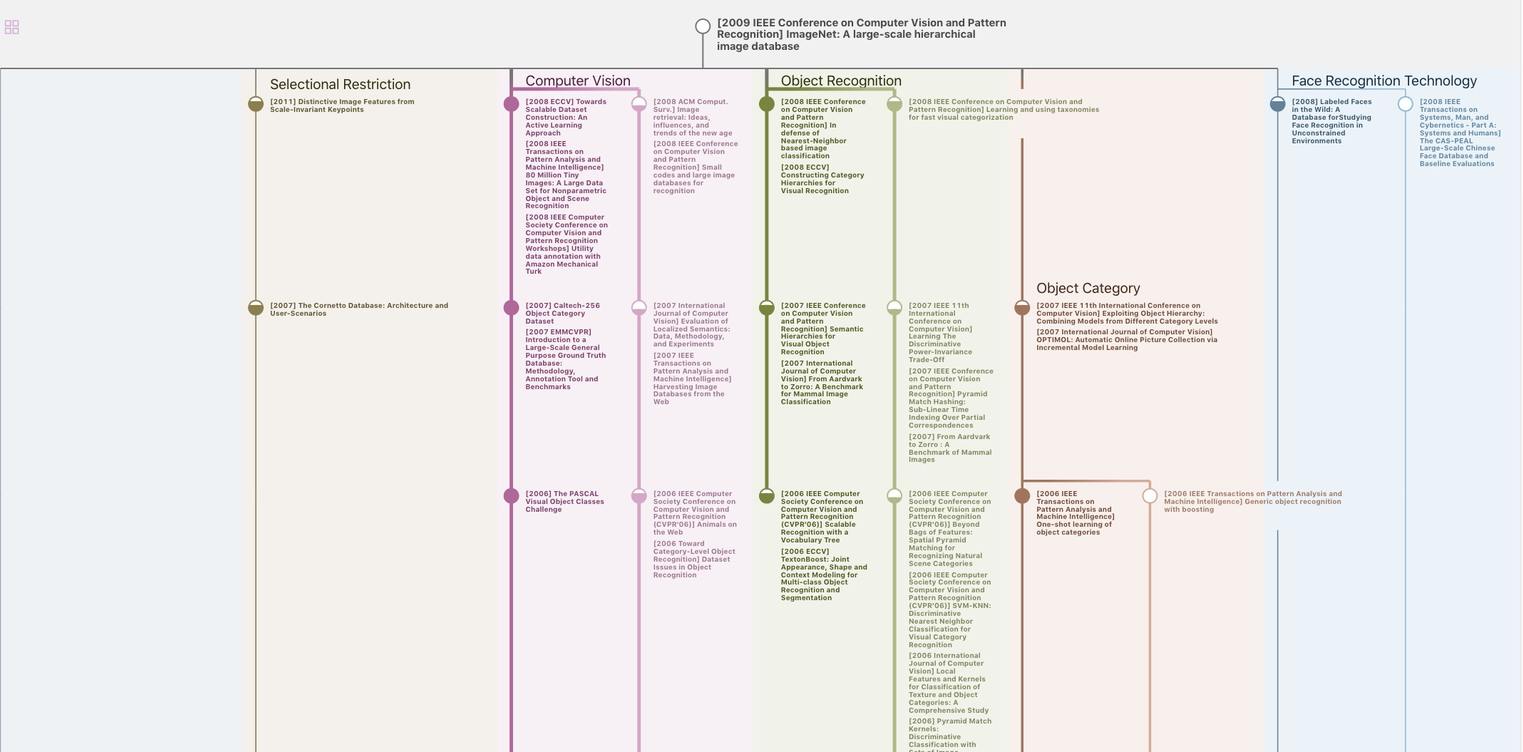
生成溯源树,研究论文发展脉络
Chat Paper
正在生成论文摘要