Feature construction using explanations of individual predictions
CoRR(2023)
摘要
Feature construction can contribute to comprehensibility and performance of machine learning models. Unfor-tunately, it usually requires exhaustive search in the attribute space or time-consuming human involvement to generate meaningful features. We propose a novel heuristic approach for reducing the search space based on aggregation of instance-based explanations of predictive models. The proposed Explainable Feature Construction (EFC) methodology identifies groups of co-occurring attributes exposed by popular explanation methods, such as IME and SHAP. We empirically show that reducing the search to these groups significantly reduces the time of feature construction using logical, relational, Cartesian, numerical, and threshold (num-of-N and X-of-N) constructive operators. An analysis on 10 transparent synthetic datasets shows that EFC effectively identifies informative groups of attributes and constructs relevant features. Using 30 real-world classification datasets, we show significant improvements in classification accuracy for several classifiers and demonstrate the feasibility of the proposed feature construction even for large datasets. Finally, EFC generated interpretable features on a real-world problem from the financial industry, which were confirmed by a domain expert.
更多查看译文
关键词
Explainable artificial intelligence,Prediction explanation,Feature construction
AI 理解论文
溯源树
样例
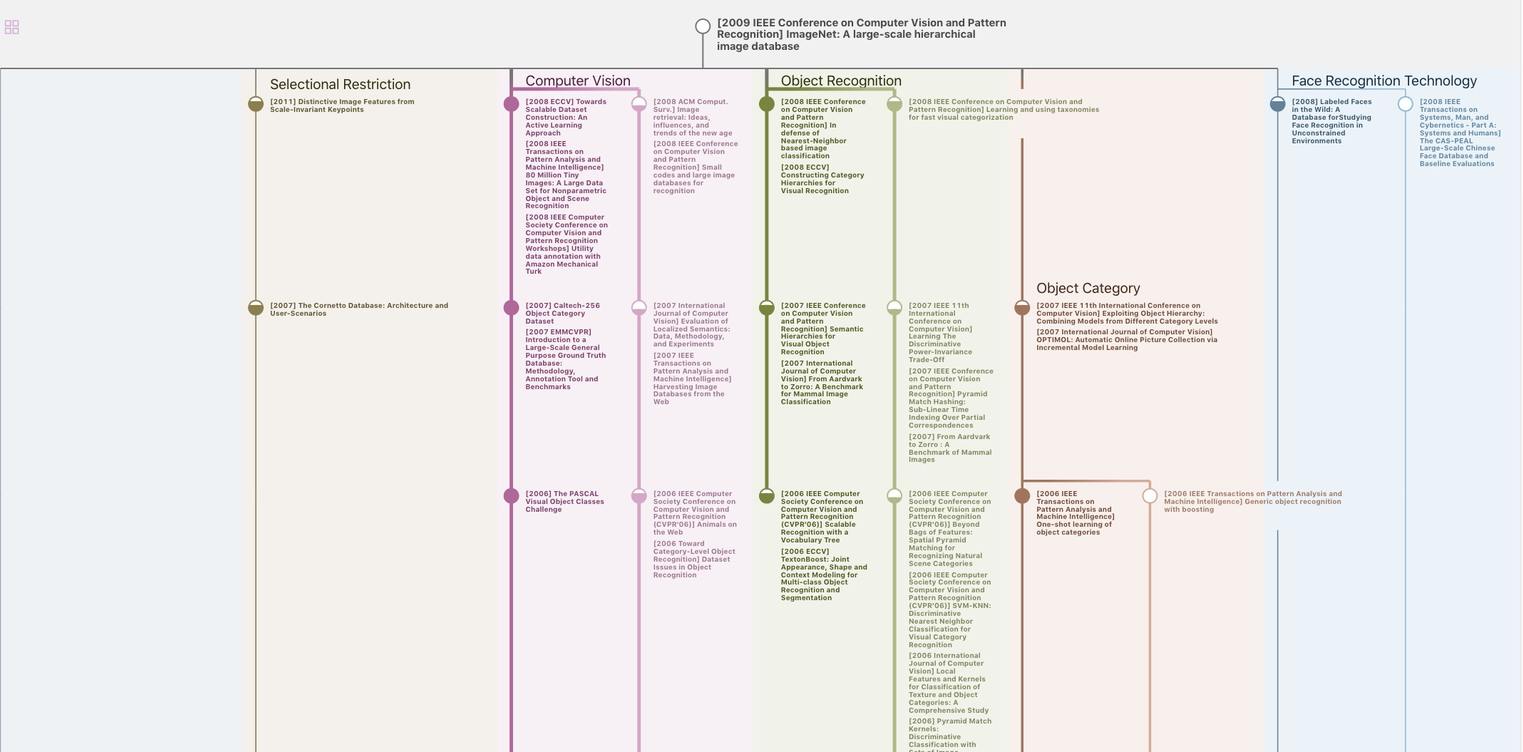
生成溯源树,研究论文发展脉络
Chat Paper
正在生成论文摘要