Adapting the Hypersphere Loss Function from Anomaly Detection to Anomaly Segmentation
CoRR(2023)
Abstract
We propose an incremental improvement to Fully Convolutional Data Description (FCDD), an adaptation of the one-class classification approach from anomaly detection to image anomaly segmentation (a.k.a. anomaly localization). We analyze its original loss function and propose a substitute that better resembles its predecessor, the Hypersphere Classifier (HSC). Both are compared on the MVTec Anomaly Detection Dataset (MVTec-AD) -- training images are flawless objects/textures and the goal is to segment unseen defects -- showing that consistent improvement is achieved by better designing the pixel-wise supervision.
MoreTranslated text
Key words
hypersphere loss function,anomaly detection,loss function
AI Read Science
Must-Reading Tree
Example
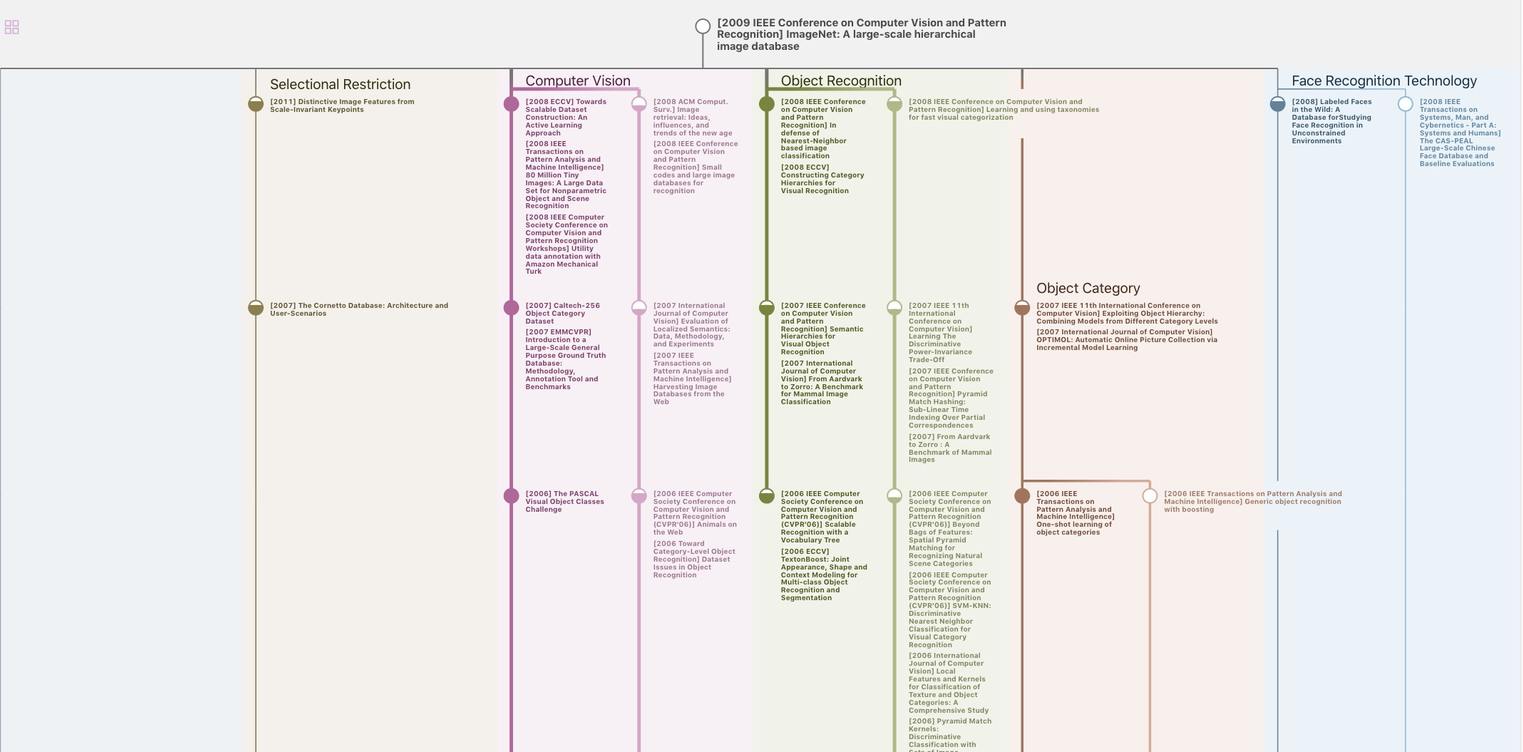
Generate MRT to find the research sequence of this paper
Chat Paper
Summary is being generated by the instructions you defined