Discriminator feature-based progressive GAN inversion
Knowledge-Based Systems(2023)
摘要
Generative Adversarial Networks (GANs) receive extensive attention due to its capability of high-fidelity image synthesis. The synthesized content can be edited by imposing transformations on the latent codes. To edit real images, the GAN inversion methods mainly focus on learning an inverse mapping from the data space to the latent space of a well-trained GAN through a separate encoder. In this paper, we propose a Discriminator Feature-based Progressive Inversion (DFPI) model for GAN-based image reconstruction and enhancement. We find that the expressiveness of the generator feature is richer than that of the latent code for a given input image. To achieve high-quality reconstruction, we estimate the generator features in a light-weight optimization process, conditioned on off-the-shelf discriminator features. Specifically, a recomposition matrix is learnt to map high-level discriminator features to the high-level generator features due to their reverse structure. To progressively improve the reconstruction quality, we adopt a convolutional correction module to alleviate the estimation error at a lower generator layer, conditioned on the features of a lower discriminator layer. By performing the progressive correction process, the input images can be accurately reconstructed. Note that the resulting recomposition matrix and correction module can be generalized to unseen images. The experiments demonstrate the superior performance of DFPI over competing methods on GAN inversion as well as a variety of image enhancement tasks.
更多查看译文
关键词
Generative adversarial networks,GAN inversion,Feature recomposition,Image enhancement
AI 理解论文
溯源树
样例
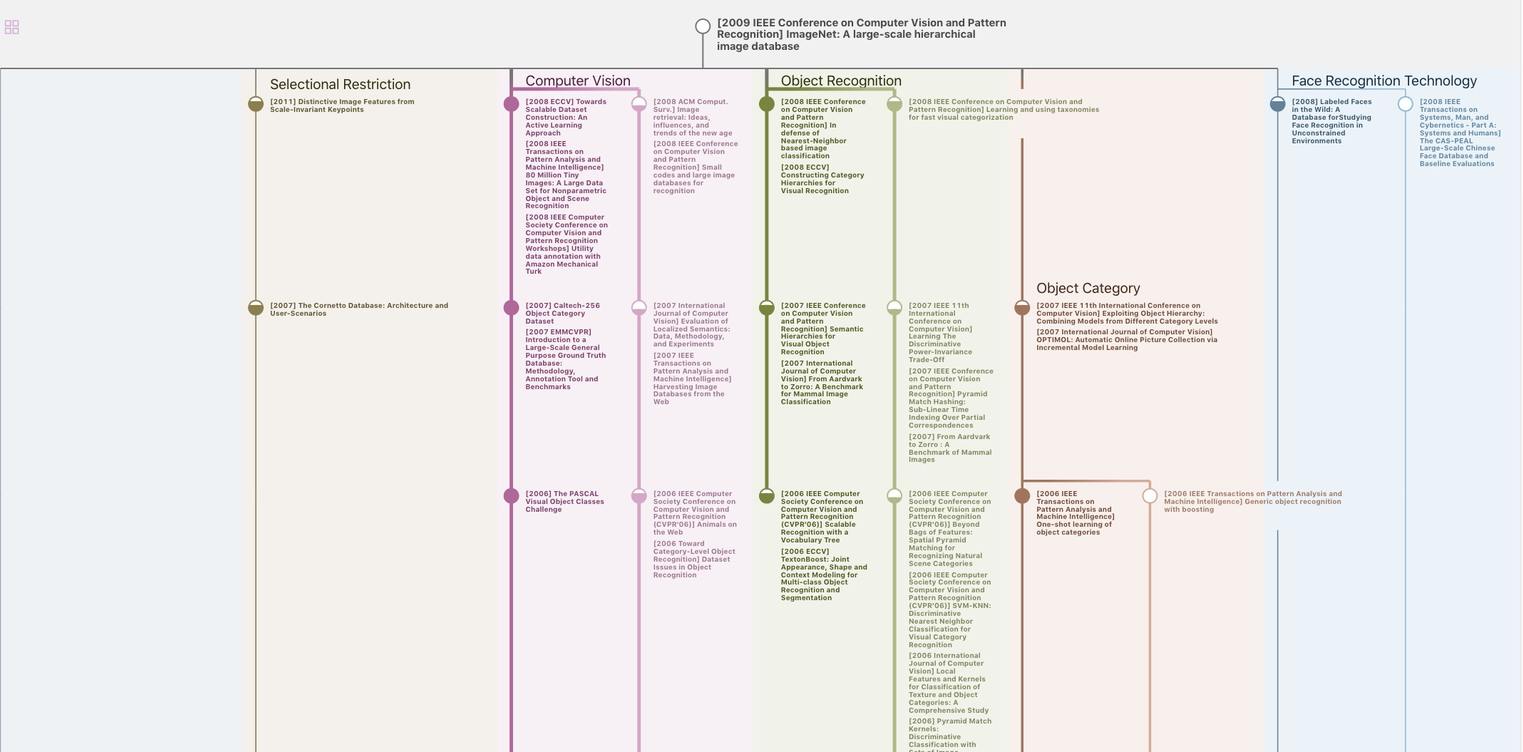
生成溯源树,研究论文发展脉络
Chat Paper
正在生成论文摘要