EREC: Enhanced Language Representations with Event Chains
INFORMATION(2022)
摘要
The natural language model BERT uses a large-scale unsupervised corpus to accumulate rich linguistic knowledge during its pretraining stage, and then, the information is fine-tuned for specific downstream tasks, which greatly improves the understanding capability of various natural language tasks. For some specific tasks, the capability of the model can be enhanced by introducing external knowledge. In fact, these methods, such as ERNIE, have been proposed for integrating knowledge graphs into BERT models, which significantly enhanced its capabilities in related tasks such as entity recognition. However, for two types of tasks, commonsense causal reasoning and predicting the ending of stories, few previous studies have combined model modification and process optimization to integrate external knowledge. Therefore, referring to ERNIE, in this paper, we propose enhanced language representation with event chains (EREC), which focuses on keywords in the text corpus and their implied relations. Event chains are integrated into EREC as external knowledge. Furthermore, various graph networks are used to generate embeddings and to associate keywords in the corpus. Finally, via multi-task training, external knowledge is integrated into the model generated in the pretraining stage so as to enhance the effect of the model in downstream tasks. The experimental process of the EREC model is carried out with a three-stage design, and the experimental results show that EREC has a deeper understanding of the causal relationship and event relationship contained in the text by integrating the event chains, and it achieved significant improvements on two specific tasks.
更多查看译文
关键词
external knowledge,event chains,commonsense causal reasoning,story ending prediction
AI 理解论文
溯源树
样例
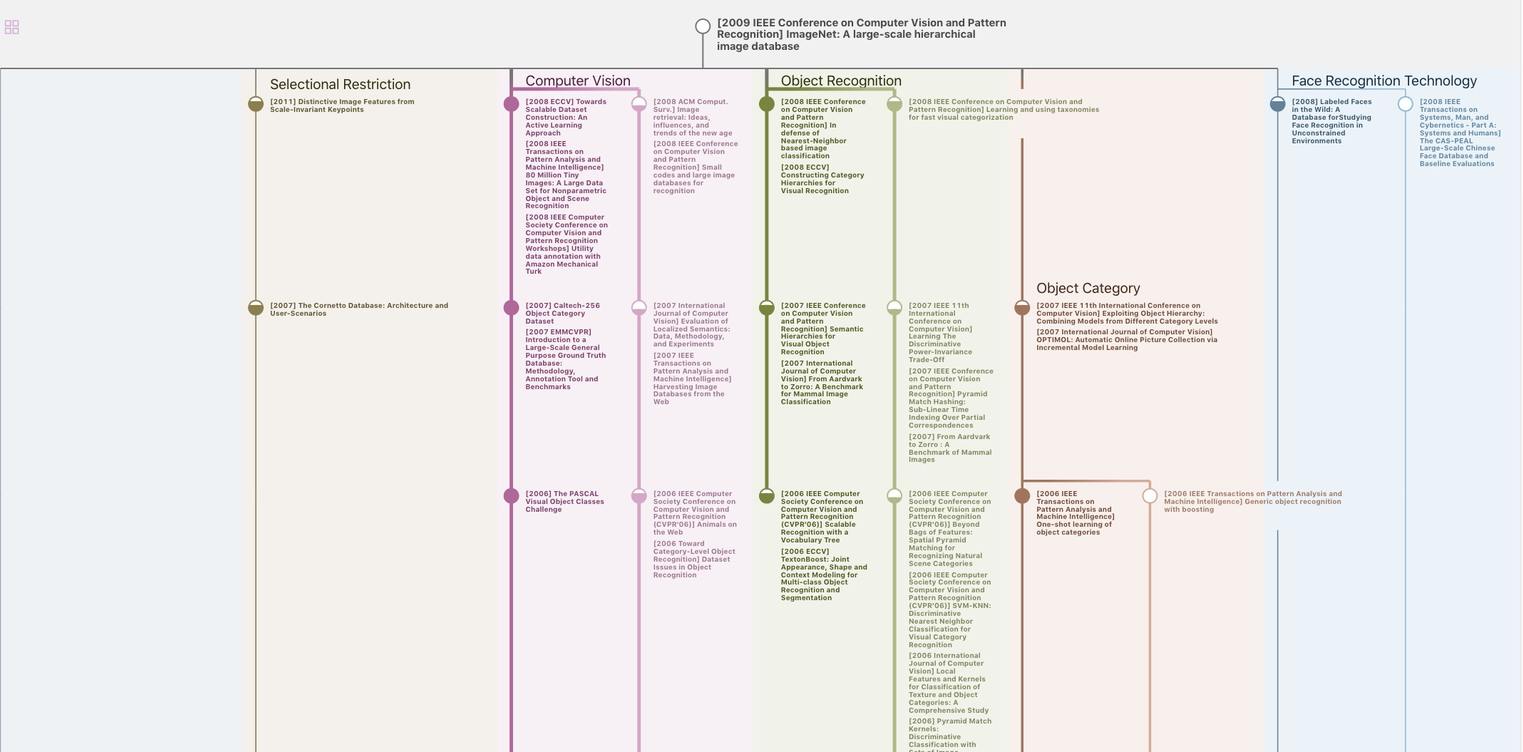
生成溯源树,研究论文发展脉络
Chat Paper
正在生成论文摘要