Anisotropic Weighted Total Variation Feature Fusion Network for Remote Sensing Image Denoising.
Remote. Sens.(2022)
摘要
Remote sensing images are widely applied in instance segmentation and objetive recognition; however, they often suffer from noise, influencing the performance of subsequent applications. Previous image denoising works have only obtained restored images without preserving detailed texture. To address this issue, we proposed a novel model for remote sensing image denoising, called the anisotropic weighted total variation feature fusion network (AWTVF(2)Net), consisting of four novel modules (WTV-Net, SOSB, AuEncoder, and FB). AWTVF(2)Net combines traditional total variation with a deep neural network, improving the denoising ability of the proposed approach. Our proposed method is evaluated by PSNR and SSIM metrics on three benchmark datasets (NWPU, PatternNet, UCL), and the experimental results show that AWTVF(2)Net can obtain 0.12 similar to 19.39 dB/0.0237 similar to 0.5362 higher on PSNR/SSIM values in the Gaussian noise removal and mixed noise removal tasks than State-of-The-Art (SoTA) algorithms. Meanwhile, our model can preserve more detailed texture features. The SSEQ, BLIINDS-II, and BRISQUE values of AWTVF(2)Net on the three real-world datasets (AVRIS Indian Pines, ROSIS University of Pavia, HYDICE Urban) are 3.94 similar to 12.92 higher, 8.33 similar to 27.5 higher, and 2.2 similar to 5.55 lower than those of the compared methods, respectively. The proposed framework can guide subsequent remote sensing image applications, regarding the pre-processing of input images.
更多查看译文
关键词
remote sensing image,noise removal,weighted total variation,feature fusion,convolutional neural network
AI 理解论文
溯源树
样例
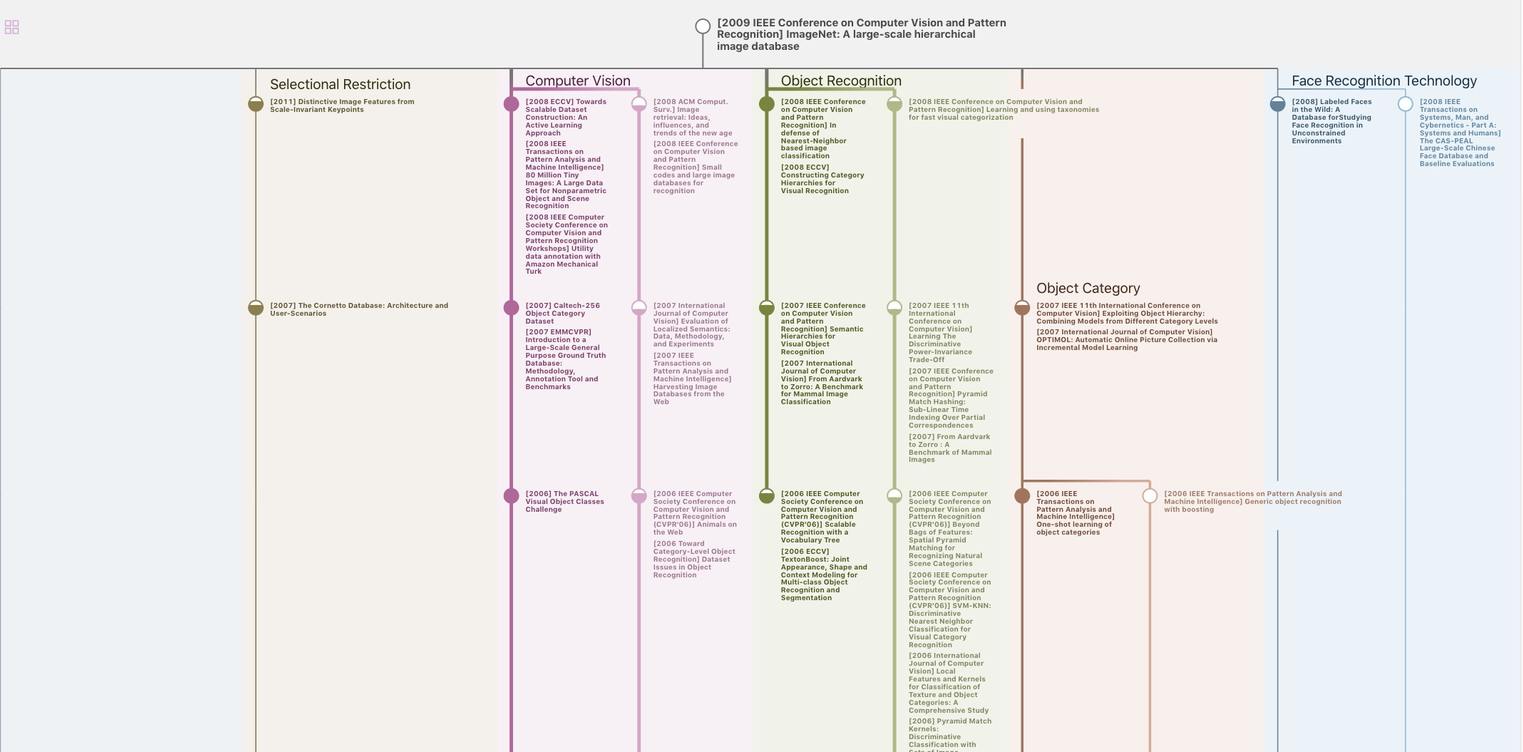
生成溯源树,研究论文发展脉络
Chat Paper
正在生成论文摘要