Social networks for enhanced player churn prediction in mobile free-to-play games
Applied Network Science(2022)
摘要
Social networks have been shown to enhance player experience in online games and to be important for the players, who often build complex communities. In online and mobile games, the behavior of players is bursty as they tend to play intensively at first for a short time and then quit playing altogether. Such players are known as churners. In the literature, several attempts have been made at predicting player churn in online and mobile games using behavioral features from the games’ player logs as input in supervised machine learning models. Previous research shows that information from social networks provides alternative and significant information when predicting churn, and yet the importance of networks has not been fully researched in mobile gaming. In this research, we study player churn in a mobile free-to-play game with one-versus-one matches. We build two types of networks based on how two players are matched. We train churn prediction models with features extracted from the networks to evaluate their predictive performance in terms of churn. Furthermore, we predict churn using the players’ behavioral features during their first day of game playing. According to our results, the network features greatly increase the predictive performance of the models, indicating that they carry alternative information about intention to churn. In addition, the first-day features are quite predictive, which means that first day activity is sufficient to predict churn of players quite accurately, validating the bursty behavior. Our research gives an indication of which aspects of game playing are associated with churn and allow us to study influence and social factors in mobile games.
更多查看译文
关键词
Mobile games,Churn prediction,Social networks,Implicit networks,Explicit networks,Burstiness
AI 理解论文
溯源树
样例
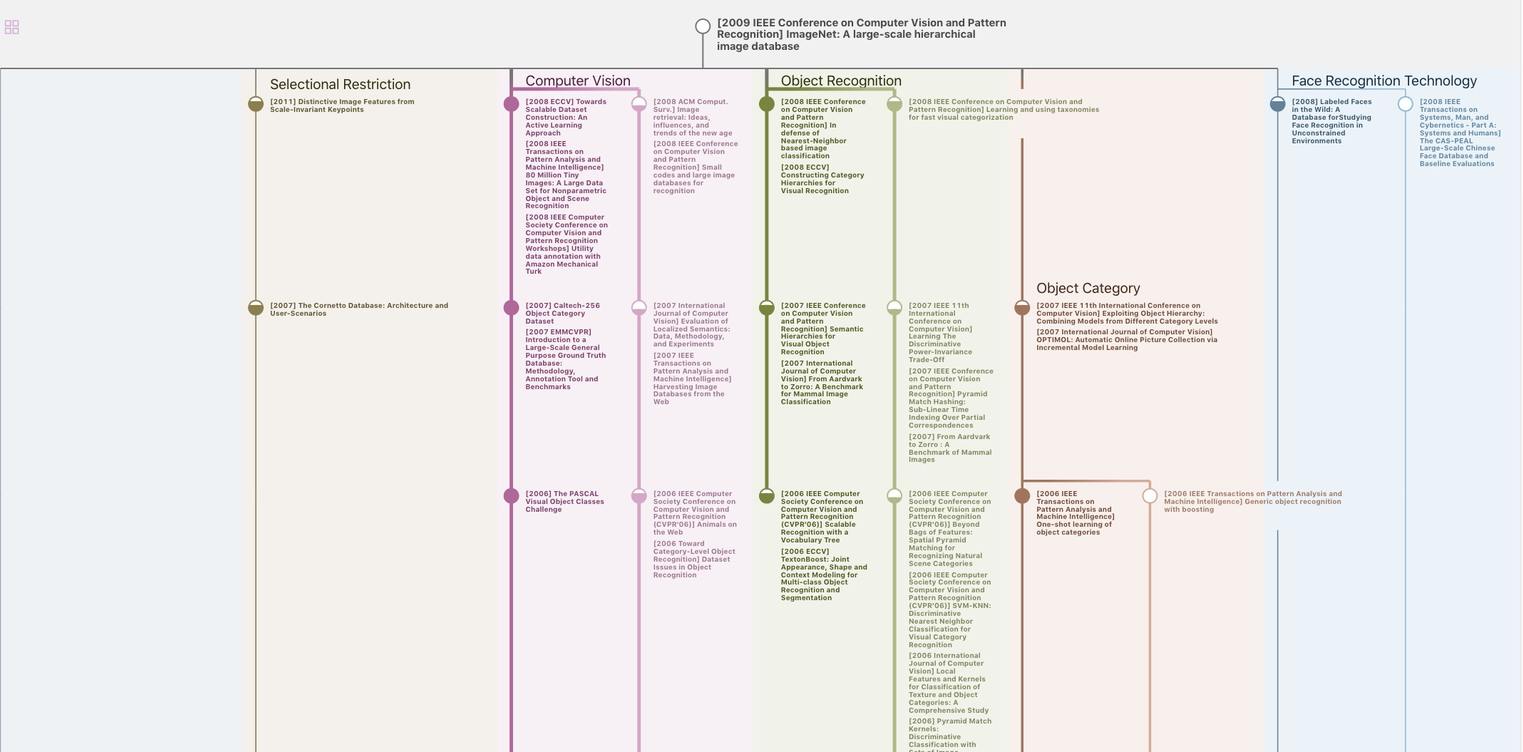
生成溯源树,研究论文发展脉络
Chat Paper
正在生成论文摘要