Slice Transformer and Self-supervised Learning for 6DoF Localization in 3D Point Cloud Maps
CoRR(2023)
摘要
Precise localization is critical for autonomous vehicles. We present a self-supervised learning method that employs Transformers for the first time for the task of outdoor localization using LiDAR data. We propose a pre-text task that reorganizes the slices of a $360^\circ$ LiDAR scan to leverage its axial properties. Our model, called Slice Transformer, employs multi-head attention while systematically processing the slices. To the best of our knowledge, this is the first instance of leveraging multi-head attention for outdoor point clouds. We additionally introduce the Perth-WA dataset, which provides a large-scale LiDAR map of Perth city in Western Australia, covering $\sim$4km$^2$ area. Localization annotations are provided for Perth-WA. The proposed localization method is thoroughly evaluated on Perth-WA and Appollo-SouthBay datasets. We also establish the efficacy of our self-supervised learning approach for the common downstream task of object classification using ModelNet40 and ScanNN datasets. The code and Perth-WA data will be publicly released.
更多查看译文
关键词
3D point cloud maps,6DoF localization,Appollo-SouthBay datasets,autonomous vehicles,LiDAR data,LiDAR map,localization annotations,localization method,ModelNet40,multihead attention,object classification,outdoor localization,outdoor point clouds,Perth city,Perth-Wa dataset,pre-text task,ScanNN dataset,self-supervised learning method,slice transformer,Western Australia
AI 理解论文
溯源树
样例
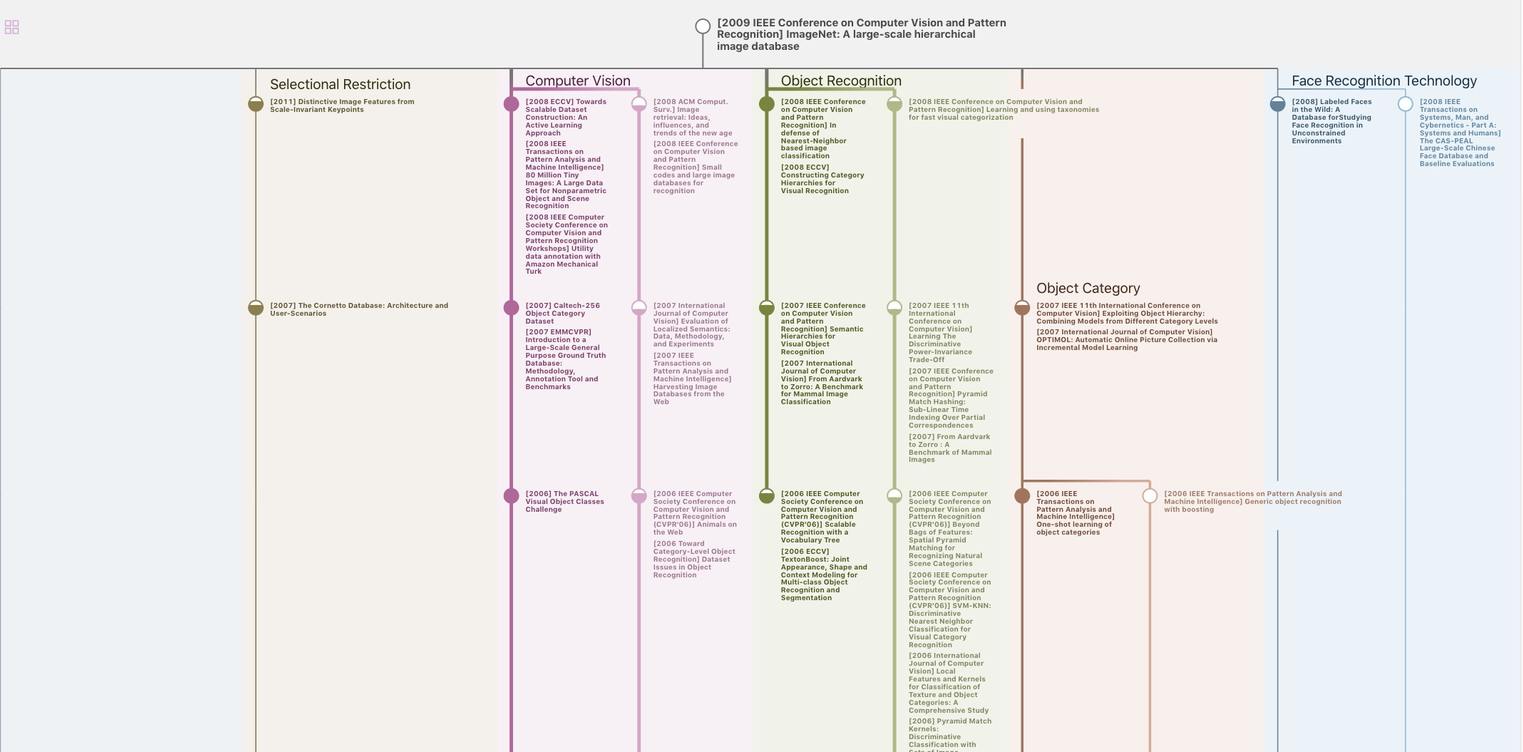
生成溯源树,研究论文发展脉络
Chat Paper
正在生成论文摘要