Binomial adversarial representation learning for machinery fault feature extraction and diagnosis.
Appl. Soft Comput.(2022)
摘要
The quality of feature extraction is a key factor in determining the performance of machinery fault diagnosis. The feature extraction of conventional deep learning-based methods has the disadvantages of uncontrollability and low quality. To overcome these disadvantages, we propose a binomial adversarial representation learning (BARL) method. Considering that the binomial distribution can make the representations have smaller intra-class distances (ICDs) and larger cross-class distances (CCDs), the adversarial learning mechanism and autoencoder are combined to learn representations obeying binomial distributions from raw monitoring signals to extract key features containing machine health information. Two case studies on rolling element bearings and gearboxes are carried out to validate the performance of the proposed method. The results show that the diagnosis accuracy of BARL outperformed comparison methods, especially under the condition of insufficient training data. According to quantitative and qualitative analysis, the representations learned by BARL were proven to have smaller ICDs and larger CCDs than the comparison methods, which illustrates the effectiveness of the proposed method.
更多查看译文
关键词
Representation learning,Binomial distribution,Fault feature extraction,Machinery fault diagnosis
AI 理解论文
溯源树
样例
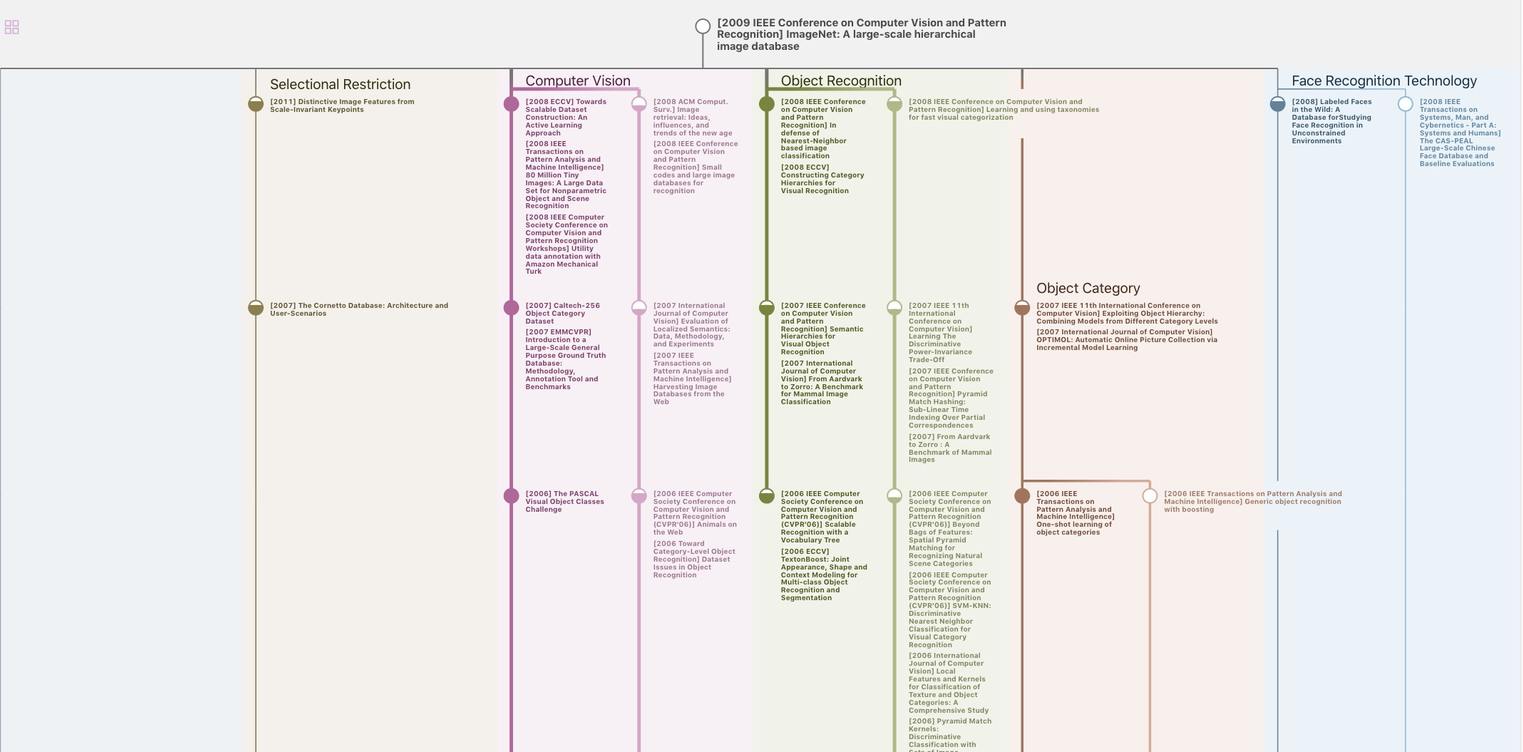
生成溯源树,研究论文发展脉络
Chat Paper
正在生成论文摘要